The convergence of GPUs, AI, and Quantum Computing marks a significant shift in technological advancements. These technologies, each powerful in their own right, are coming together to create solutions that were previously unimaginable. From enhancing data analysis in finance to revolutionizing healthcare diagnostics, the integration of these systems is setting the stage for a new era of computational efficiency and innovation.
The Convergence of GPUs, AI, and Quantum Computing
GPUs, originally designed for complex graphics and video processing, now play a central role in AI applications due to their parallel processing capabilities. These features accelerate machine learning computations, benefiting industries like healthcare, finance, and manufacturing. AI systems can swiftly analyze medical images or predict stock market trends, offering vast potential for transformation.
Quantum Computing, with its qubits capable of existing in multiple states simultaneously, promises even greater advancements. Unlike traditional binary bits, quantum computers can handle vast datasets and perform complex calculations at unprecedented speeds, unlocking new possibilities across various sectors.
Quantum AI combines these advancements, meeting the demands for faster data analysis and pattern recognition. In finance, this means more accurate predictions and optimized trading strategies. Healthcare benefits from rapid genomic analysis, streamlining drug discovery and personalized treatments.
Cybersecurity is significantly enhanced by this synergy. Quantum AI can quickly analyze large datasets, identifying and neutralizing threats in real-time. Quantum-resistant cryptography fortifies data security, adapting to quantum-level decryption attempts. eDiscovery becomes quicker and more precise, saving time and resources while ensuring data integrity in legal proceedings.
However, integrating these technologies poses challenges. Compatibility between GPUs and quantum computers requires ongoing research due to their different operational mechanisms. Scaling quantum systems to practical levels involves complex error correction techniques to maintain qubit reliability.
Organizations like IBM, Google, and Microsoft are heavily investing in overcoming these challenges. IBM's Heron, with hundreds or thousands of qubits, represents a significant step forward. Fujitsu's 64-qubit architecture and 40-qubit quantum simulator highlight rapid advancements in the field. Progress is being made towards achieving fault-tolerant quantum computing, a milestone that could revolutionize computational efficiency and security.
NVIDIA's Blackwell platform introduces groundbreaking advancements in GPU architecture. Its six transformative technologies, including the world's most powerful chip and second-generation transformer engine, promise substantial improvements in AI applications, data processing, and quantum computing. With enhanced reliability, security, and data analysis capabilities, Blackwell is set to become a critical component in AI-driven industries.
Public and private investments in quantum technologies are escalating. IDC forecasts that by 2027, investments in quantum computing will reach $16.4 billion.1 Companies like Fujitsu and NVIDIA are pushing boundaries, developing new architectures and platforms that harness the combined power of GPUs, AI, and quantum computing.
Hybrid quantum-classical algorithms integrate the strengths of both classical and quantum computing, solving complex problems previously deemed intractable. This approach unlocks potential across various fields, enhancing computational efficiency and paving the way for groundbreaking advancements.
The collaboration across industries and the open-source development of AI frameworks contribute to the democratization of these technologies. Platforms like GitHub foster a collaborative environment, accelerating innovation and making these advanced technologies accessible to a broader audience.
Advancements in Quantum Computing
IBM's Heron and Google's Sycamore showcase the incredible potential of qubits and quantum mechanics to transform computation. Quantum computers illustrate a significant departure from traditional binary systems. Qubits possess the ability to exist in multiple states at once, enabling computations at speeds unimaginable with classical computers.
IBM's Heron, featuring hundreds or thousands of qubits, embodies the rapid progress in this domain. It demonstrates the feasibility of large-scale quantum systems and highlights the practical applicability of quantum algorithms. Google's Sycamore epitomizes the concept of quantum supremacy, solving a problem in 200 seconds that would take a classical supercomputer 10,000 years.2 These milestones signify that quantum computing is becoming a tangible technology capable of tackling complex problems across various industries.
In finance, quantum computing can vastly improve market predictions and risk assessment models. Quantum algorithms process and analyze vast datasets more efficiently, providing optimized trading strategies. The healthcare sector stands to gain immensely as well. The speed and precision of quantum computers in analyzing genomic data may revolutionize drug discovery and personalized medicine, leading to faster and more effective treatment options.
Material science is also poised for transformation. Simulating molecular structures using quantum computers can lead to the discovery of new materials with unique properties, opening possibilities in energy storage and semiconductor design. Similarly, quantum computing holds the potential to revolutionize climate modeling, enabling accurate simulation of climate patterns and effective strategies to combat climate change.
However, the journey towards fully operational and practical quantum computers is fraught with challenges. Ensuring qubit stability and managing quantum errors are significant hurdles. Quantum error correction techniques are critical to scaling quantum systems, aiming to mitigate the sensitivity of qubits to external disturbances. Researchers are continually working on these techniques to build more robust and fault-tolerant quantum computers.
Hybrid quantum-classical algorithms present a promising avenue, leveraging the strengths of both paradigms to solve problems previously deemed unsolvable. These algorithms harness the processing power of classical systems while utilizing quantum capacities for specific tasks, providing a balanced and efficient computing model.
On the horizon, more powerful quantum computers are anticipated, driven by substantial investments from both public and private sectors. IDC's projection of $16.4 billion in quantum computing investments by 2027 underscores the growing recognition of quantum potential.1 Companies like IBM, Google, Microsoft, and Fujitsu are at the forefront, developing quantum architectures and platforms that promise to redefine computational boundaries.
As these technological advancements continue to unfold, the implications for industries are profound. Quantum computing, once a concept relegated to theoretical physics, is steadily moving towards practical, real-world applications.
Quantum AI Applications
Quantum AI, a fusion of Quantum Computing and Artificial Intelligence, emerges as a groundbreaking technology revolutionizing various industries, notably financial markets, healthcare, cybersecurity, and eDiscovery. This convergence leverages the immense power of quantum mechanics to bolster AI's capabilities, enabling tasks that were previously infeasible with classical systems.
In the financial sector, Quantum AI transforms predictive analysis by handling massive datasets and performing intricate calculations at unprecedented speeds. This advancement leads to more precise market forecasts and optimized trading strategies. Traders and analysts can leverage these insights to make informed decisions, mitigate risks, and capture emerging opportunities. Financial institutions benefit from enhanced portfolio management and automatic detection of fraudulent activities.
Healthcare stands to gain immensely from Quantum AI's capabilities. Quantum AI can analyze genomic data with remarkable speed, facilitating rapid identification of genetic markers and the development of personalized medical treatments. This capability accelerates drug discovery, reduces development costs, and opens doors to therapies that improve patient outcomes. Immediate analysis of complex medical data also enhances predictive diagnostics, potentially catching diseases at early and more treatable stages.
Cybersecurity is significantly bolstered by Quantum AI. The ability to process vast amounts of data in real-time enhances threat detection and mitigation. Quantum AI systems can identify anomalous patterns in network traffic and detect potential cyber threats far more swiftly than traditional systems. Additionally, quantum-resistant cryptography offers protection against advanced decryption techniques, ensuring that sensitive data remains secure.
In the legal domain, particularly in eDiscovery, Quantum AI proves invaluable. Quantum AI accelerates the process of managing and analyzing massive volumes of electronic data during legal proceedings. It rapidly sifts through documents, emails, and digital evidence to identify relevant information. This capability reduces the time and costs associated with legal discovery and improves the accuracy and thoroughness of the search. Moreover, Quantum AI can detect and highlight potential evidence tampering, preserving the integrity of the legal process.
The applications of Quantum AI reflect its transformative potential across diverse sectors, driving innovations that enhance efficiency, accuracy, and security. As these technologies continue to evolve, we can anticipate more profound impacts, fostering advancements that address some of the most complex challenges we face today.
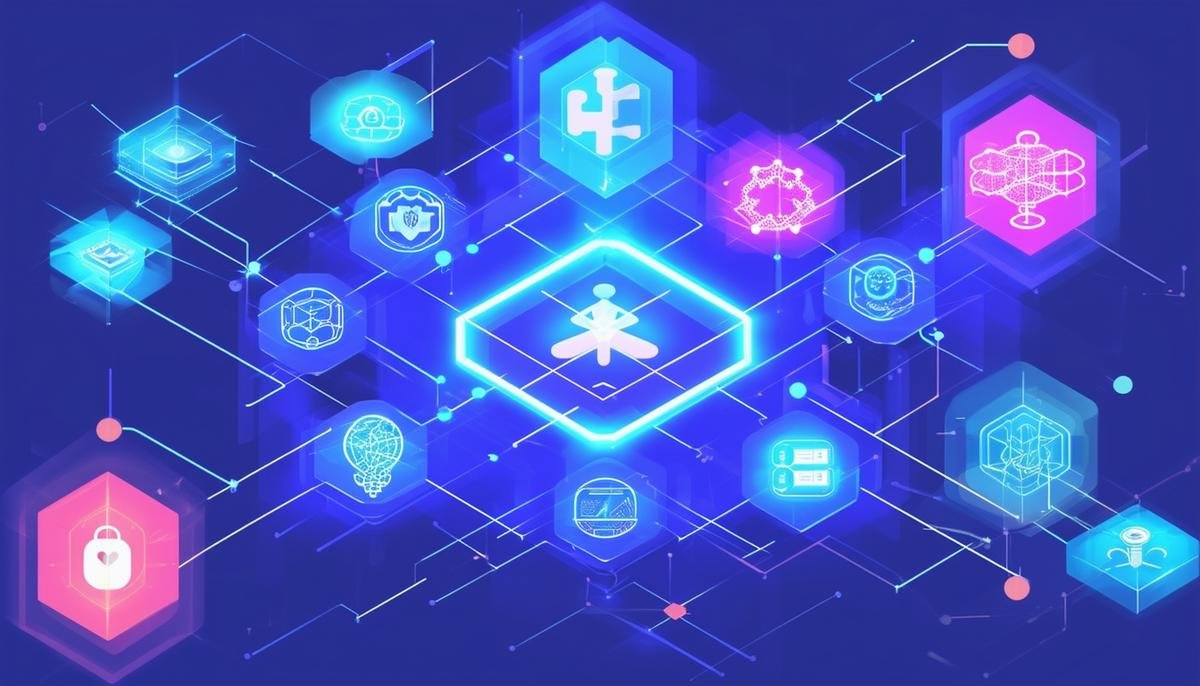
Challenges and Solutions in Quantum Computing
The integration of quantum computing with GPUs and AI presents several challenges that researchers and industry leaders are actively working to overcome. One of the primary obstacles is the compatibility between these fundamentally different types of computing technologies. While GPUs excel at parallel processing and handling large datasets, quantum computers leverage qubits and quantum mechanics principles, which require unique operating conditions and are highly sensitive to external disturbances. Bridging this gap necessitates innovative solutions that can seamlessly combine classical and quantum computational capabilities.
Scaling quantum computing is a significant challenge. Quantum systems must manage qubit errors and maintain stability, which is no small feat given the fragile nature of qubits. Quantum error correction techniques are critical in addressing this issue. These techniques involve intricate processes designed to detect and correct errors without disturbing the quantum state. For example, the use of redundant qubits to encode data helps in identifying and rectifying errors that occur due to decoherence or operational faults. As these techniques advance, they are instrumental in pushing the boundaries of quantum computing towards more practical and reliable applications.
Hybrid quantum-classical algorithms represent a promising solution to compatibility and scalability challenges. These algorithms leverage the strengths of both classical and quantum computing, allocating tasks based on the best-suited technology for the problem at hand. This hybrid approach ensures optimal use of available resources, enhancing overall computational performance and making quantum computing more accessible and functional.
Further advancements in quantum computing infrastructure are crucial. IBM's recent developments, such as System Two, exemplify steps towards modular quantum systems that can connect multiple quantum processors.3 This modularity is key to scaling quantum systems, enabling them to handle larger, more complex problems. Similarly, advancements by companies like Fujitsu and the introduction of the world's fastest 40-qubit quantum simulator demonstrate significant progress in making quantum computing more practical.
Software plays a pivotal role in bridging the gap between classical and quantum computing. Developers are creating innovative quantum algorithms and frameworks that facilitate the integration of quantum capabilities into existing classical computing environments. Platforms like Microsoft's Azure Quantum provide a comprehensive suite of tools and resources for developers, enabling them to experiment with and develop quantum applications. This collaborative approach accelerates the development and deployment of hybrid quantum-classical solutions.
Robust encryption and security measures are essential to protect the integrity and confidentiality of data processed by quantum systems. Quantum-resistant cryptography is being developed to withstand potential vulnerabilities introduced by quantum decryption capabilities.4 This ensures that as quantum computing becomes more prevalent, the security of sensitive information remains uncompromised.
While the integration of quantum computing with GPUs and AI poses significant challenges, ongoing research and development efforts are paving the way for practical solutions. Quantum error correction techniques and hybrid quantum-classical algorithms are at the forefront, enhancing the scalability and compatibility of quantum systems. As the technology continues to evolve, we can expect more seamless integration and groundbreaking advancements, driving forward the capabilities and applications of quantum computing in various industries.
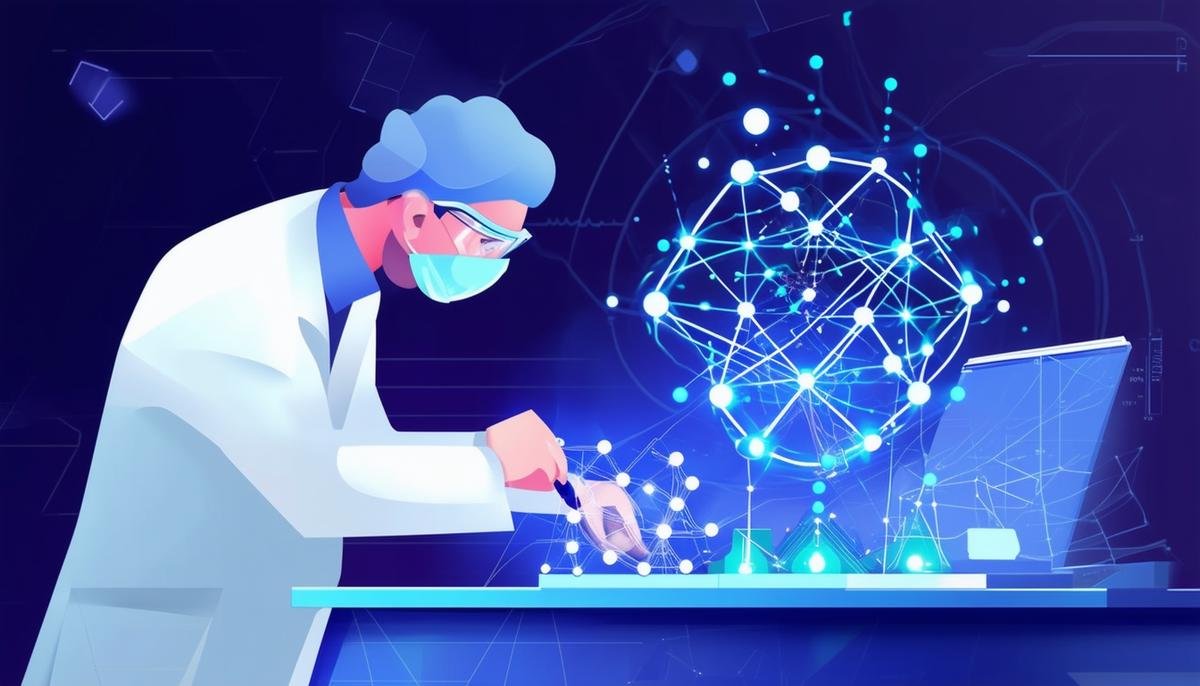
NVIDIA's Blackwell Platform
NVIDIA's Blackwell platform represents a significant leap in GPU technology, positioned to catalyze a new era of computing with its powerful capabilities and applications across various sectors. The platform incorporates six transformative technologies designed to maximize efficiency and performance in data processing, engineering simulation, electronic design automation, computer-aided drug design, and generative AI.
At the core of the Blackwell platform is the world's most powerful chip, which boasts 208 billion transistors. Manufactured using a custom-built 4NP TSMC process, the chip employs two-reticle limit GPU dies connected by a 10 TB/second chip-to-chip link, creating a single, unified GPU. This level of integration significantly boosts computational power and speeds, enabling real-time processing of immense data sets.
The second-generation transformer engine is another cornerstone of the Blackwell platform, doubling the compute and model sizes that can be handled due to its new micro-tensor scaling support and advanced dynamic range management algorithms. These enhancements, integrated into NVIDIA TensorRT-LLM and NeMo Megatron frameworks, facilitate more sophisticated AI inference, supporting 4-bit floating point operations. This capability is crucial for training and deploying trillion-parameter large language models (LLMs), which are essential in generative AI applications.
The platform's fifth-generation NVLink significantly accelerates performance for multitrillion-parameter and mixture-of-experts AI models. With a 1.8TB/s bidirectional throughput per GPU, NVLink ensures seamless, high-speed communication among up to 576 GPUs, making it ideal for the most complex LLMs. This capability is instrumental in training large models faster and more efficiently, directly impacting the development of advanced AI solutions.
A dedicated RAS (Reliability, Availability, Serviceability) engine in Blackwell-powered GPUs adds a new layer of robustness to the platform. Utilizing AI-based preventive maintenance, these GPUs can run diagnostics and forecast potential reliability issues. This improves system uptime and resiliency, ensuring that massive-scale AI deployments can operate continuously for extended periods.
The Blackwell platform's secure AI capabilities introduce advanced confidential computing features, crucial for data-sensitive industries like healthcare and financial services. These features protect AI models and customer data without compromising performance, supporting new native interface encryption protocols. This ensures that even as AI applications become more pervasive, data privacy and security standards can be maintained.
A dedicated decompression engine further supports the Blackwell platform, accelerating database queries and enhancing data analytics and data science performance. This engine enables organizations to handle large volumes of data more efficiently, unlocking faster insights and driving better business decisions.
The NVIDIA GB200 Grace Blackwell Superchip, a pivotal component of the platform, exemplifies the integration of the Blackwell GPUs with NVIDIA's Grace CPUs. This combination, connected via a 900GB/s ultra-low-power NVLink chip-to-chip interconnect, maximizes AI performance. The GB200 is part of the NVIDIA GB200 NVL72 system, which integrates 72 Blackwell GPUs and 36 Grace CPUs, delivering unprecedented performance for the most compute-intensive workloads. This system acts as a single GPU with 1.4 exaflops of AI performance and 30TB of fast memory, setting a new benchmark for processing power in AI applications.
The widespread adoption of Blackwell technology by industry leaders such as AWS, Google, Meta, Microsoft, and Tesla underscores its significance. These organizations leverage Blackwell's capabilities to enhance their AI and computing solutions, driving forward innovations in areas ranging from cloud computing to automotive technologies.
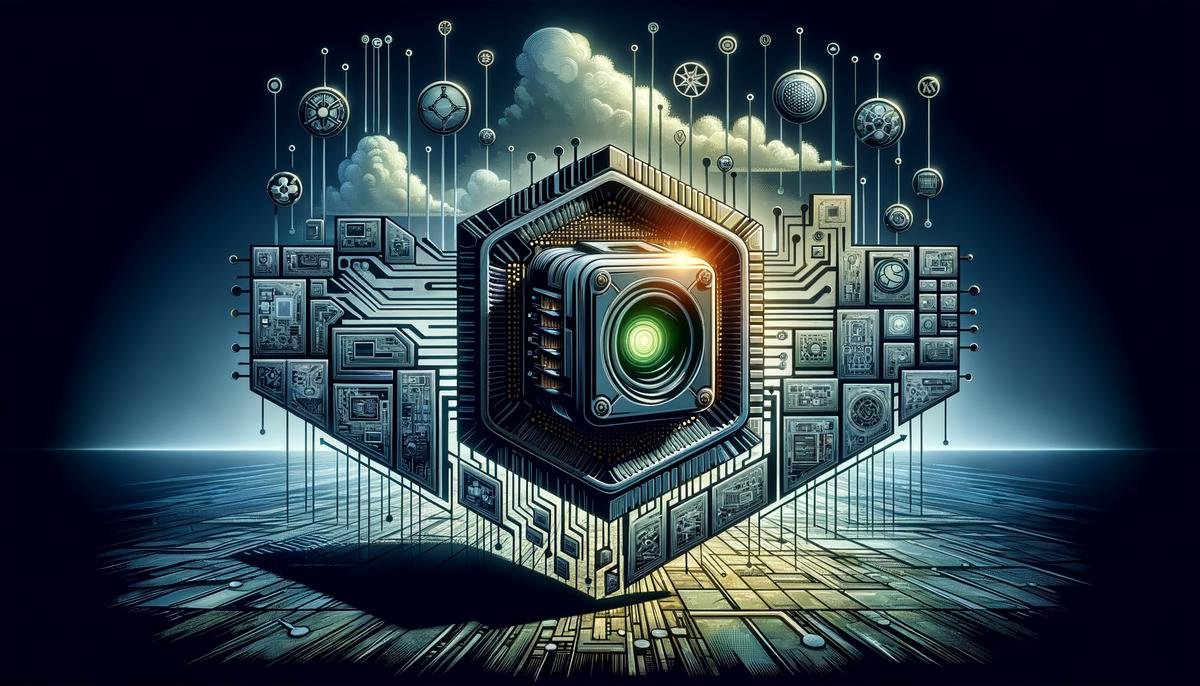