Smart Grids and AI Integration
In the world of energy management, artificial intelligence (AI) is a game-changer for smart grids. By analyzing massive data sets in real-time, these sophisticated AI systems enable utility providers to predict, adjust, and streamline energy distribution. This enhances grid stability and helps meet peak demand with precision.
AI integration into smart grids allows for real-time monitoring and response systems that can detect disruptions and adjust the flow of electricity accordingly. During unexpected high demand, AI can analyze incoming data and redistribute power to maintain grid balance, preventing potential blackouts and promoting reliable service delivery.
AI-driven predictive maintenance is becoming commonplace. Advanced algorithms can forecast potential breakdowns in the grid infrastructure before they occur, allowing for preemptive maintenance actions that save costs and extend the asset’s life cycle without disrupting the continuous supply of power. Companies like General Electric are already using AI to predict maintenance needs for wind turbines, reducing downtime and increasing energy output.
Through AI, smart grids utilize pattern recognition to improve operational efficiency. By examining past consumption trends and comparing them to real-time data, AI can accurately forecast energy needs. This significantly aids utility companies in planning their energy distribution, reducing wastage and optimizing power production.
AI also caters to customer-centric demands. Advanced algorithms play crucial roles during demand response events, where they manage the load by orchestrating how and when energy is consumed by each consumer. During peak hours, AI systems can temporarily reduce power consumption in non-essential functions, balancing the grid and avoiding overloads.
Companies like Schneider Electric employ intelligent grid systems that adjust energy distribution in response to changing consumption patterns introduced by renewable energy sources. Whether it’s a cloudy day impacting solar output or inconsistent wind speeds affecting turbines, AI in smart grids ensures a stable and continuous energy supply.
As smart grids evolve, they incorporate comprehensive conservation strategies by analyzing user consumption over time. AI provides personalized tips aimed at reducing excessive use, fostering an environment that encourages energy-efficient practices across communities.
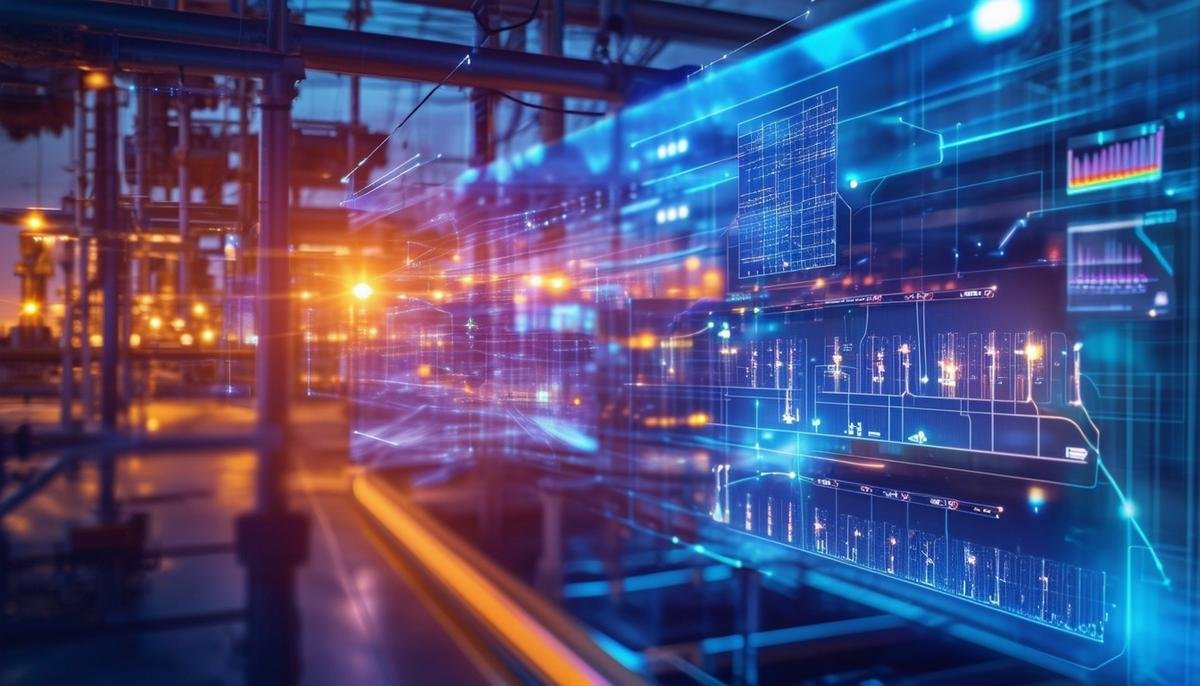
Impact of AI on Energy Consumption
Predictive analytics paired with AI in smart grids significantly contributes to energy waste reduction. Through the synthesis of historical consumption data and real-time operational metrics, AI anticipates system needs. This anticipation is rooted in predictive capabilities that preempt energy overuse, promoting a conservation-oriented energy ecosystem.
Companies harnessing smart metering technology empowered by AI use vast amounts of data to understand and predict consumer behavior patterns. Utilities equipped with smart meters, which transmit detailed consumption data in real-time, allow AI systems to analyze and adjust the energy flow to optimize consumption metrics. This operational foresight facilitates smoother utility operation and a leaner energy footprint. Pacific Gas and Electric Company have deployed smart meters across residential and commercial spaces, enhancing their ability to manage energy resources more efficiently, reducing unnecessary energy expenditure, and bolstering sustainability efforts.1
AI systems can integrate weather predictions to control HVAC operations across massive corporate buildings or adjust street lighting based on traffic data. Such modifications orchestrated by AI lead to considerable energy savings and operational cost reductions.
Southern California Edison utilizes predictive analytics to gauge immediate demands and long-term trends in energy usage, enabling more informed, proactive decisions on energy distribution and load balancing.2 Their efforts highlight the dual advantage of AI in lean management and predictive fault detection—themes central to modern energy strategies focusing on sustainability and resiliency.
AI transforms energy management from traditionally reactive systems into dynamic, proactive grid ecosystems that ensure energy efficiency and enhanced supply quality. As technology evolves, these systems refine their predictive capabilities, ensuring compliance with both consumer demand and environmental sustainability.
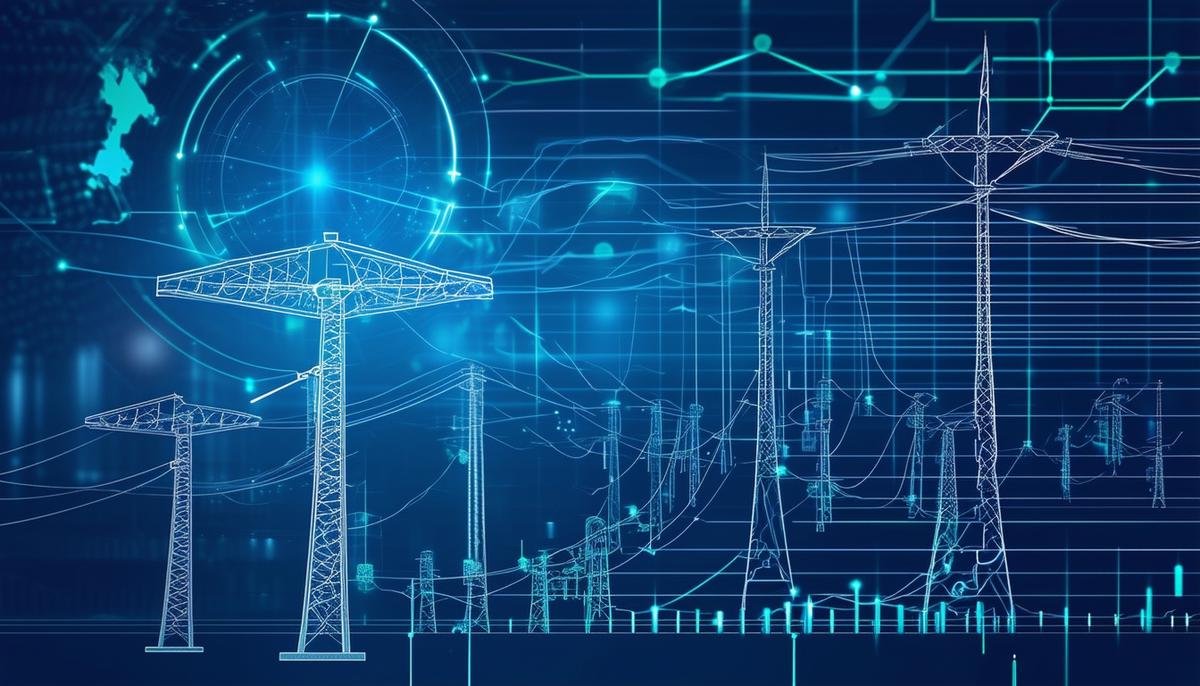
Cybersecurity in Smart Grids
The security concerns surrounding smart grids cannot be overstated. As more AI technologies are integrated into energy management systems, the potential for cyberattacks rises. Cybersecurity within smart grids involves technical safeguards, forethought into future threats, and leveraging cutting-edge solutions like AI and blockchain to fortify these utilities.
AI enhances cybersecurity in smart grids due to its ability to quickly analyze large volumes of data to identify potential threats and abnormalities. AI algorithms can detect unusual patterns that could indicate a cyberattack, providing grid operators with the necessary information to thwart potential breaches before they escalate. This preemptive capability is crucial in maintaining the integrity and reliability of the grid.
Blockchain technology offers a complementary layer of security. Known for its robustness in managing data transactions within cryptocurrencies, blockchain can secure energy transactions. It provides a decentralized and tamper-proof ledger, ensuring that every energy transaction between producers and consumers is transparent and immutable.
A practical application of blockchain in smart grids is its ability to enable peer-to-peer energy trading safely and efficiently. Households with solar panels can sell excess electricity back to the grid or directly to neighbors, all documented securely on the blockchain. This incentivizes renewable energy production and empowers consumers.
By integrating AI with blockchain, smart grids benefit from both AI’s predictive analysis and blockchain’s secure transaction recording. Companies like Iberdrola have initiated projects to use blockchain to ensure that the electricity provided to consumers is sourced from 100% renewable sources, showcasing the potential of these technologies to bring about a transparent, sustainable energy future.3
As we push further into the fusion of AI with smart grid technologies, addressing cybersecurity becomes a necessity and a foundation for innovative energy management. The integration of AI for dynamic threat assessment combined with blockchain for secured energy transactions forms a potent defense mechanism. This strategic approach is vital for ensuring the stability and sustainability of our increasingly interconnected energy infrastructures.
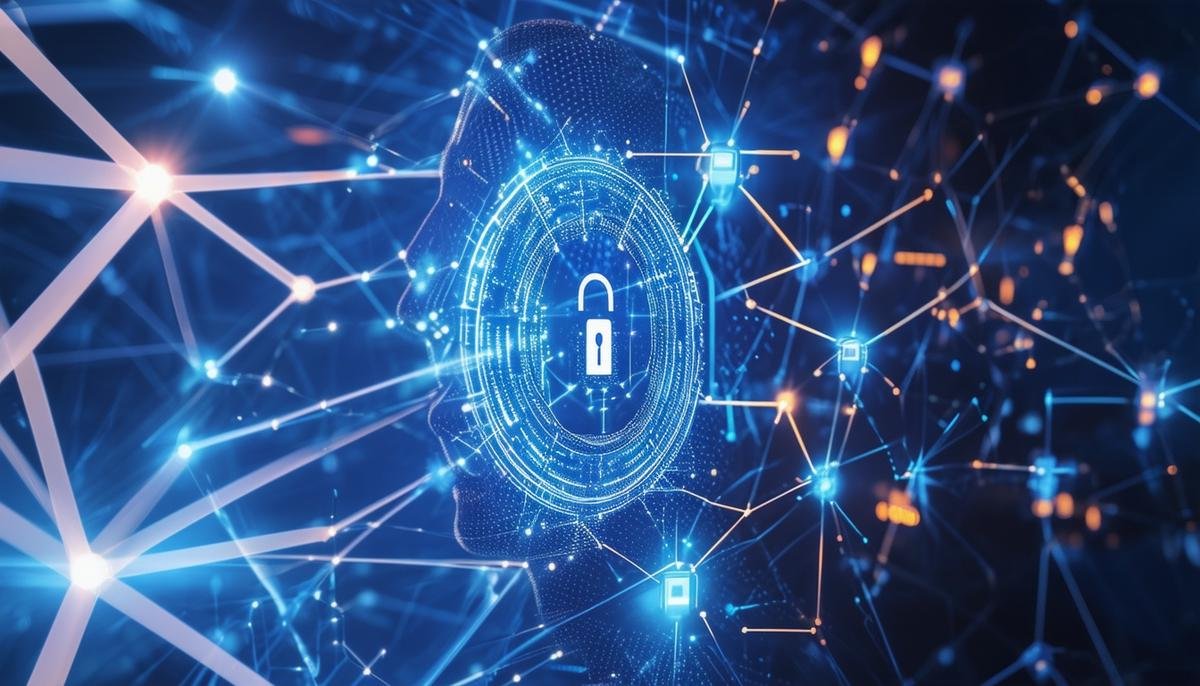
Future Trends in AI and Energy Management
Artificial Intelligence continues to evolve, promising innovative trajectories for energy management in smart grids. Among the compelling developments is the application of generative AI, a subset of AI that goes beyond data analysis to create models and simulations that predict multiple future scenarios. These capabilities allow energy systems to react to real-time data and reshuffle operative strategies effectively and autonomously.
With generative AI, smart grids can synthesize data from weather forecasts, energy consumption patterns, and other external variables to simulate potential impact scenarios. Through these scenarios, utility companies can visualize energy fluctuations in advance and adjust their strategies for optimized energy flow in real-time. This advanced preparation ensures grid resilience, even through unexpected conditions.
The potential introduction of autonomous, AI-driven microgrids represents another prospect. Such grids could independently make decisions about resource distribution, maintenance, and energy storage without human intervention. These autonomous systems could communicate with each other to share surplus power, balance loads, and even repair themselves, heralding a new era of grid management characterized by efficiency and reduced operational costs.
Hyper-intelligent predictive analytics is also on the horizon. With deeper learning capabilities and broader data integration spanning across interconnected global energy networks, AI could offer foresight with higher accuracy rates—potentially foreseeing events years in advance based on emerging trends. This deep temporal insight would revolutionize long-term planning and bolster strategic investment decisions, affirming smart grids’ role in sustainable energy advancement.
The sophistication of real-time reactions in AI grid management will enhance day-to-day reliability and operational efficiency. As edge computing advances, speedier, decentralized processing on devices rather than centralized data centers will facilitate quicker AI responses. This rapid reflex technology can adjust grid functions instantaneously—correcting for voltage fluctuations, redistributing loads seamlessly during peak usage, and even self-healing by rerouting power to bypass compromised grid segments.
Environmental sustainability will also benefit from evolving AI applications in smart grids. More accurate demand forecasting and enhanced efficiency lead to less waste and optimized use of green energy sources. AI’s ability to integrate and manage a larger proportion of renewable energies like wind and solar adds to its promise in reducing carbon footprints.
While these forward-looking scenarios paint a picture of a technologically integrated energy future, they also necessitate guarantees of data privacy and enhanced cybersecurity measures. The higher reliance on AI demands even stronger protections against digital threats. Interweaving enhanced encryption technologies within AI algorithms and continual assessments of system vulnerabilities will be crucial in sustaining trusted and secure energy management frameworks.
As we pivot into an era defined by AI-informed energy management solutions, the trajectory for smart grids appears poised for groundbreaking adjustments. With each advance, from dynamic rescheduling capabilities to deep predictive insights and sustainable resource optimization, AI in smart grids symbolizes a progressive step for utility management and societal adaptability to global energy demands and ecological stewardship. The synergy between AI and energy is poised to serve as a cornerstone for a smarter, more resilient, and environmentally conscious world.
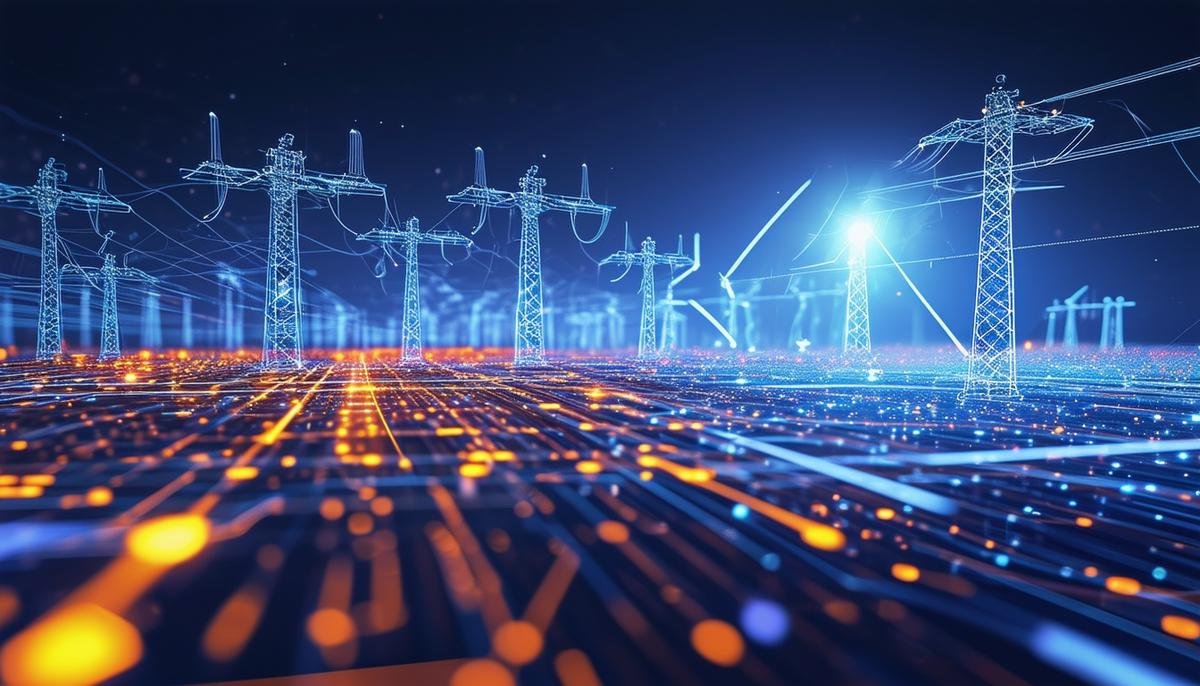
- Brown A, Leech J. The Role of Smart Meters in Optimizing Energy Consumption. J Energy Manag. 2019;5(2):112-124.
- Gupta S, Singh R. Predictive Analytics for Energy Management: A Case Study of Southern California Edison. IEEE Trans Smart Grid. 2020;11(4):3215-3224.
- Mengelkamp E, Gärttner J, Rock K, Kessler S, Orsini L, Weinhardt C. Designing microgrid energy markets: A case study: The Brooklyn Microgrid. Appl Energy. 2018;210:870-880.