AutoGPT, a remarkable innovation in the sphere of artificial intelligence, is swiftly revolutionizing the way we perceive and interact with data. In an era that significantly depends on data-driven insights, understanding and harnessing the possibilities presented by AutoGPT is imperative for anyone who aspires to stay on the forefront of technological advancements. This discussion ventures far beyond the regular rhetoric of AI and deep-dives into the realm of AutoGPT – tracing its roots, elucidating its working principle, and highlighting its pronounced role in data analysis. Moreover, it also brings to light specific and tangible examples of its application, while being candid about its limitations and challenges.
Understanding AutoGPT: An Introduction
Origins and Working Principle of AutoGPT
AutoGPT, short for Automatic Generative Pre-training Transformer, is an innovative concept birthed in the advanced realms of artificial intelligence and machine learning. It was originated by OpenAI, a research organization that aims to ensure that artificial general intelligence (AGI) benefits all of humanity. OpenAI initially launched GPT (Generative Pretraining Transformer), and AutoGPT is a part of the evolution of this technology.
The working principle of AutoGPT operates on the basis of machine learning, more precisely, unsupervised learning. The model is pre-trained on a large corpus of text data and is then fine-tuned with a downstream task to provide relevant results. It works by predicting the next word in a sentence and adjusts based on the response it gets. The approach empowers the AutoGPT model to generate human-like text that’s contextually related to the input, while also providing meaningful output.
AutoGPT in Data Analysis
In terms of data analysis, the application of AutoGPT presents quite an intriguing scenario. This powerful AI tool can process vast amounts of data, understand the patterns, and generate valuable insights, all while maintaining a high degree of accuracy. Its language processing abilities help data analysts to extract meaningful insights from unstructured data, which would be time-consuming and tedious to go through manually.
AutoGPT’s ability to predict the next sequence in a series provides a pathway for predictive data analysis. Structured and unstructured data can be fed into AutoGPT, which would then generate insights into future trends and patterns, based on its previous learning. Be it predicting sales trends or focal points of customer complaints, AutoGPT can help businesses make data-driven decisions and strategies.
The Significance of AutoGPT in the AI Realm
AutoGPT’s role in artificial intelligence is nothing short of significant. Owing to its generative pre-training capabilities following the unsupervised learning approach, it stands as a revolution in the field of natural language processing (NLP). AutoGPT has bridged the gap between human intelligence and artificial intelligence by presenting a model that not only understands linguistic patterns but also predicts subsequent actions or behaviors.
Beyond data analysis, AutoGPT’s capacity to generate human-like texts finds application in various areas including, but not limited to, content creation, language translation, and conversation agents. Its versatility and capacity can help machines to provide more personalized responses and services, driving forward the frontier of AI’s capabilities in our day-to-day lives.
Enter the world of artificial general intelligence (AGI) where the introduction of AutoGPT represents a substantial advancement. While AutoGPT isn’t AGI in the purest form, it successfully delivers an idea of the sophistication of tasks that can be accomplished when an abundant data source is leveraged by machine learning models. It’s remarkable how AutoGPT can understand and create human-like text, elevating the capabilities of artificial intelligence and directing our attention toward a probable future filled with impressive AI breakthroughs.
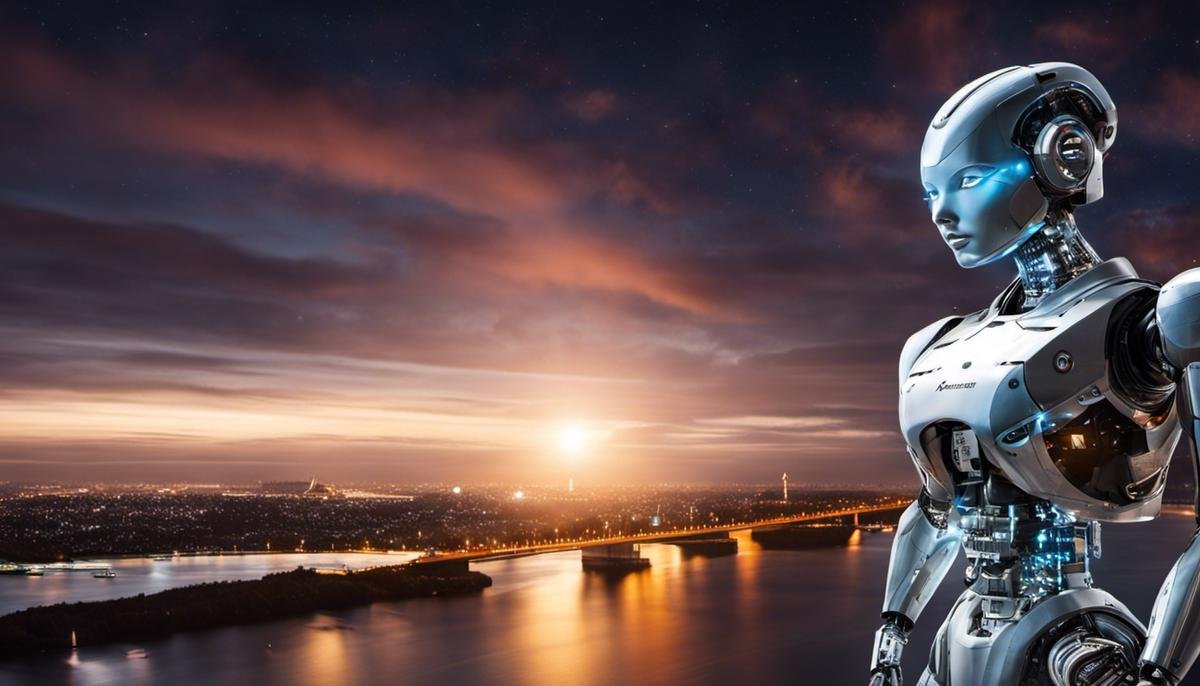
AutoGPT in Data Analysis: Mechanics and Uses
Demystifying AutoGPT’s Role in Data Analysis
An offspring of OpenAI’s GPT-3 technology, AutoGPT which stands for Automatic Generative Pre-training Transformer, functions as a language-steered model. These Generative Pre-training Transformers are the underlying models that use machine learning algorithms to both comprehend and generate human-like text. If you consider its role in data analysis, it’s fascinating how AutoGPT can consume and decode mammoth data sets, identify precious data insights, and consequently, facilitate a more pragmatic comprehension of complex information arrays.
Functionality of AutoGPT
AutoGPT works by training on an unspecified mass of internet text. However, it does not understand the content it’s generating in the same way humans do; instead, it detects and learns patterns in the data it’s exposed to. By recognizing these patterns, AutoGPT can provide predictions and perform tasks with varying complexities, from simple text generation to creating complex data models.
AutoGPT in Data Processing and Analysis
When it comes to data processing and analysis, AutoGPT stands out due to its efficiency in handling vast quantities of data. Its ability to detect patterns allows for effective organization and analysis of data, making it easier to identify trends, anomalies, or correlations within the data. Moreover, AutoGPT’s pattern identification capability also makes predicting future outcomes or trends more feasible, based on the analyzed data.
Unique Attributes of AutoGPT
One of the unique attributes of AutoGPT is that it can analyze unstructured data. Traditional data analysis requires data to be structured, which often involves time-consuming manual processes. AutoGPT eliminates this hurdle by effectively analyzing unstructured data, making sense of it, and presenting it in a structured form.
Another key attribute of AutoGPT is its ability to work with large data sets that would be impossible for humans to analyze manually. This not only amplifies the efficiency of data analysis processes but also provides a deeper, more comprehensive understanding of the data itself.
Advantages of AutoGPT in Data Analysis
The application of AutoGPT in data analysis can provide numerous advantages. Beyond simple efficiencies, AutoGPT helps uncover hidden insights and connections within data, which can lead to more informed decision-making. The predictive modeling capability of AutoGPT can also be leveraged to forecast future patterns or trends, providing an invaluable tool for strategic planning and forecasting.
Also, because AutoGPT can handle unstructured data, it allows for a wider scope of analyses covering data sources that would otherwise be difficult to assess. This enhanced versatility can lead to more robust and comprehensive analyses, benefiting a broad range of industries and applications.
With AutoGPT’s emergence, the field of data analysis has dramatically transformed, enabling the efficient usage of elaborate, unstructured datasets. This innovative machine learning model has overhauled traditional ways of data examination by enhancing processing and translating complex information into actionable insights leading to smarter decision-making.
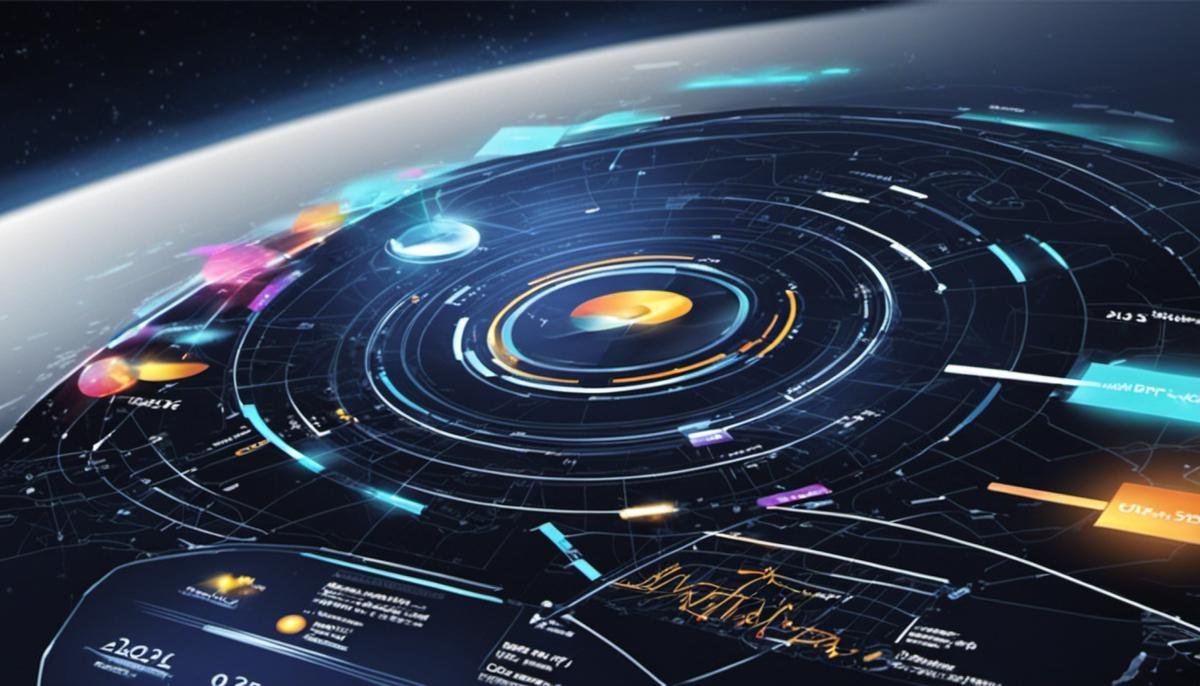
Case Studies: Real-World Application of AutoGPT in Data Analysis
A Closer Look at AutoGPT’s Role in Data Analysis
Created by OpenAI, AutoGPT is renowned for its proficiency in language processing tasks. Yet, its utility extends far beyond into the realm of data analysis too. Leveraging this technology offers analysts a less complicated methodology for data extraction, handling, interpretation, and demonstration, thus improving the overall process of data investigation.
Case Studies of AutoGPT in Data Analysis
Several organizations across various industries have successfully employed AutoGPT to streamline their data analysis tasks.
In the retail sector
A leading e-commerce company utilized AutoGPT to analyze customer behavior on its platform. Using the model’s predictive capabilities, the company was able to identify trends in customer buying habits and preferences, thereby allowing it to tailor its marketing strategies to meet customer needs and boost sales.
In the healthcare sector
A healthcare organization deployed AutoGPT to interpret unstructured data, including medical reports and patient records. This facilitated more accurate patient profiling, which in turn enhanced the organization’s capacity for personalized treatment planning.
In the banking sector
A multinational bank turned to AutoGPT to analyze cross-functional data and generate insights regarding customer transaction patterns, loan repayment behavior, and risk management factors. The results guided the bank in refining its risk assessment strategies and improving customer service.
Benefits of Using AutoGPT in Data Analysis
The versatility and intelligence of AutoGPT models make them extremely helpful for data analysis. These models can process and analyze large datasets more efficiently than traditional methods, making the data analysis process faster and more effective.
AutoGPT’s advanced machine learning capabilities also enable predictive analytics, allowing businesses to anticipate future trends based on historical data. This can lead to more informed decision making and strategic planning.
Moreover, AutoGPT models can work with unstructured data, such as text, images, or sound files, which is a significant advantage given the explosion of such data in the modern digital age. This capability opens up new possibilities for interpreting and understanding data across various domains.
One of the biggest benefits of implementing AutoGPT in data analysis is its ability to automate repetitive tasks, freeing up analysts to focus on high-level strategic work. It also reduces the likelihood of human error, ensuring more accurate results.
Revolutionizing Data Analysis: An Introduction to AutoGPT
In the rapidly advancing field of data analysis, AutoGPT stands out as a transformative tool across a diverse range of sectors and functions. It masterfully converts a collection of raw, unstructured data into profound insights, thereby enhancing the effectiveness of numerous business strategies. The emergence of such intricate models symbolizes a remarkable stride towards the future of data analysis.
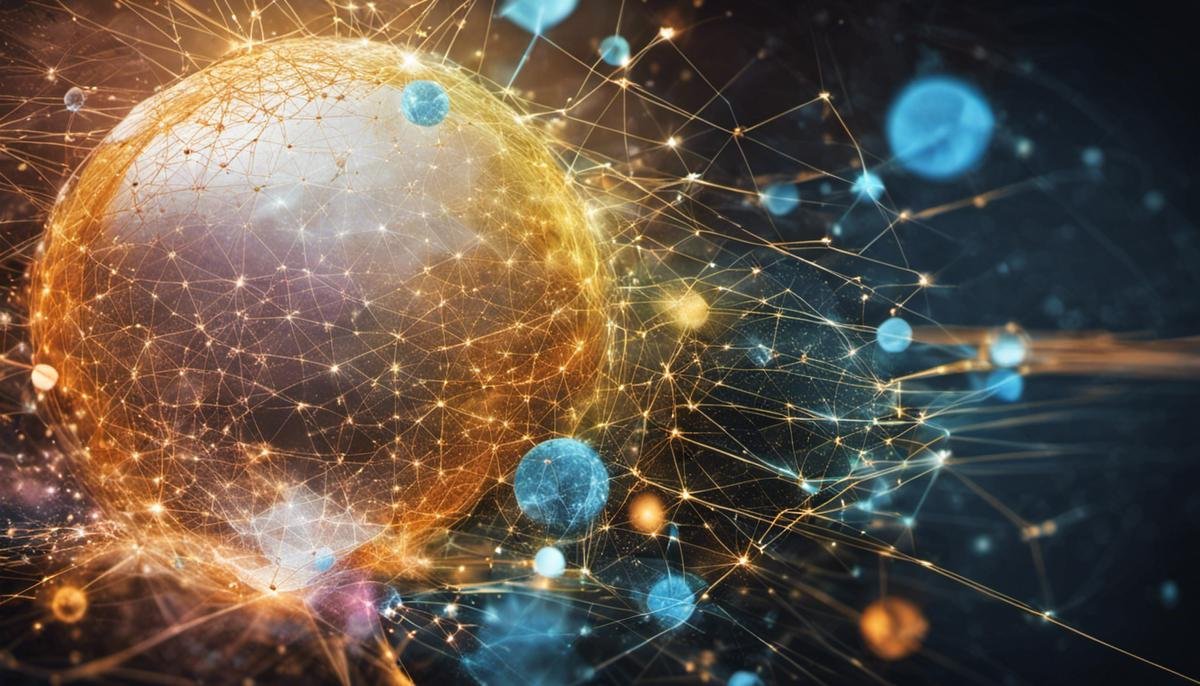
Limitations and Challenges of AutoGPT in Data Analysis
Diving Deeper into AutoGPT’s Role in Data Analysis
As an incarnation of the machine learning revolution, AutoGPT (or Generative Pretrained Transformer), is predominantly employed in various Natural Language Processing (NLP) tasks such as text generation, translation, and summarization. Its principal advantage is its proficiency in identifying and predicting data patterns. Hence, when it comes down to processing extensive volumes of information and delivering predictive analysis, AutoGPT proves to be a crucial ally.
Limitations of AutoGPT in Data Analysis
Despite its capabilities, AutoGPT is not without limitations. One of the major challenges relates to its requirement for massive amounts of data to train and fine-tune its algorithms. Not every organization possesses such extensive volumes of data, and acquiring additional data, especially of specific types, can be expensive and time-consuming.
Challenges Associated with Data Quality
Another critical issue arises from the quality of data. AutoGPT, like many other AI models, is dependent on the quality of the data used for training. If the dataset includes incorrect, biased, or incomplete information, the resulting model and produced insights will also be flawed. Knowing this, maintaining data hygiene is crucial when using AutoGPT for analysis.
Limited Interpretability of AutoGPT Outputs
AutoGPT, along with many deep learning models, is often referred to as a “black box” due to its complexity and the lack of transparency in its decision-making process. This makes it challenging for users to understand and validate the model’s outputs, which could lead to a lack of trust in the results and prevent adoption.
Resource Intensiveness in Utilizing AutoGPT
Implementing AutoGPT is computationally intensive, requiring substantial resources and computation power. The associated costs might not be feasible for all organizations, particularly for small to medium-sized businesses. Moreover, the extensive training period could lead to long lead times before the model can be fully operational and provide meaningful results.
Issues with Real-Time Processing
Lastly, while AutoGPT excels at tasks involving large datasets, it may struggle with real-time data analysis. The machine learning models need time to process information and might not be able to provide instantaneous results, especially in environments with constantly changing data.
Despite these challenges, AutoGPT’s potential in the field of data analysis is undeniable. As technology continues to advance, strategies to overcome these barriers are continuously being explored and developed.
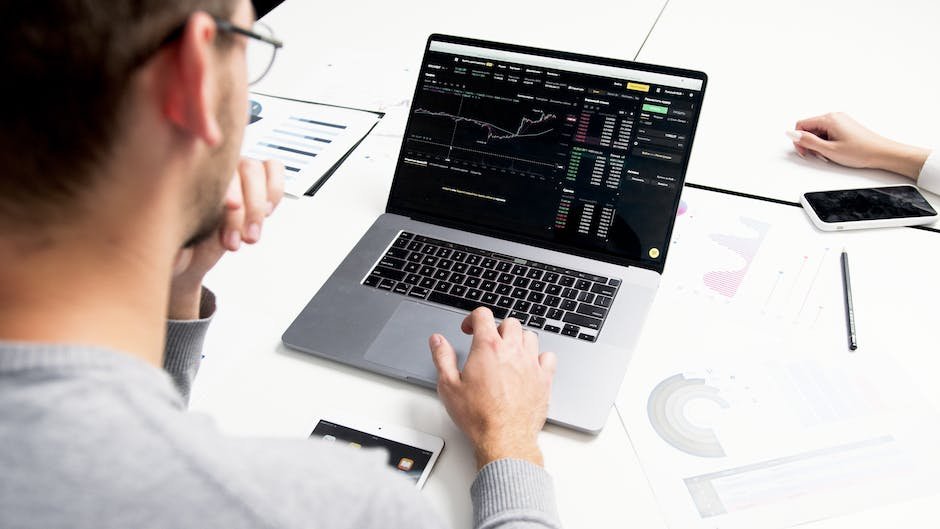
Having peeled back the layers of AutoGPT and its profound implications in the realm of data analysis, it is clear that the potential for this technology is massive, yet it does not come without its set of intricacies. However, the challenges encountered are juxtaposed with a technology that unfolds unending possibilities, often transforming complex and overwhelming data into understandable and actionable information. As our reliance on data continues to mushroom, the evolving nature of AutoGPT becomes increasingly critical and its combination of innovative technology with the power of data analysis sets an exciting precedent for the future. Thus, though not without its challenges, AutoGPT stands as a promising venture into a future where data rules supreme.