Evolution of DALL-E
DALL-E, designed by OpenAI, allows users to generate images from textual descriptions. Its early success hinged on evolving generative adversarial network technology, which had grown from rudimentary designs to complex systems capable of stunning image synthesis.
OpenAI introduced critical updates to DALL-E, enhancing its resolution capabilities and the subtlety with which it handled fine details. This marked an evolution from generating mere representations to creating sharp, detailed, and more nuanced images.
DALL-E 2 was released in 2022, boasting an improved understanding of spatial relationships and more sophisticated text-to-image functionalities. This version promised greater coherence in visual elements when generating artworks from text descriptions containing specific and multiple instructions.
Adjustments continued, focusing on fine-tuning the model’s ability to handle compositions and abstract concepts. These enhancements addressed artists’ and designers’ needs to create intricate and abstract pieces without losing the essence of the intended artwork.
Ethical guidelines surrounding the use of generated images were rigorously defined to curb the generation of harmful content and ensure respectful and responsible use of AI technology in art.
DALL-E 3 is rumored to be in the works, with speculation suggesting it will include even more powerful tools for precision editing, enabling artists to tweak AI-generated pieces with unprecedented control.
Each version of DALL-E improved visually and expanded the language understanding abilities of the AI, enabling it to interpret and execute increasingly complex prompts. This evolution underscores a future where DALL-E could serve as a creative partner, offering artists a new medium through which to express their visions.
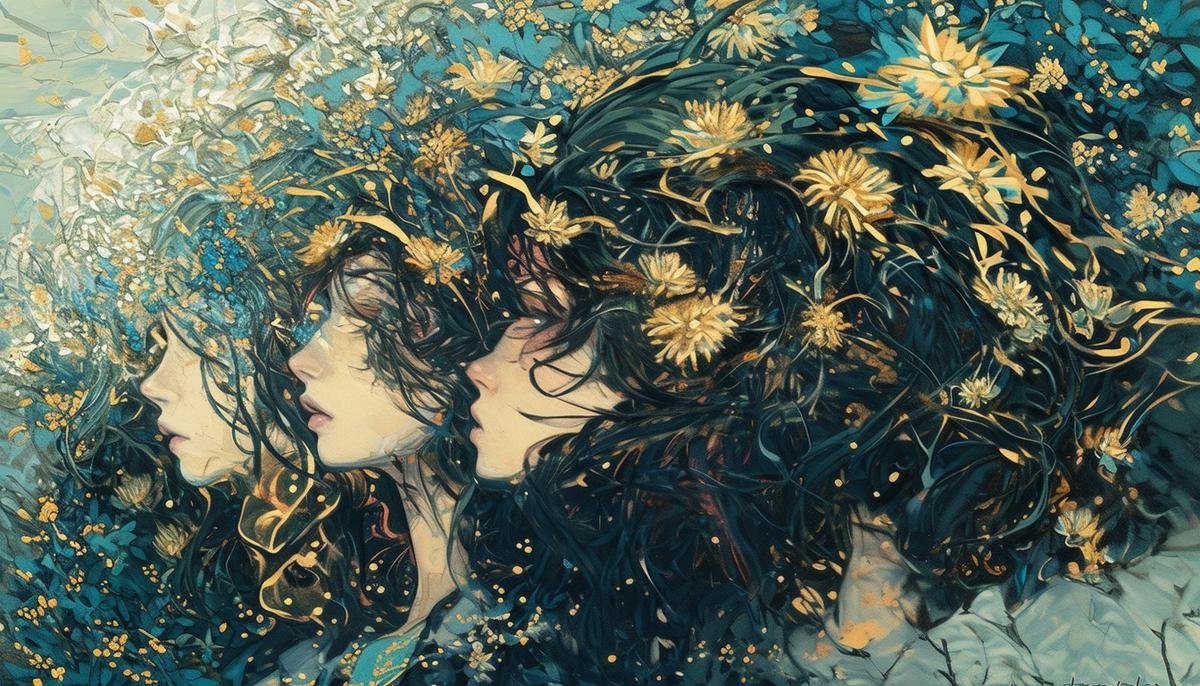
Impact on Digital Art Galleries
The influence of DALL-E on digital art galleries is profound, reshaping how art is created, curated, displayed, and sold. Digital galleries employ AI like DALL-E to create captivating exhibition pieces and curate shows, attracting diverse audiences by offering unique, personalized viewing experiences.
AI-generated art mechanisms like DALL-E are a game-changer for digital gallery operations. By lowering the barriers to entry for creating high-quality, innovative artworks, these AI tools allow digital galleries to continually refresh their offerings at a fraction of the traditional cost and time. The ability for galleries to quickly produce and display new thematic exhibits in response to current trends or world events adds dynamism to their schedules.
Digital art pieces, particularly AI-generated artworks, often do not adhere to traditional pricing guidelines; their values are influenced as much by algorithmic originality as by aesthetic appeal or artistic name.
Artists and creators see these tools as collaborators that challenge and extend their creative process, pushing artistic boundaries. This synergetic collaboration often results in artworks that are poignant reflections on technology’s role in society and culture.
As optimistic as the impact of DALL-E seems on the digital art world, it also promulgates debates about authenticity and authorship. Regulatory frameworks and ethical guidelines are vigorously pursued alongside technological enhancements to ensure the digital artspace remains a harmonious blend of innovation and originality.
In such an environment, galleries need to be custodians of art and tech-savvy innovators who harness technologies conscientiously to preserve the soul of artistic endeavor. These integrations suggest a vibrant symbiosis between human ingenuity and machine intelligence in a digitally native ecosystem that redefines cultural consumption for future generations.
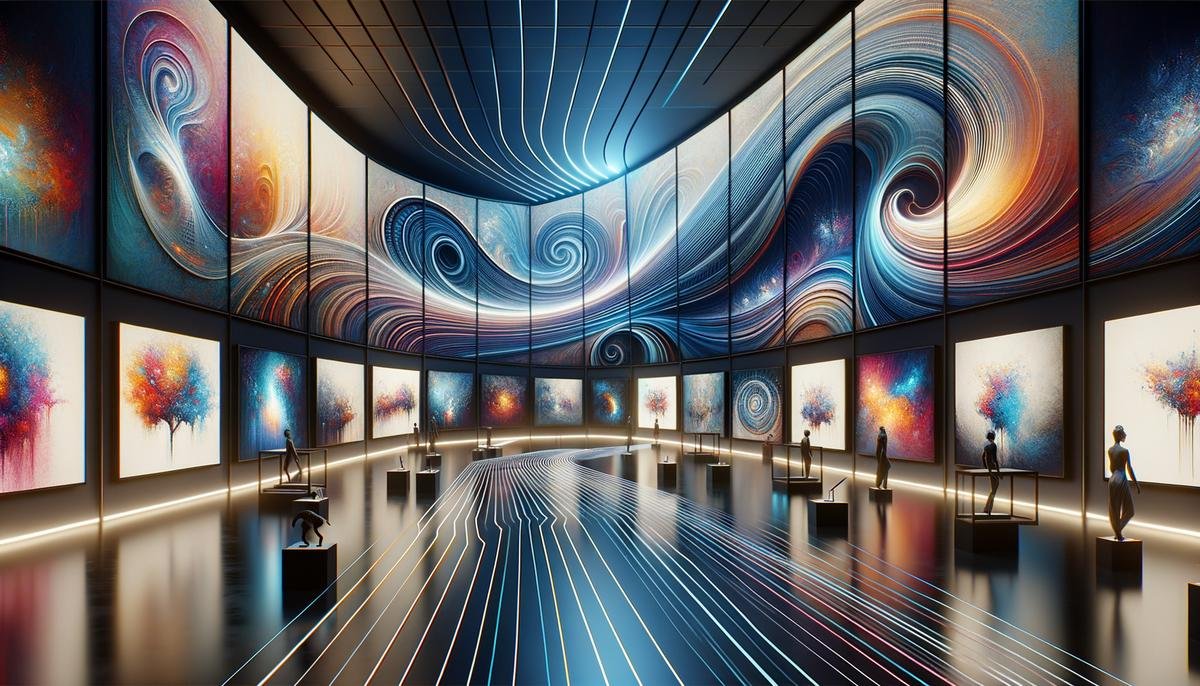
Technological Innovations
DALL-E’s magic springs from deep learning technologies that utilize neural networks modeled loosely on the human brain’s architecture. These neural networks consist of layers of nodes, or “neurons,” each capable of performing specific calculations. Input data—like text prompts submitted by users—passes through these layers, with each node responding according to its programmed or learned function, ultimately translating descriptive text into complex, layered imagery.
The heart of DALL-E’s ability to create art lies in a type of neural network known as a Generative Adversarial Network (GAN). This system includes two main components:
- The Generator: works to produce images that are increasingly indistinguishable from genuine artworks, learning from each attempt to better fool its counterpart.
- The Discriminator: evaluates these generated images against a training set of real artworks, learning to detect nuances that differentiate machine-made from authentic.
This adversarial process continues iteratively, with both sides evolving until the Generator produces results so convincing that the Discriminator can’t reliably tell them apart from human-made works.
Further sharpening DALL-E’s capabilities are machine learning algorithms known as transformers. Initially developed for natural language processing tasks, transformers manage the relationships and contextual nuances in data. In DALL-E’s case, these transformers interpret text prompts, determining from linguistic cues what elements, styles, and themes are expected in the resulting images, allowing for a richer understanding and rendering of complex requests that can involve multiple elements and sophisticated inter-relationships.
Additionally, as OpenAI continuously feeds more curated and diverse datasets into DALL-E’s training regimen, the AI learns from a broader spectrum of artistic styles and cultural iconography. This boosts the model’s adaptive capacity to generate fresh, contextually relevant artworks and enhances its sensitivity to subtler elements of human creativity like satire, symbolism, and thematic depth.
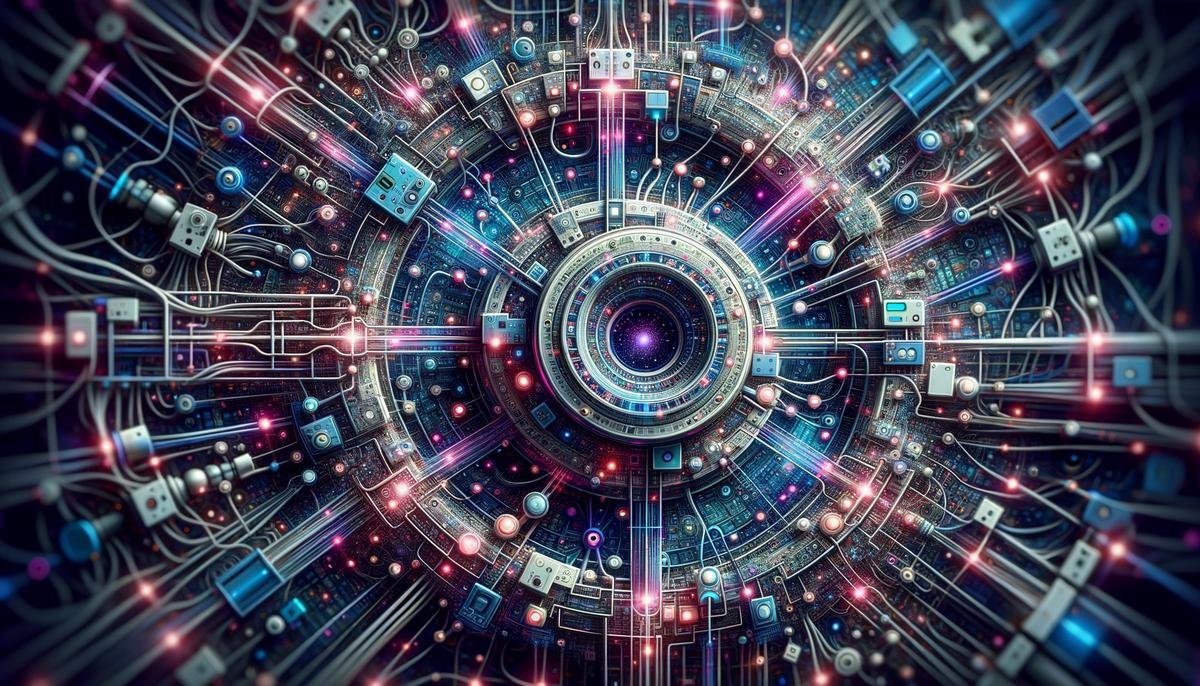
Comparative Analysis with Other AI Tools
While DALL-E has made significant strides in the AI art generation space, it stands alongside formidable counterparts such as Midjourney and Stable Diffusion, each bringing unique attributes to the table.
Midjourney transforms textual prompts into vivid images but positions itself distinctly with a stylistic inclination towards more ethereal and visionary art. Its network seems particularly adept at creating dream-like scenes that border on the surreal, distinguishing its aesthetic from DALL-E’s often more literal interpretations.
Stable Diffusion goes yet another direction emphasizing customization and democratization of AI art generation. It allows anyone to run the model locally on their computer, granting users a high level of control over the image generation process. This tool offers versatility and personalization in the artistic process by permitting tweaks to the model itself.
Regarding output quality, DALL-E is known for producing remarkably clear and precise images that maintain fidelity even at high resolutions. This makes it especially suitable for applications that require detailed, realistic renderings of envisioned scenes based on textual descriptions. Contrastingly, while Stable Diffusion excels in delivering artistically varied outputs and supports extensive user customization, its results can sometimes exhibit a style that is less consistent than DALL-E’s, which might be a trade-off for its versatility.
Understanding these nuances between DALL-E, Midjourney, and Stable Diffusion is key for anyone immersed in the digital creation space or those venturing into AI-assisted art for the first time. Each tool has carved out its niche, catering to different segments of digital artists and creators based on artistic needs, interaction style preferences, and technical capabilities.
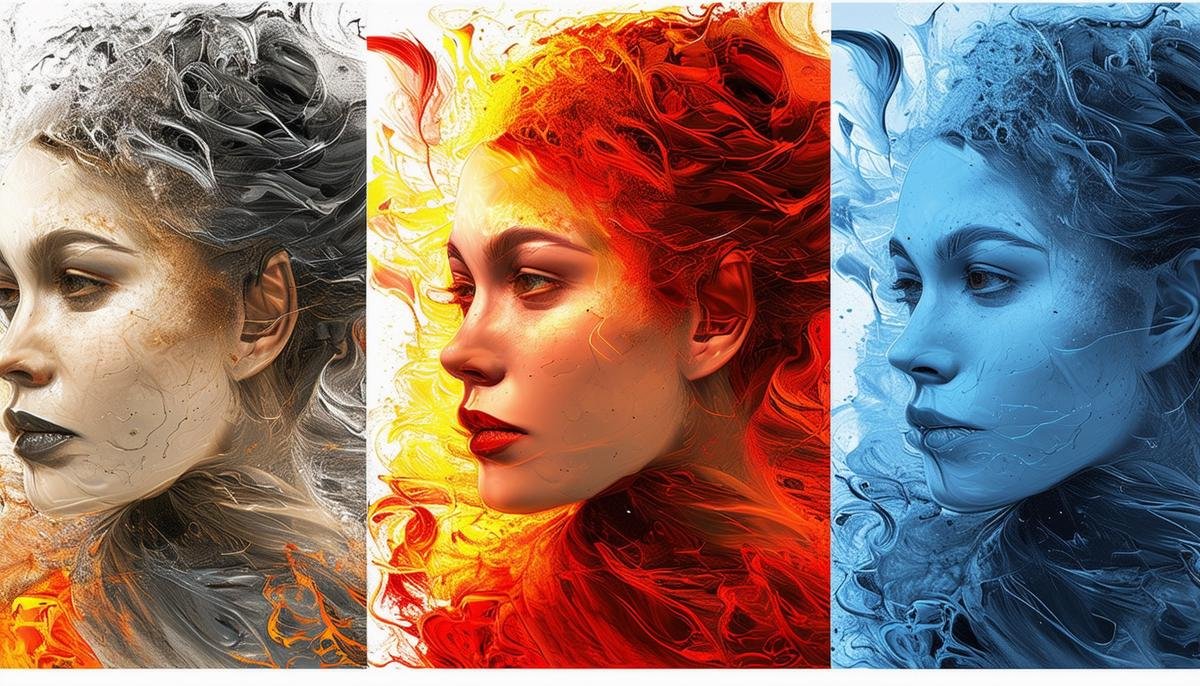
Future Prospects
Looking ahead, the potential applications and developments for DALL-E in the art world appear boundless. As this technology continues to mature, we might speculate on several emerging trends and future features that could further revolutionize the landscape of digital and generative art.
One exciting direction is the integration of virtual reality (VR) and augmented reality (AR) with DALL-E-generated artworks. Imagine artists and galleries adopting VR to create fully immersive digital exhibits where viewers can step into and interact with scenes from AI-generated pieces. This could be complemented by AR applications that overlay digital art, created on-the-fly by AI, into our real-world environments.
AI collaborations could evolve as well, with DALL-E being used to generate initial art concepts which human artists can refine or reinterpret for hybrid works. Such collaborations could push new art movements or styles that blend traditional techniques with AI’s unique qualities.
Another forthcoming feature could include advanced sentiment analysis capabilities where DALL-E’s output reflects more complex human emotions and narratives, learned through deep learning from vast repositories of global literature, music, and visual arts. This would increase the emotional depth of AI-generated artwork.
Real-time art generation might become commonplace, enabling audiences at live performances or events to suggest themes or elements that DALL-E incorporates instantaneously into stage backdrops or interactive displays.
DALL-E might also further democratize art creation through enhanced user-friendly interfaces that cater to non-technical users. We could see simplified versions of the technology made available on smartphones or as cloud services.
We should also anticipate continual discussions and evolutions surrounding copyright and creative rights pertaining to AI-generated art. As legal frameworks mature alongside AI technologies, there could be new structures to recognize and commercialize AI-generated artwork responsibly.1
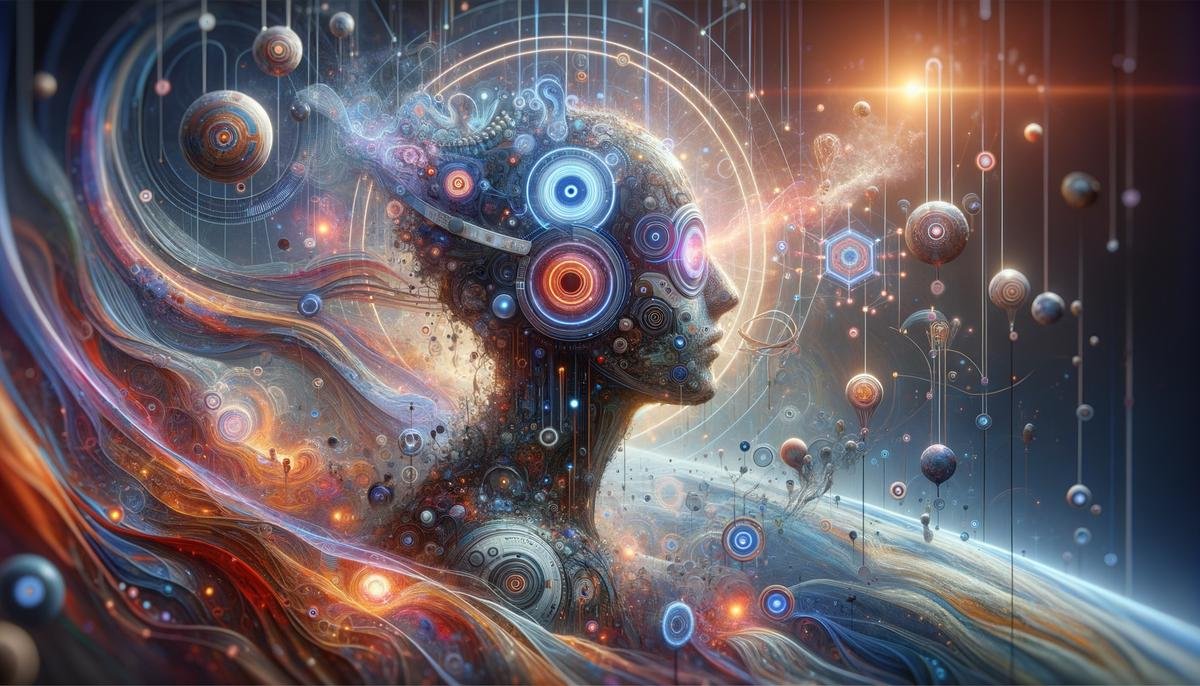
In conclusion, DALL-E’s journey from a novel AI experiment to a sophisticated tool capable of collaborating with human creativity underscores its potential to redefine artistic expression. As we look forward, the integration of such technologies promises to further blur the lines between human and machine-generated art, enriching the cultural landscape and expanding the possibilities for creators worldwide.
- Zhu J, Park T, Isola P, Efros AA. Unpaired Image-to-Image Translation using Cycle-Consistent Adversarial Networks. In: 2017 IEEE International Conference on Computer Vision (ICCV). IEEE; 2017:2242-2251.