Predictive Maintenance
The integration of machine learning algorithms and real-time data analytics into predictive maintenance is transforming traditional maintenance programs in manufacturing. These AI systems analyze sensor data from factory floors, detecting irregular patterns that signal potential equipment malfunctions. By identifying issues early, manufacturers can schedule precise maintenance activities, preventing downtime and boosting productivity.
AI can also adjust machine settings to align with changing environmental conditions, such as temperature or humidity fluctuations, defending equipment from immediate threats and reducing wear and tear. Predictive models empower management decisions by forecasting various failure scenarios, guiding timely interventions that save maintenance costs and minimize revenue loss from unplanned downtime.
AI's predictive capabilities extend to scheduling optimal maintenance times across entire equipment fleets, ensuring minimal disruption to production cycles. The system continuously learns from the outcomes of maintenance actions, refining its predictive accuracy over time. This allows manufacturers to focus their expertise on critical issues flagged by AI, augmenting decision-making with intelligent, data-driven insights.
Quality Control and Process Optimization
Advanced computer vision systems powered by AI are revolutionizing quality control in manufacturing. These systems scrutinize products on assembly lines with exceptional accuracy, detecting even the slightest defects that may escape human inspection. By continuously monitoring production, AI algorithms can identify and flag items that don't meet quality standards, reducing the risk of subpar products reaching consumers.
AI's ability to analyze comprehensive datasets from various stages of the manufacturing process enables it to spot trends and inefficiencies invisible to human operators. By providing actionable insights, AI systems help streamline manufacturing procedures, enhancing efficiency and productivity. For instance, AI might suggest adjustments in machine speed or feed rate based on production run data, leading to improved product quality and reduced material waste.
Real-time adjustments driven by AI provide another layer of process enhancement. Unlike traditional systems that rely on static schedules, AI systems work proactively, anticipating issues using predictive analytics. This allows manufacturers to preemptively modify parameters before inefficiencies turn into problems, fostering a smart manufacturing environment that learns and evolves.
Supply Chain and Inventory Management
AI's predictive capabilities are significantly impacting supply chain logistics and inventory management. By analyzing market trends, consumer behavior patterns, and socio-economic indicators, AI algorithms can forecast future product demands with high accuracy. This enables manufacturers to adjust production schedules and inventory levels in advance, ensuring that supply meets demand without overproduction or stockouts.
Intelligent algorithms optimize inventory by analyzing turnover rates, lead times, and historical sales data to determine optimal stock levels. This tight control over inventory frees up warehouse space and reduces carrying costs, enhancing operational efficiency.
AI also enhances supplier relations and procurement strategies by evaluating supplier performance through data-driven assessments. This insight allows businesses to:
- Negotiate better terms
- Collaborate more effectively with suppliers
- Devise contingency plans for potential supply chain disruptions
- React swiftly to vulnerabilities
The synergy between AI and supply chain logistics is creating proactive strategies that transform traditional operations into dynamic systems capable of adapting to changing market conditions with speed and efficiency.
Generative AI and Product Development
Generative AI is pioneering new approaches to product development, particularly in prototyping and testing. By leveraging deep learning, generative AI can autonomously generate multiple design solutions from predefined parameters and constraints. This rapid exploration of diverse design alternatives pushes the boundaries of innovation and creativity, accelerating the development process.
Each cycle through generative AI's process yields potentially viable solutions evaluated based on efficiency, performance, and cost-effectiveness. This quick turnaround is advantageous during prototyping, where multiple iterations can be produced, tested, and refined swiftly. The technology introduces objectivity, delivering insights based on data and resulting in designs that can outperform traditional ones.
Generative AI's capability lends itself well to complex system designs commonly found in electronics and consumer goods. By simulating real-world conditions and pressures, generative AI can forecast potential fail points and suggest design adaptations, regulating operational risk and pushing product standards to new heights.
The collaborative approach fostered by generative AI reduces feedback loops, improving the efficiency and harmony of development teams. As companies navigate competitive marketplaces, those equipped with generative AI tools secure technological superiority and market responsiveness, positioning themselves as leaders in innovation and industrial evolution.
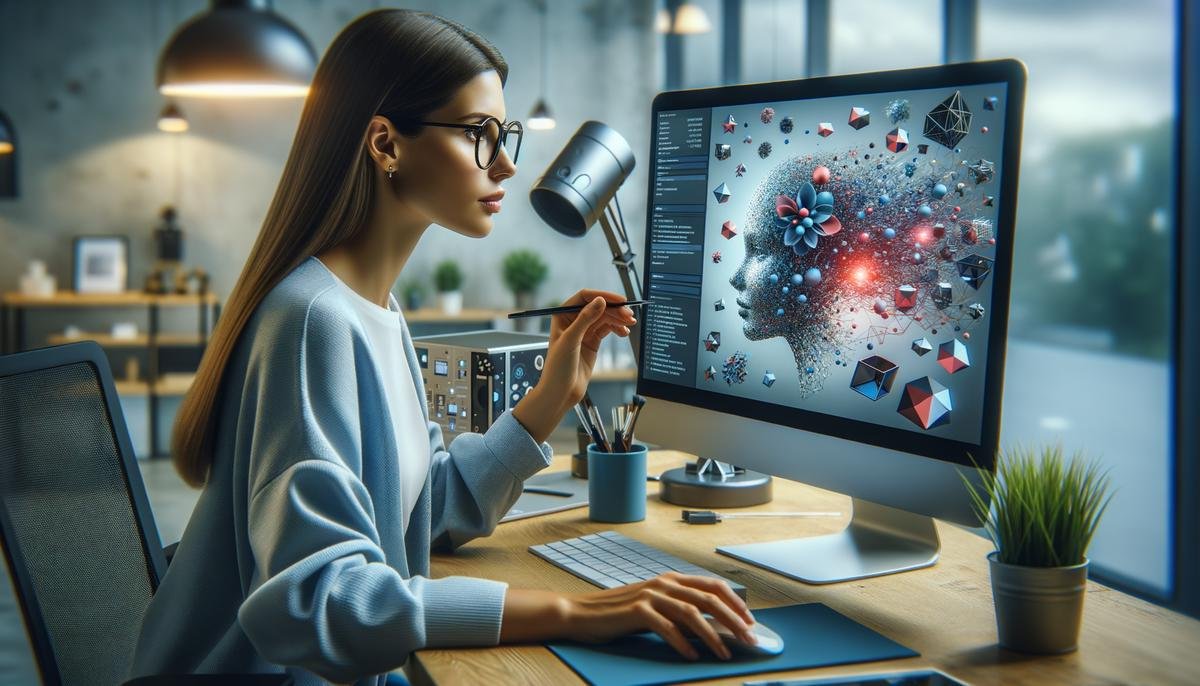
AI-Driven Robotics and Automation
The convergence of AI and robotic technologies is reshaping the production landscape, enabling the automation of a wide range of manufacturing processes with greater precision and agility. Collaborative robots, or cobots, embody the frontier of workplace cohabitation between humans and robots, complementing human actions rather than completely replacing them.
Equipped with sensors, cameras, and self-correcting AI algorithms, cobots assist in automating routines that were previously unfeasible due to safety or technological limitations. Their nuanced intelligence allows them to sense and adapt to human presence, making them invaluable assets in environments requiring a balance between mechanical consistency and human ingenuity.
The intersection of human skills and robotic precision escalates productivity and reduces the potential for error and injury. Cobots can handle repetitive tasks with refined accuracy, while humans focus on more complex quality controls or overrides requiring a level of discernment outside current AI capabilities. This collaboration boosts efficiency and worker morale by diverting them from wearisome tasks.
AI-driven robots also enable flexible production methods, adapting to different products or configurations rapidly through machine vision and adaptive learning. This aligns with lean manufacturing principles and just-in-time production1. Additionally, advanced robots incorporate predictive analytics to forecast equipment failures or maintenance needs, enhancing uptime and resilience in industrial ecosystems.
As technological advances continue to surge, the prospective evolutions in AI-infused robotics promise a renaissance in manufacturing paradigms, enhancing operational facets and revolutionizing the very infrastructure on which industries are built.
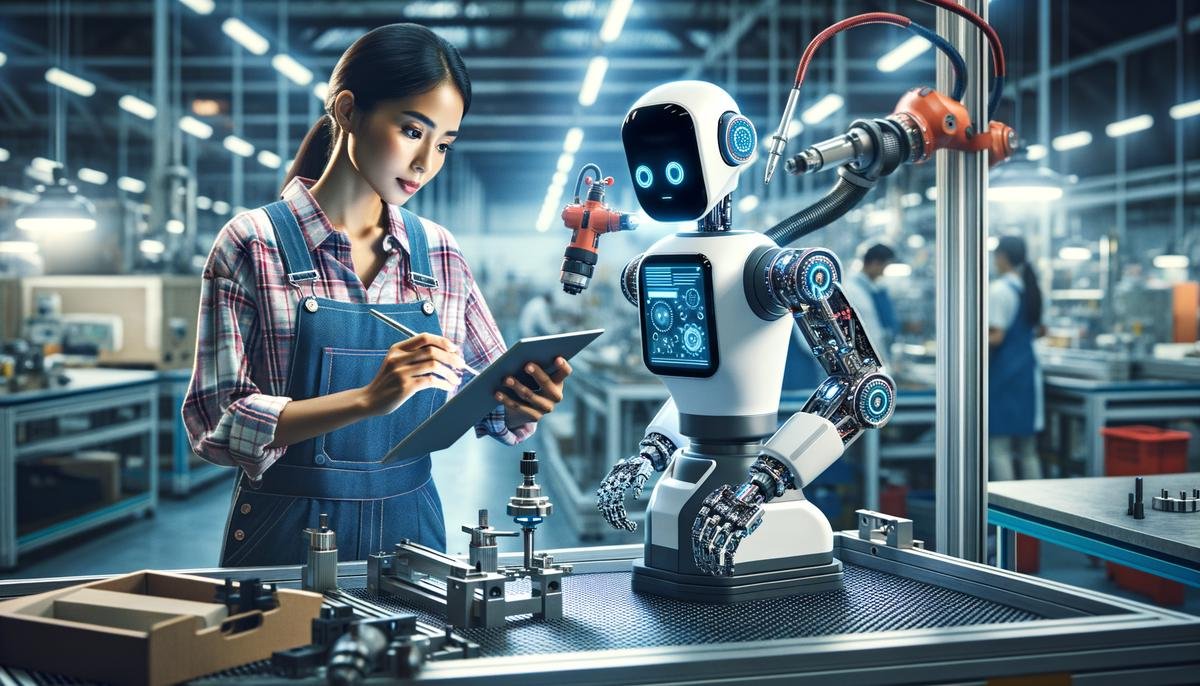
- Krafcik JF. Triumph of the lean production system. MIT Sloan Management Review. 1988;30(1):41.