AI Applications in Wildlife Monitoring
Camera traps are pivotal in this transformation. Washington State University, for example, placed 650 camera traps across over 4,300 square miles in northeastern Washington. These traps generated millions of images that AI analyzed to assess Canada lynx populations. The AI significantly speeds up data processing, reducing the workforce needed for such intensive tasks. The cameras capture countless hours of footage, identifying and recording the presence of shy or nocturnal animals that researchers might never notice in person.
Drones take AI's capabilities a step further by offering a bird's-eye view of habitats. Unlike someone trudging through the forest, drones can cover vast areas swiftly and efficiently. They can fly over areas inaccessible to humans, capturing detailed images and videos from their vantage point. AI algorithms then analyze these images to identify animal species, track their movements, and even detect illegal activities like poaching. Conservation AI has effectively utilized drones to identify species such as pangolins in Uganda and orangutans in Malaysia. They process images at lightning speed, handling tens of thousands of photos every hour, which is far beyond human capacity.
Acoustic sensors add another dimension to wildlife monitoring. They pick up sounds that are often missed by the human ear. For example, researchers have placed recorders in the dense forests of Central Africa to monitor savannah elephants. These sensors capture a variety of sounds, from elephant calls to predator noises. AI then sorts through these sounds, identifying specific species and behaviors. This method was employed by Peter Wrege's team at Cornell University. They recorded the sounds of elephants to detect the presence of both the animals and their predators.1 These sensors are especially useful in environments where visual observation is challenging.
Soundscapes provide another layer of understanding. AI can analyze these soundscapes to quantify biodiversity. In Ecuador's Chocó region, Jörg Müller and his team used AI to dissect animal sounds from diverse habitats. Their findings revealed how different stages of forest recovery influenced species presence. AI managed to identify many bird species, further showcasing its efficiency in processing complex data sets compared to traditional methods.2
These technologies are not without challenges. AI requires enormous amounts of data to function effectively. Collecting and labeling this data can be arduous, especially in remote locations. AI systems may exhibit biases or inaccuracies if the training data is skewed. Continuous monitoring and re-training are essential to maintain accuracy.
Privacy and ethical considerations also come into play. While surveillance is essential for conservation, it must be balanced against potential invasions of privacy, particularly when monitoring near human settlements.
AI-driven technologies like TrailGuard AI, recognized by TIME as one of the best inventions of 2023, are addressing these challenges. TrailGuard AI uses real-time image transmission to prevent poaching and monitor wildlife near human settlements. It's a sophisticated system designed by biologists to serve biologists, and currently operates in numerous protected areas across Asia, Africa, and South America. This technology has successfully thwarted poaching attempts and mitigated human-wildlife conflicts.
The use of AI in wildlife monitoring is growing, driven by platforms like Conservation AI and techniques used by institutions like Washington State University. AI doesn't merely serve to collect data but helps interpret and react to it rapidly. This speed and efficiency are vital in the fight to protect endangered species and their habitats.
AI's role in conservation is bound to expand, requiring careful balance and continuous learning. From camera traps to drones and acoustic sensors, AI offers a promising future for wildlife conservation, bringing advanced technology to the front lines of protecting our planet's biodiversity.
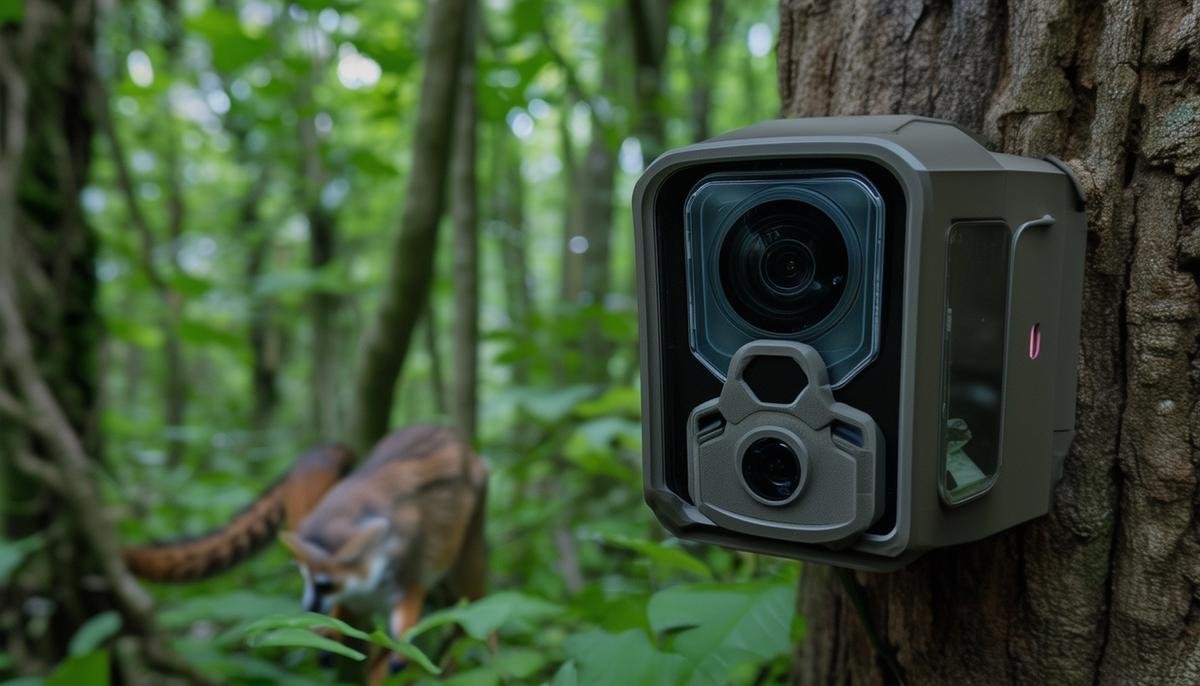
Predictive Modeling and Species Distribution
AI's capability in predictive modeling and species distribution is revolutionizing conservation efforts through its ability to foresee and map environments and behaviors. By analyzing vast datasets, AI algorithms can predict where species are likely to be found and how suitable various habitats are for them. These predictive models are essential in identifying conservation priorities, enabling researchers and policymakers to allocate resources effectively and strategize interventions where they are most needed.
One pertinent example is from the UK-based non-profit Conservation AI, which utilizes machine learning algorithms to forecast species distribution. These models employ historical data on species sightings, climate conditions, vegetation patterns, and other environmental factors. By doing so, the AI can predict the presence of species in unexplored or under-surveyed areas. For instance, by analyzing the historical migration patterns of bird species under changing climatic conditions, researchers can predict future shifts in migration routes. This predictive capability is crucial for preemptively identifying and protecting new critical habitats affected by climate change.
Another compelling study comes from researchers at Washington State University, who applied AI to develop habitat suitability models for the Canada lynx. By feeding vast amounts of data—including temperature variations, prey availability, and human activities—into their AI system, they were able to delineate areas most likely to support lynx populations. The insights derived from these models inform conservation strategies, such as pinpointing areas that require protective measures or identifying where new protected zones might be established.3
AI's ability to create detailed species distribution models aids conservationists in responding to emerging threats. For instance, when modeling the habitat of endangered tigers in India's Kanha-Pench corridor, AI predicted critical zones that required anti-poaching patrols, highlighting areas where human-tiger conflicts were most likely to occur. This proactive approach helps protect the tigers and mitigates risks to human communities living nearby—foreshadowing potential conflict areas and enabling timely intervention.
Further advancing this field, researchers have employed AI to understand the impacts of historical environmental factors on biodiversity. For instance, a study at the University of Birmingham leveraged AI to analyze genetic material from lake sediments, linking past biodiversity changes to specific environmental conditions like pollution and temperature fluctuations.4 This approach helps predict future biodiversity trends based on historical data, guiding long-term conservation planning.
AI-driven predictive models are also vital for planning translocation efforts. By simulating various scenarios, conservationists can predict the success of relocating species to new habitats. These simulations consider numerous variables, including habitat suitability, potential threats, and the presence of other species. This reduces the risks associated with such moves, increasing the chances of survival for endangered species.
Incorporating AI into conservation planning doesn't stop at predicting species locations and habitats; it also extends to understanding the potential impacts of human activities. For example, predictive models can anticipate how urban expansion, deforestation, or agricultural activities might encroach on natural habitats, allowing policymakers to devise strategies to mitigate these effects before they occur.
AI's role in species distribution and habitat suitability is expanding, bringing unprecedented precision to conservation efforts. Predictive modeling allows researchers to tackle future challenges head on, making conservation strategies more proactive rather than reactive. As AI technology continues to advance, so too will its applications in conservation, promising a better future for endangered species and the ecosystems they inhabit.
AI in Anti-Poaching and Threat Detection
AI's application in anti-poaching efforts and threat detection is revolutionizing the way conservationists can protect endangered species from illegal activities. Technologies like TrailGuard AI play a pivotal role in detecting and responding to poaching activities and other threats to wildlife.
TrailGuard AI, renowned for its recent recognition by TIME as one of the best inventions of 2023, is a prime example of how advanced AI can be deployed in conservation. This system integrates AI algorithms, advanced hardware, and versatile communication protocols to provide real-time alerts. It operates in more than 25 protected areas across Asia, Africa, and South America, monitoring critical habitats for any signs of poaching or human-wildlife conflict. TrailGuard AI's real-time alert system enables rapid responses, which is crucial in preventing poaching incidents before they escalate. For instance:
- New deployments in India's Kanha-Pench tiger conservation landscape have led to the arrest of poachers.
- The system has reduced human-tiger conflicts in the Dudhwa Tiger Reserve.
The success of these deployments underscores the potential of AI to transform wildlife protection strategies.
Another impactful case involves the Rainforest Connection, a San Francisco-based NGO using AI to combat illegal wildlife poaching in Africa. This organization has developed advanced systems that use acoustic sensors to detect the sounds of gunshots or chainsaws, which are often associated with poaching activities. The collected audio data is then processed by AI algorithms to confirm suspicious sounds. Upon detection of such sounds, alerts are sent to park rangers, enabling them to act swiftly and prevent illegal activities. This system has been effective in real-time threat detection, protecting species without direct human monitoring.
DeepMind, a UK-based AI company, has also contributed significantly to AI-powered wildlife protection by developing models that identify and count animals in protected areas like Tanzania's Serengeti National Park. These AI systems identify species, analyze population trends, and predict poaching hotspots. The data gathered allows conservationists to deploy anti-poaching patrols more effectively and preemptively address potential threats.5
AI technology isn't limited to ground detection. Drones equipped with AI capabilities offer a significant advantage in anti-poaching efforts. These aerial devices can patrol large areas and relay real-time footage back to rangers. AI algorithms analyze this footage to identify suspicious activities or unauthorized movements, triggering alerts that prompt immediate action. The speed and coverage of drones far surpass human patrols, making them a cost-effective solution for vast and remote conservation areas.
The Protection Assistant for Wildlife Security (PAWS) is another AI-based application demonstrating promising results. PAWS uses predictive analysis to optimize patrol routes for rangers based on data about poaching patterns, animal movement, and environmental conditions. This application integrates environmental and poaching data to predict where poachers are likely to strike, enabling rangers to focus their efforts in high-risk areas. This strategic approach has improved the efficacy of anti-poaching operations and reduced wildlife crimes in several protected parks.6
Conservation Metrics, a Santa Cruz-based company, aids wildlife monitoring through AI tools. Utilizing machine learning models, they analyze population trends and identify crucial areas threatened by poaching or habitat destruction. By mapping poaching incidents and correlating them with time and location data, Conservation Metrics helps rangers to optimize their patrol strategies, ultimately enhancing the protection of endangered species.
The integration of AI in anti-poaching efforts extends beyond immediate threat detection to the broader conservation landscape. By providing real-time, actionable data and predictive insights, AI-enabled systems empower conservationists to make informed decisions and implement effective strategies. As AI technologies continue to evolve, their role in wildlife protection will expand, offering new tools to combat poaching and preserve biodiversity.
The journey ahead involves refining these technologies to overcome current limitations, such as data quality and accessibility in remote areas. Investment in AI research and development, alongside collaboration among conservation organizations, will be crucial. The future of AI in anti-poaching is undeniably promising, offering hope for the sustainable coexistence of humans and wildlife.
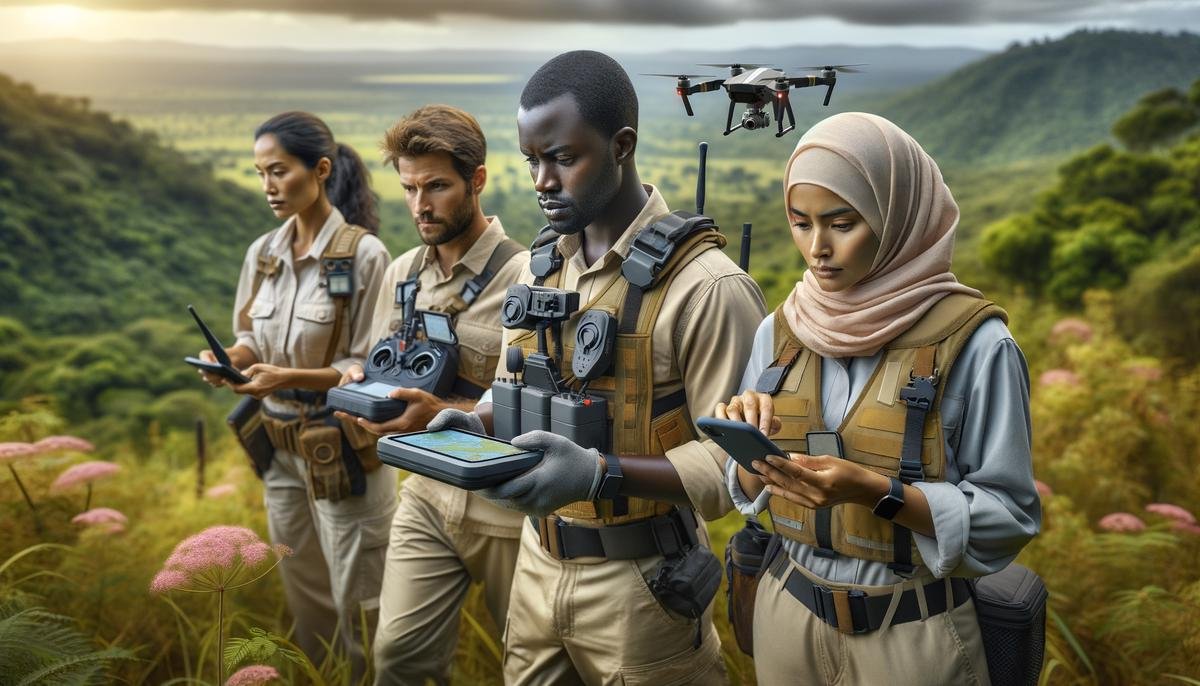
Environmental DNA and Biodiversity Analysis
AI plays a transformative role in analyzing environmental DNA (eDNA) and other biodiversity data, enabling scientists to gain deeper insights into species detection and biodiversity monitoring. AI-based methods leverage advanced computing techniques to process and interpret large datasets efficiently, offering significant benefits over traditional approaches.
Environmental DNA involves collecting genetic material left behind by organisms in their environments, such as water, soil, or air. AI enhances eDNA analysis by processing vast amounts of genetic data quickly and accurately. For instance, AI algorithms can sift through complex datasets to identify and match DNA sequences to specific species. This method is particularly valuable in monitoring elusive or rare species that are difficult to detect through conventional means.
One notable application of AI in eDNA analysis was demonstrated by researchers at the University of Birmingham. They developed an AI model to analyze genetic material from lake sediments to understand how past environmental conditions influenced biodiversity. By integrating climate data, pollution records, and genetic sequences, the AI identified correlations between environmental factors and biodiversity loss. This approach provided crucial insights into how historical changes affect current species distributions, informing future conservation efforts.
In tropical forests, AI's ability to analyze soundscapes has proven invaluable. Researchers like Jörg Müller at the University of Würzburg utilized AI to process audio recordings from Ecuador's Chocó region. By feeding these recordings into AI models, they could identify and quantify species based on their vocalizations. This method revealed how different stages of forest recovery influenced animal populations, providing a detailed understanding of biodiversity dynamics.
The application of AI in biodiversity analysis extends to the study of freshwater ecosystems. An example is a study published in eLife, where AI was used to analyze environmental DNA from lake sediment layers, linking genetic diversity changes to specific environmental stressors like insecticides and temperature fluctuations.1 By learning from historical data, AI models can predict future biodiversity trends and inform conservation strategies.
AI's predictive capabilities also play a crucial role in conservation planning. By modeling species distributions and habitat suitability, AI helps researchers identify critical habitats and prioritize conservation efforts. For example, Conservation AI's machine learning algorithms use historical data to forecast species presence in unexplored areas, guiding field surveys and resource allocation effectively. Such predictive models allow conservationists to anticipate and mitigate the impacts of climate change on biodiversity, ensuring that adaptive strategies are in place to protect vulnerable species.
In addition to these benefits, AI's integration in eDNA analysis can lead to more comprehensive biodiversity assessments. AI-enhanced eDNA techniques can detect elusive organisms, providing a fuller picture of ecosystem health. This holistic approach is essential for maintaining biodiversity and supporting sustainable ecosystem management.
The ongoing advancements in AI and eDNA analysis underscore the potential for continued innovation in biodiversity monitoring. By combining AI's computational power with cutting-edge genetic techniques, researchers can achieve unprecedented levels of precision and efficiency in their conservation efforts. As AI technology evolves, its applications in environmental monitoring will expand, offering new tools and methodologies to safeguard our planet's biodiversity.
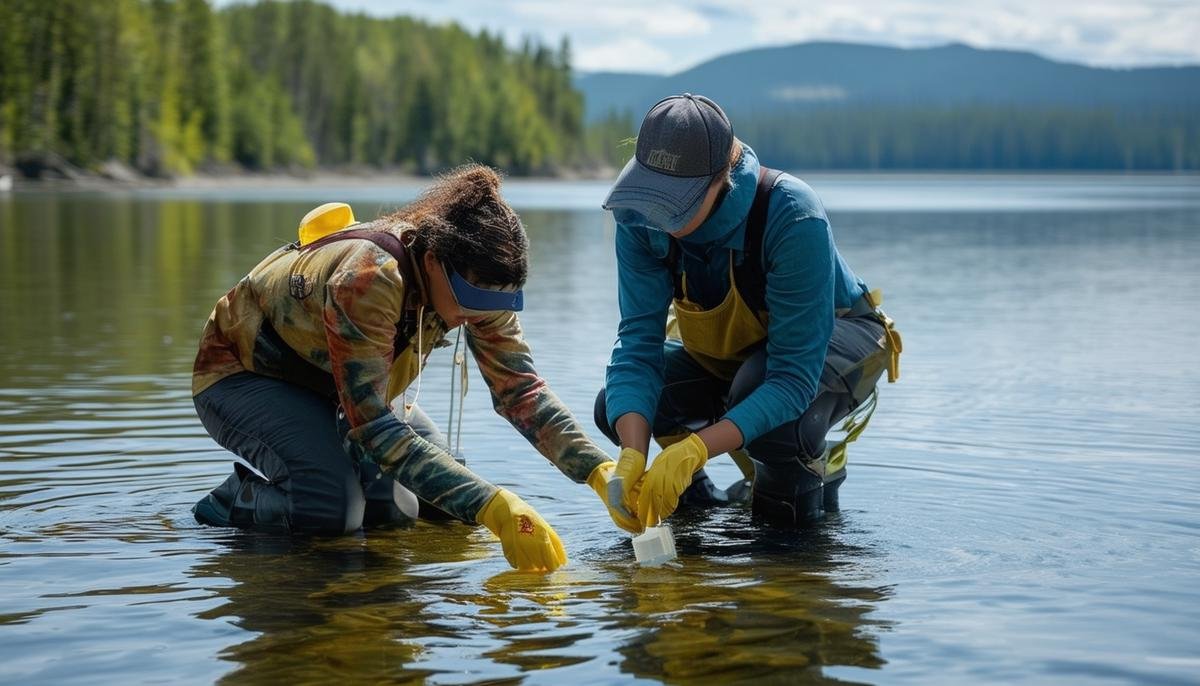
Challenges and Future Directions
Implementing AI in wildlife conservation comes with its set of challenges. One significant hurdle is the quality and availability of data. AI algorithms rely heavily on large, accurately labeled datasets to function optimally. However, collecting such data in remote or challenging environments can be difficult. Variables like inconsistent data collection methods, incomplete datasets, and the need for real-time updates pose substantial obstacles. The data often needs to be carefully annotated, which requires significant human effort and expertise.
Bias in AI models is another critical issue. If training data is skewed or lacks diversity, the resulting AI models may exhibit biases, leading to inaccurate predictions or overlooking certain species. Continuous monitoring, validation, and re-training of AI systems are essential to minimize these biases and maintain accuracy. This process, however, adds to the intricacy and resource requirements of implementing AI in conservation efforts.
Resource limitations also hinder the widespread use of AI in wildlife conservation. Many conservation organizations operate on limited budgets and may not have the financial means to invest in cutting-edge technology. Accessing the necessary computational resources, acquiring specialized hardware, and hiring skilled personnel can be prohibitively expensive. The deployment and maintenance of AI systems in remote areas require robust infrastructure and continuous technical support, further straining limited resources.
Ethical considerations are also paramount. The deployment of surveillance technologies to monitor wildlife must balance the benefits of data collection with the potential impacts on privacy and local communities. Involving local communities in the planning and implementation process can help mitigate these concerns and ensure that conservation efforts are sustainable and culturally sensitive.
Looking to the future, several advancements in AI technology hold promise for enhancing conservation efforts. One potential development is the improved integration of multimodal data sources. Combining data from various sources—such as satellite imagery, drone footage, acoustic recordings, and eDNA—can provide a more comprehensive understanding of ecosystems. Advances in machine learning algorithms capable of processing and integrating these diverse data types will enable more accurate and holistic monitoring and analysis.
Another promising direction is the development of more sophisticated and efficient AI models. Innovations in deep learning, such as the use of attention mechanisms and transformers, can enhance the capability of AI systems to handle complex and heterogeneous datasets. These advancements could improve species identification, trend analysis, and predictive modeling, leading to more effective conservation strategies.
Edge computing represents another frontier in AI technology. By processing data locally on devices near the data collection point, edge computing can reduce latency and dependence on centralized servers. This approach can be particularly beneficial in remote locations with limited internet connectivity, ensuring that AI applications remain functional and responsive even in challenging conditions.
AI can also benefit from advancements in collaborative platforms and data-sharing frameworks. Creating centralized repositories and cloud-based platforms for conservation data will facilitate collaboration among researchers and organizations. AI-powered data management tools can automate data curation, annotation, and sharing, promoting transparency and consistency across different datasets. Enhanced collaboration can lead to faster innovations and more robust conservation strategies.
Developing AI models that incorporate adaptive learning mechanisms can help mitigate the challenges of dynamic and evolving environments. These models can continuously learn from new data, adapting to changes in environmental conditions, species behaviors, and human impacts. This adaptability will be crucial for maintaining the relevance and accuracy of AI systems in long-term conservation efforts.
Investing in capacity building and education is also vital. Training conservationists, ecologists, and local communities in using AI tools and interpreting their outputs will empower them to leverage technology effectively. Educational initiatives and partnerships with academic institutions can help bridge the knowledge gap and ensure that AI-driven conservation is accessible and inclusive.
The future of AI in wildlife conservation holds tremendous potential, with ongoing research and technological advancements paving the way for more sophisticated and effective applications. By addressing current challenges and focusing on innovation, collaboration, and education, AI can become an indispensable tool in safeguarding our planet's biodiversity and ensuring a sustainable future for all species.
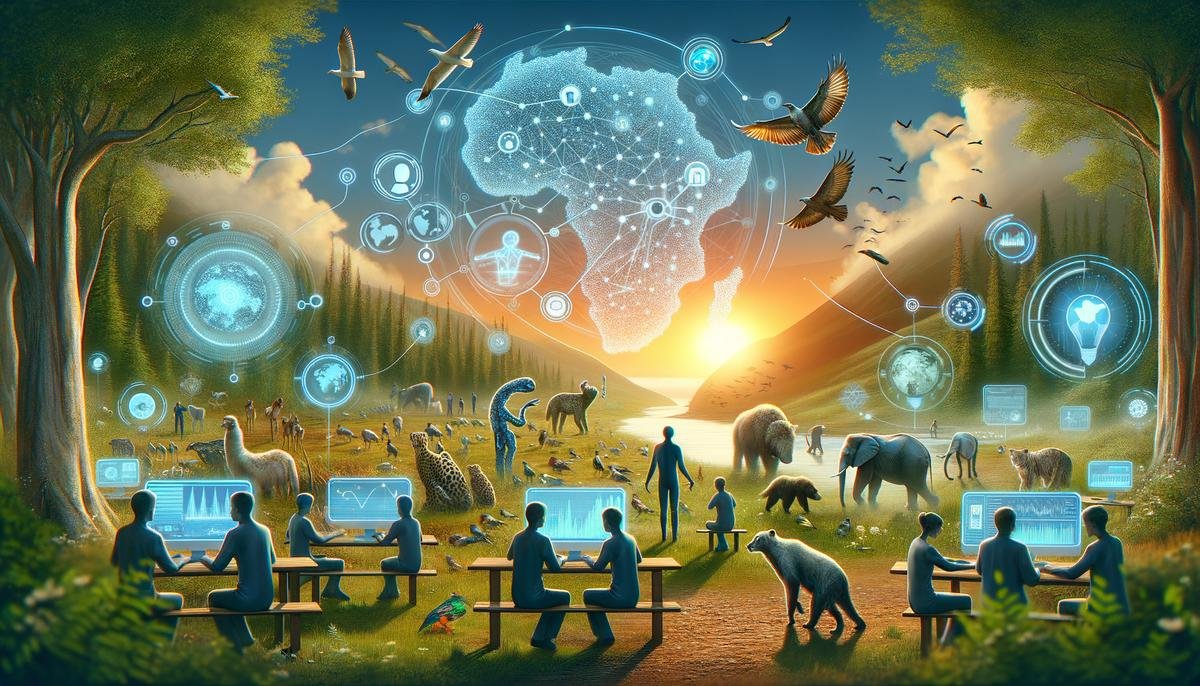
AI's role in wildlife conservation is expanding, offering promising advancements in protecting endangered species and their habitats. By leveraging technology to interpret and react swiftly to data, AI is becoming an essential tool in the fight to preserve our planet's biodiversity.