AI’s Role in Translational Research and Clinical Trials
AI has revolutionized the analysis of large datasets in translational research. It handles the complexity of data from pathology, radiology, molecular science, and clinical wearables with ease, finding new biomarkers and unraveling disease pathways in ways previously unimaginable.
In evaluating solid tumors, AI quantifies biomarkers with precision, revealing spatial intricacies of the microenvironment that provide insights undetectable by the human eye. This approach could lead to the discovery of new treatment targets and more precise biomarkers customized for individual patients.
In clinical trials, AI helps select patient populations most likely to benefit from therapies. It carefully measures patient responses, which is critical in trials for conditions like metabolic dysfunction-associated steatohepatitis (MASH). For MASH, AI replaces subjective manual scoring with reproducible results on continuous scales.
In molecular trials for immunotherapies, AI evaluates overall survival and potential toxicities like pneumonitis, hepatitis, and colitis with precision. By assessing routine clinical data, AI curates predictions with an accuracy rate of 75% to 80%.1
However, data quality and biases are major concerns. Pathologists face difficulties integrating varied data formats and incomplete datasets. Addressing biases, especially in training data, is crucial to avoid misapplying predictions to underrepresented groups.
Generative AI, like ChatGPT, shows potential for treatment suggestions but requires stringent validation due to its proclivity for errors. Understanding why an AI reaches certain conclusions remains critical for clinicians trusting these tools in decision-making.
AI’s contributions are transformative, giving researchers the ability to comprehensively analyze multifaceted data sets. The alliance of AI precision and human expertise promises a healthcare future with quicker diagnoses, more customized treatments, and profoundly improved lives.
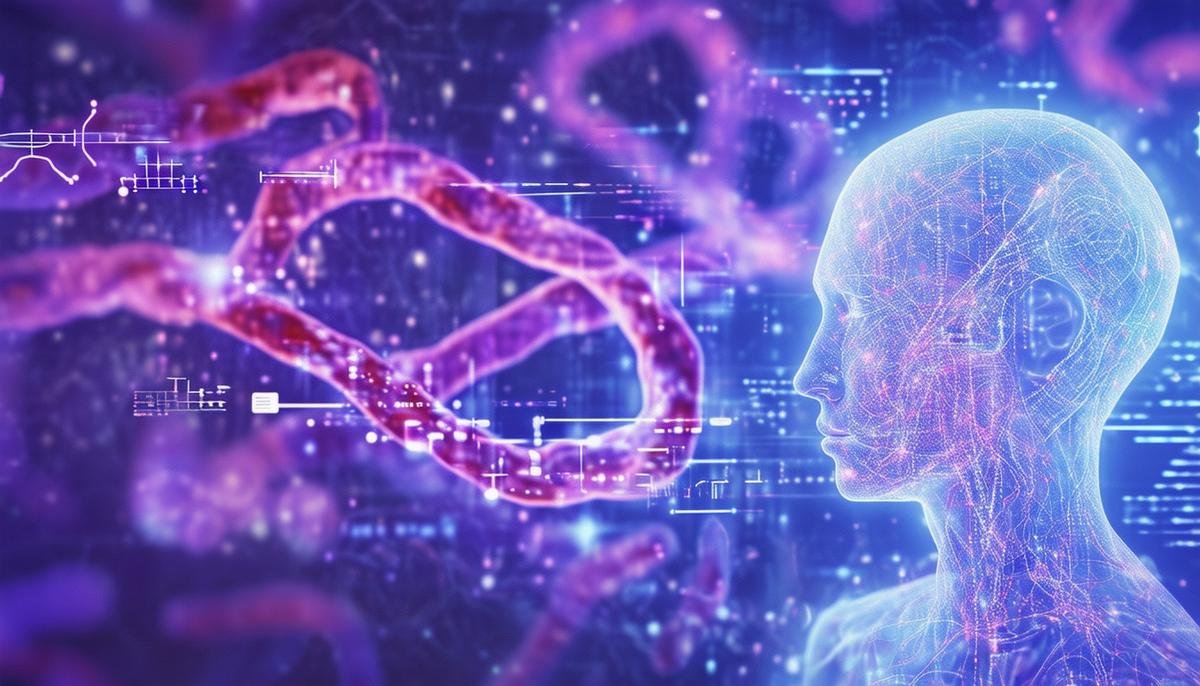
Impact on Clinician Well-Being and Workflow Efficiency
Digital pathology and AI-powered workflows streamline many aspects of a physician’s routine, eliminating inefficiencies. Digitally scanned slides are instantly available for evaluation, speeding up diagnostic processes and enabling higher accuracy and repeatability compared to manual methods.
AI flags significant findings, such as the presence of tumors, allowing pathologists to focus their expertise on more intricate cases. This automation enhances the reliability of diagnoses, like counting nuclei in glioblastoma.
Cloud infrastructure enables seamless cross-border collaborations, allowing pathologists to consult with peers worldwide in real-time, fostering a more cohesive global medical community.
AI’s potential to alleviate physician burnout is significant. As physicians offload repetitive tasks to AI systems, they gain time for patient care and other critical duties, reducing stress and burnout risk. This transformation makes the profession more attractive to new trainees.
AI-driven workflows introduce flexibility, enabling remote work and better work-life balance for pathologists. This appeals to existing professionals and upcoming generations seeking a modern, adaptable career path.
By handling time-consuming tasks, AI allows pathologists to focus on applying their expertise and judgment to make critical decisions. This synergy of human skill and AI efficiency promises to redefine the future of healthcare diagnostics, creating a more sustainable, rewarding, and effective healthcare system.
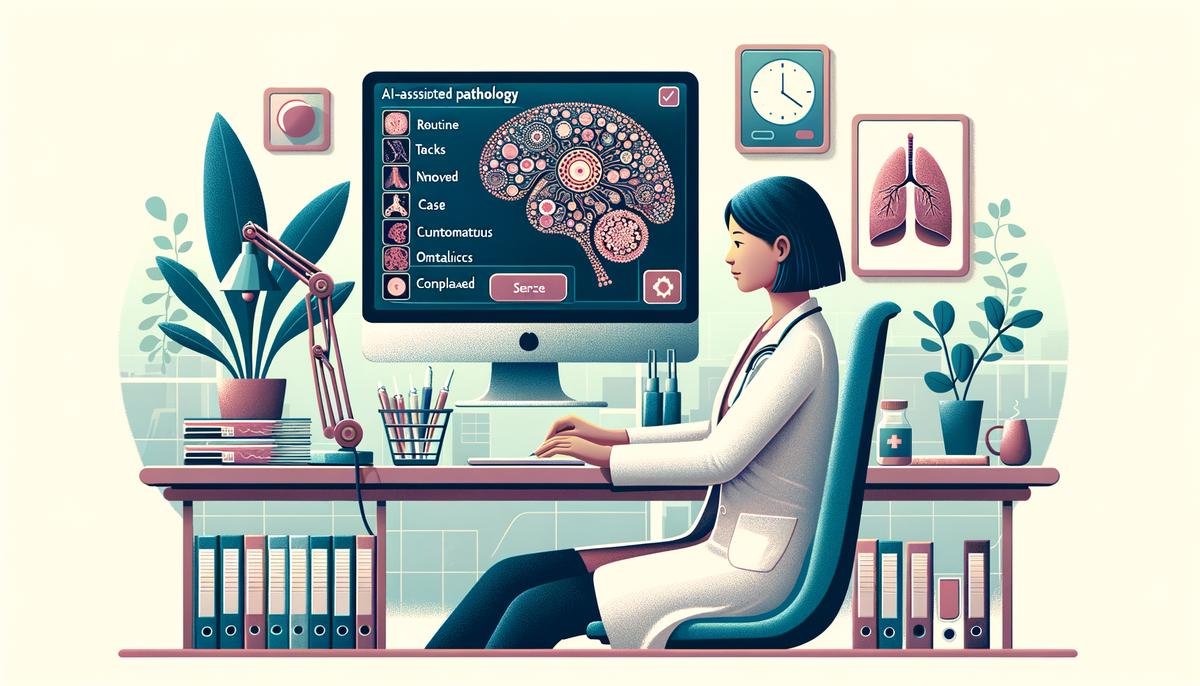
Economic and Cost Benefits of AI in Pathology
Transitioning to digital pathology and AI-enabled systems requires significant initial investment in infrastructure, which can be challenging for smaller labs. However, the potential for return on investment (ROI) is promising.
For individual laboratories, adopting these technologies can result in immediate productivity improvements. Digital pathology, supported by AI, drastically reduces workload by automating processes, allowing pathologists to analyze slides more swiftly and with higher precision.
Efficiency gains translate into cost savings. With AI handling repetitive tasks, pathologists can focus on more intricate diagnostic tasks, speeding up the process and reducing costly errors. The reduction in manual labor hours and enhanced reliability contribute to a laboratory’s operational efficiency.
At the health system level, large-scale adoption of AI and digital pathology can lead to systemic enhancements in workflow and productivity. Standardizing diagnostic processes facilitates smoother operations and reduces inter-laboratory variability, ensuring consistent diagnostic accuracy and patient care network-wide.
- Digital pathology streamlines the management of pathology services.
- Centralized digital storage and easy retrieval of images enhance collaboration among specialists, enabling quick sharing and discussion of cases, leading to faster diagnosis and treatment, which can improve patient outcomes and reduce hospital stays.
- AI-driven pathology can enhance a health system’s competitiveness, attracting top talent and increasing participation in advanced clinical trials, bolstering reputation and opening avenues for additional funding and investment opportunities.
AI’s ability to analyze vast datasets and identify patterns contributes to more effective patient stratification in clinical trials, improving trial outcomes, securing faster drug approvals, and bringing new therapies to market earlier. This enhances clinical trial capabilities and partnerships with biopharma companies, driving economic growth and innovation.
Partnerships, pilot programs, and phased rollouts can help labs gradually adapt to new technologies, allowing for continuous evaluation and improvement without overwhelming financial pressures.
While the initial adoption of AI and digital pathology requires noteworthy investment, the long-term economic benefits outweigh the costs. Improved productivity, enhanced diagnostic accuracy, and operational efficiencies lead to significant cost savings, providing substantial ROI at both individual laboratory and health system levels.
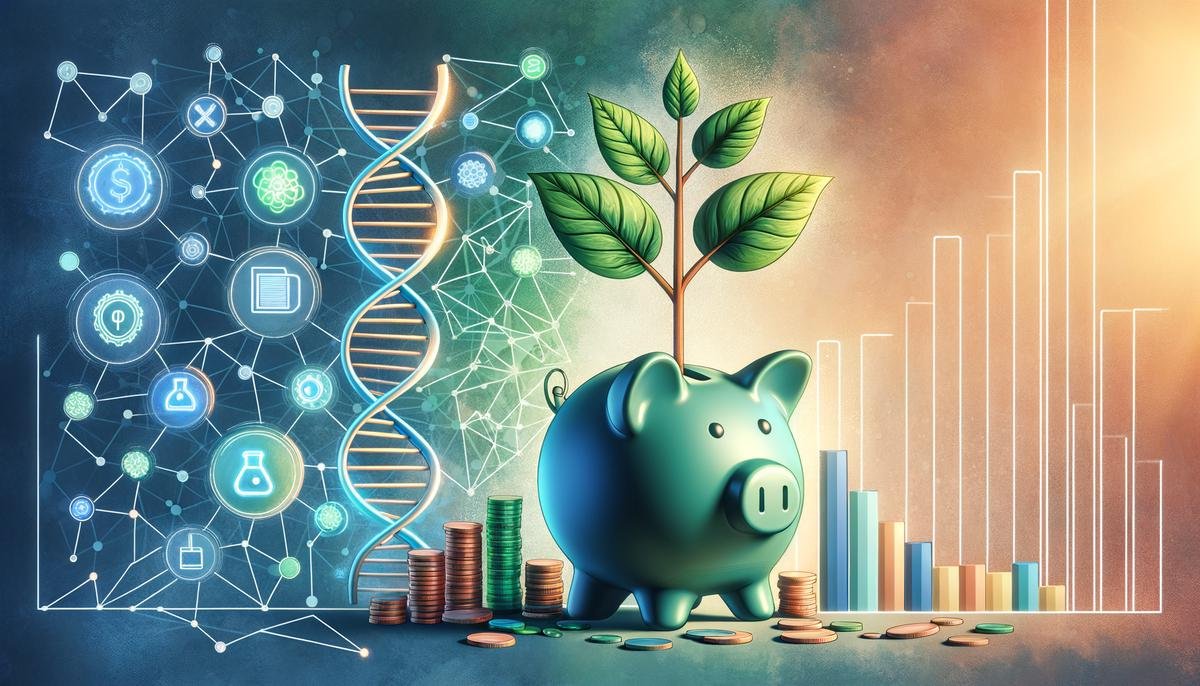
Enhancing Patient Experience and Health Equity
AI-powered pathology enhances the patient experience by providing unprecedented speed and precision in diagnostic processes. Through automated analysis, digital pathology can deliver results much faster, facilitating quicker decisions on treatment plans. This rapid turnaround is crucial for conditions where early intervention can dramatically improve outcomes.
The precision of AI-enabled diagnostics means patients receive information they can trust. AI algorithms can detect minute details and patterns that may be overlooked by the naked eye, ensuring faster and more accurate diagnoses, leading to more effective treatment strategies. This reduces anxiety and uncertainty for patients, knowing they are less likely to suffer from diagnostic errors.
AI-assisted pathology reports enable physicians to better explain diagnoses and treatment options, fostering a more informed and engaged patient. Patients who understand their condition and the rationale behind their treatment are more likely to adhere to prescribed therapies, improving their overall prognosis.
In terms of health equity, AI-powered pathology stands as a pivotal force. By training algorithms on data from varied demographics, AI can produce more generalizable and equitable diagnostic tools, avoiding biases that might lead to misdiagnoses in underrepresented groups.
Distributing digital pathology infrastructure to underserved areas can address health disparities. Remote areas can access advanced diagnostic services through telepathology, where digital slides are sent to centralized hubs equipped with AI tools. These hubs can provide rapid and accurate assessments, ensuring that patients, regardless of location, receive the same quality of care.
Pathologists in lesser-resourced settings can collaborate with and learn from experts worldwide through cloud-based digital platforms, ensuring access to global knowledge repositories.
Federated learning allows AI models to be trained on data from multiple sources without direct data sharing, preserving patient privacy while enhancing the model’s reliability and applicability.2 This enables healthcare providers across different regions and institutions to contribute to and benefit from AI without the risks associated with data breaches or privacy violations.
Integrating AI into pathology is not just a technological evolution but a step towards a more equitable healthcare system. It ensures that the benefits of advanced diagnostics and personalized treatment plans are accessible to a diverse and widespread patient population, paving the way for better health outcomes across the board.
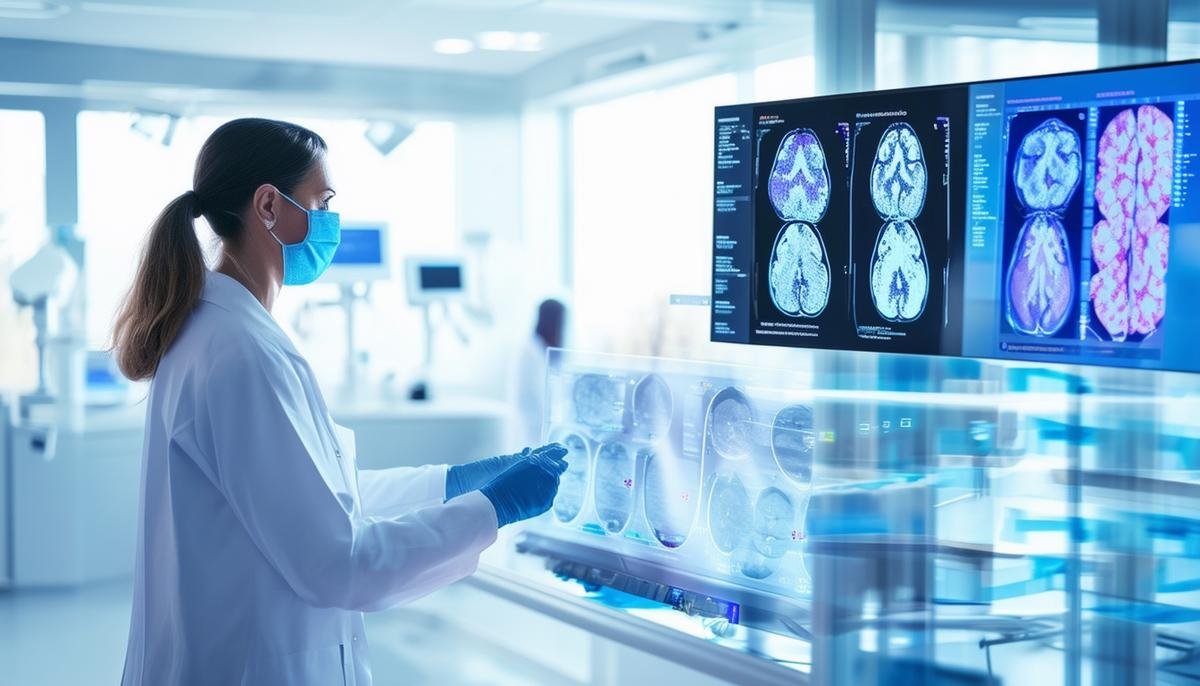
Challenges and Future Directions
Despite the promise of AI in healthcare, skepticism among providers remains a hurdle. This skepticism stems from limitations and missteps in AI’s application within the clinical setting. Concerns around bias, data quality, and generalizability are at the forefront, influencing cautious adoption.
Bias in AI often originates from the training datasets used to develop the algorithms. If these datasets do not adequately represent diverse patient demographics, the resulting models may demonstrate biased performance. For instance, an algorithm trained predominantly on data from a specific ethnic group may not accurately diagnose conditions in individuals from other ethnicities, leading to disparities. Proactive measures in AI model development are necessary to ensure inclusivity and fairness.
Data quality plays a crucial role in the effectiveness of AI models. Datasets must be comprehensive, accurate, and up-to-date to train reliable algorithms. However, real-world clinical data often suffers from inconsistencies, missing values, and varying formats, posing challenges for AI applications. Data curation—cleaning, standardizing, and harmonizing—is critical yet arduous, demanding thorough attention to detail.
The generalizability of AI models remains a pressing concern. While a model may perform well on the data it was trained on, its utility in broader, real-world scenarios often remains untested. Ensuring that these models maintain accuracy and reliability across diverse patient populations and clinical settings is paramount. Without rigorous external validation, there is a risk that AI tools may fail when applied to new datasets, undermining clinicians’ trust.
Generative AI brings an additional layer of complexity and promise. Unlike traditional AI models that rely on structured data, generative AI can process unstructured information, including text and images. Tools like ChatGPT showcase the potential for generating insights from complex clinical data. However, the propensity of generative AI to produce “hallucinations”—false or misleading information—introduces a significant risk, particularly in patient care where accuracy is non-negotiable. This underscores the need for rigorous validation and containment mechanisms.
Transparency and explainability are pivotal in addressing the trust deficit in AI. Healthcare providers need to understand how AI models derive their conclusions to trust and utilize these tools effectively. Explainable AI (XAI) seeks to provide insights into the decision-making processes of AI models, highlighting the underlying rationale. This transparency is crucial for clinicians who remain the primary decision-makers in patient care.
The future of AI in healthcare must prioritize ethical considerations, regulatory frameworks, and collaboration across disciplines. Regulators and industry leaders must work together to develop standards and guidelines that ensure responsible deployment. These measures will help mitigate risks and foster a trustworthy environment for integrating AI into clinical practice.
Addressing the challenges of bias, data quality, and generalizability is imperative. The advent of generative AI offers exciting possibilities, yet demands oversight to prevent errors. Transparency and explainability in AI models are essential to forging trust with healthcare providers. Through deliberate efforts and ongoing innovation, the integration of AI into healthcare can enhance patient care and advance the future of medicine.
Key Challenges in AI for Healthcare:
- Bias in training datasets
- Data quality and inconsistencies
- Generalizability to real-world scenarios
- Risk of “hallucinations” in generative AI
- Lack of transparency and explainability
AI’s integration into healthcare diagnostics is a step towards more accurate, efficient, and equitable patient care. By combining AI’s precision with human expertise, we can look forward to a future where diagnoses are faster, treatments are more personalized, and healthcare outcomes are improved. The synergy between artificial intelligence and human intelligence holds the key to revolutionizing healthcare delivery and improving the lives of patients worldwide.1
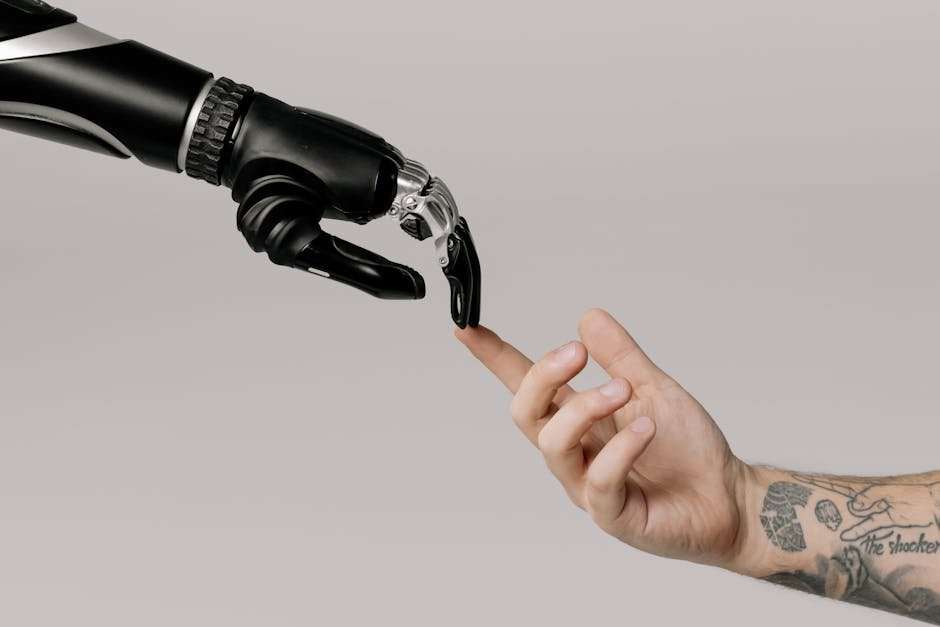