The realm of language models has become exceedingly complex and dynamic, heralded by transformative innovations like AutoGPT. AutoGPT, standing as an automated version of the Generative Pre-training Transformer, has pushed the frontiers of computational language models by offering capabilities that span various language-related tasks. While still a nascent technology, the magnitude of its impact belies its age, reshaping our interactions with synthetic language and the manner in which we understand and harness machine learning. Gaining a keen understanding of its functionality, performance, comparative standing, applications, limitations, and an insightful look into the future prospects can greatly enhance our perspective on this ground-breaking technology.
Understanding AutoGPT
In seeking to demystify the vast world of machine learning, it is essential to delve into one of its most fascinating developments: AutoGPT, or Autoregressive Generative Pretraining and Transformer. AutoGPT, while just a speck in the cosmos of machine learning developments, holds an arena of infinite possibilities, enabling us to fathom advancements in artificial intelligence that were once only a figment of scientific conjecture.
At the heart of AutoGPT lies the foundational principle of autoregression, a statistical model that measures how crucial variables change over time based on their own past values. With this insight on board, we may think of AutoGPT as a time traveller – it harnesses previous knowledge to generate accurate predictions of yet-to-be-seen future events.
The masterpiece orchestrating these processes is the Transformer, an architectural model designed in the shadows of Recurrent Neural Networks (RNNs) and Convolutional Neural Networks (CNNs). Its design is suited to handle sequential data, the type of data that is ordered or formed in a sequence, while avoiding some of the drawbacks of its predecessors. In essence, Transformers provide AutoGPT with the necessary “sight” to both comprehend past contexts and construct plausible future predictions.
AutoGPT’s power, however, doesn’t stop with prediction; its true prowess lies in discussing its favorite topic – text. Harnessing the power of autoregression and transformers, AutoGPT has advanced the realm of Natural Language Processing (NLP). It understands, generates, translates, and summarizes human languages with increasing fidelity – often rivaling its human counterparts. It doesn’t just mimic human language; it dives into the depths of syntax, semantics and even sentiment, delivering outputs that echo human-like comprehension.
Imagine a book lover who encapsulates a vast library in their mind. AutoGPT is an equivalent. Through Generative Pretraining, it engulfs large volumes of text data, familiarizing itself with the innumerable dance steps of words and phrases across different contexts. To simplify, think of Generative Pretraining as AutoGPT’s intensely comprehensive preparatory reading session before it takes its text-generation exam.
One might wonder, why all this effort for text? The answer lies in the digital age’s lifeline: Data. The overwhelming portion of our digital data landscape consists of unstructured text – a treasure trove of information waiting to be deciphered. AutoGPT is our Rosetta Stone, translating this cryptic world of text into comprehensible, usable insights to elevate our understanding, decision making and open doors to new possibilities.
In conclusion, AutoGPT is not just another product of machine learning. It is a champion of the symbiosis between machines and human language, reshaping our expectations from technology. It’s an epitome of evolutional synchrony between human thought processes and artificial intelligence. Yet, regardless of its triumphs in text analysis and generation, the beauty of AutoGPT, much like the realms it seeks to decode, remains in its constant evolution and the promise of unexplored territories yet to be unveiled.
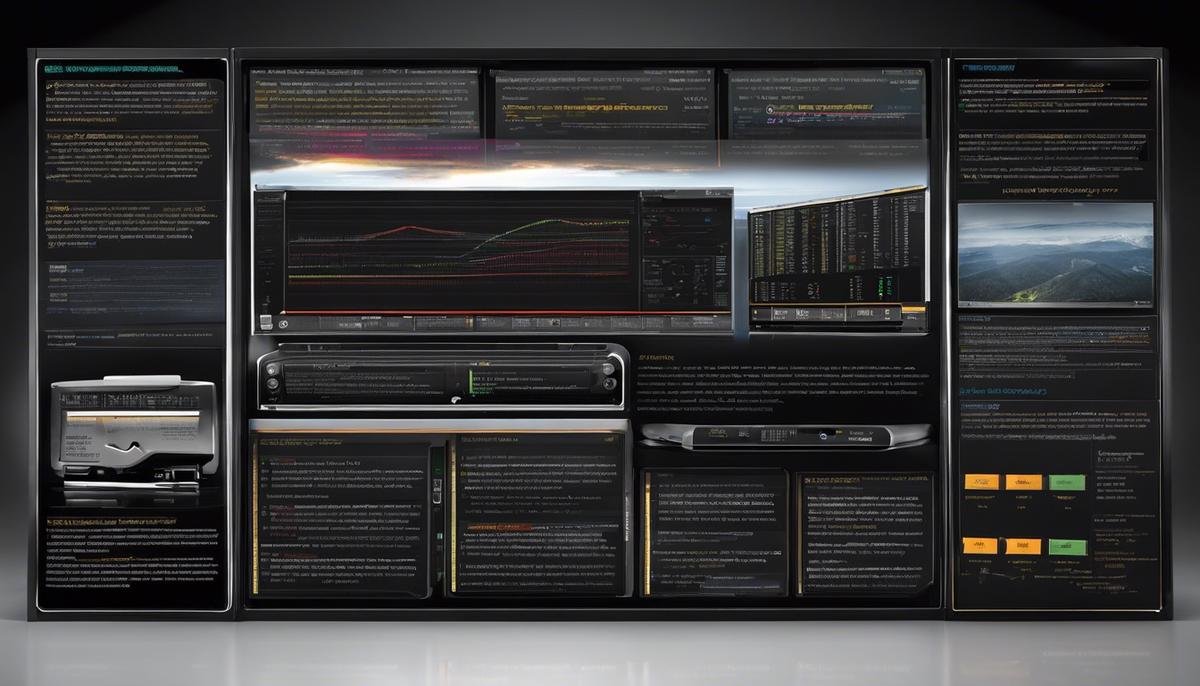
Breaking Down AutoGPT Performance
Building upon the fundamentals of autoregression and the transformative capabilities of the Transformer model, AutoGPT (Automatic Generative Pre-training Transformer) demonstrates an astonishing precision in various aspects of machine learning and Natural Language Processing (NLP). With an intensive focus on key performance aspects, it advances the potentials of text generation, making a more substantial contribution to the future of digital communication.
One crucial feature that enhances the prowess of AutoGPT pertains to topicality. It excels at generating organic, coherent and topic-specific lines of thought within its output. Its robust structure ensures a consistent narrative, an essential aspect for applications ranging from drafting emails to creating code, song lyrics, poetry or prosaic writing. Thus, AutoGPT boasts impressive versatility, helping machines understand and simulate the nature of human dialogue and communication.
The model’s robustness also plays a critical role in understanding and reflecting nuances of tone and style in generated text. AutoGPT‘s ability to adapt and replicate a specific set of linguistic prompts to carry communication in a particular style is commendable. This ability pivots the expansion of automated communication strategies in various sectors including class-rooms, customer relationship management, and newsrooms amongst others.
Further, the zero-shot and few-shot learning capabilities of AutoGPT deserve special attention. The model demonstrates an impressive comprehension of instructions and the capacity to generalize from limited examples. This critically reduces data dependency, improving performance, and saving resources. Applications are far-reaching including aiding in language translation, summarizing complex text, and generating new, unseen linguistic patterns.
Moreover, AutoGPT‘s efficient scalability sets it apart. Larger models invariably outrank the smaller versions. This association between size and result quality underlines the immense potential of AutoGPT, fueling continued research and development toward more elaborate models.
However, the image-to-text generation capability is an area where AutoGPT proves to be a game-changing technology. Unlocking the potential to translate pixels into phrases, it imbues machines with the capability to describe visual contents, a significant advancement in machine perception. This breakthrough could revolutionize fields like diagnostics, security, and digital content creation.
Furthermore, AutoGPT demonstrates proficiency in blending explicit programming concepts with machine learning, thus making strides in the realm of Programmable Artificial Intelligence. This convergence is pivotal in establishing a clearer, more efficient communication between humans and AI.
Despite the astounding capabilities, it is essential to acknowledge the inherent risks and challenges concerning biases in text generation, data security, and ethical considerations. As AutoGPT continues to evolve, these crucial factors co-evolve, demanding constant vigilance and fine-tuning to ensure that technology serves as an ally to humanity and not a formidable force.
In conclusion, the key performance areas associated with AutoGPT extend beyond text-generation, proving instrumental in enhancing machine learning architecture’s efficiency, versatility, and interpretability. As this remarkable technology burgeons, one can only remain wonderstruck at the impending intersection where human language and digital language will seamlessly converge.
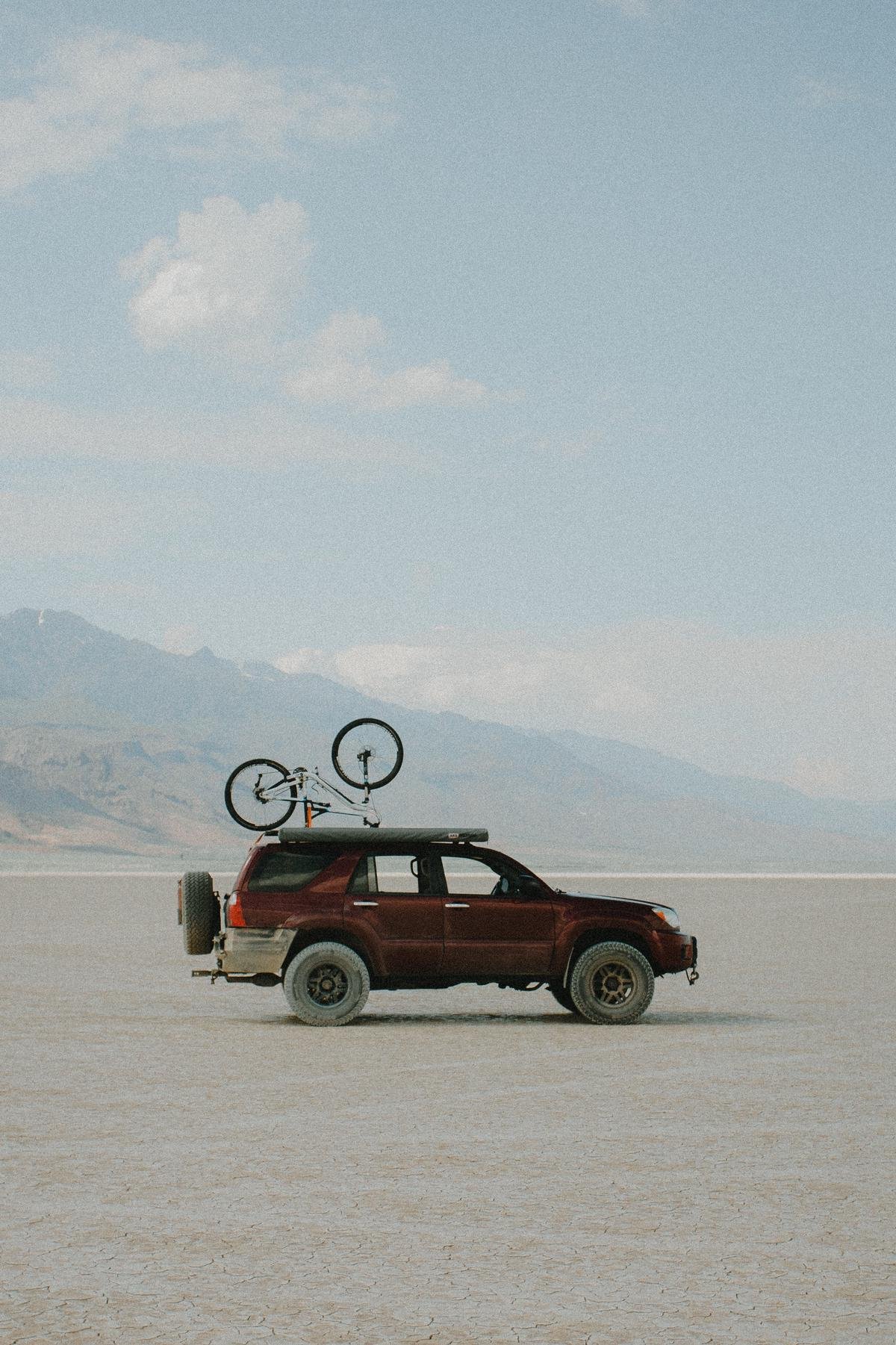
Photo by hananedwards on Unsplash
Comparative Analysis of AutoGPT
Now that an understanding of the methodology and foundational elements of AutoGPT has been established, it’s pivotal to explore its performance through direct comparison with other similar models. Amongst the pantheon of sharp machine-learning tools, AutoGPT has demonstrated noteworthy strides, demonstrating a level of accuracy and efficiency when faced with language data manipulation.
Performance in machine learning speaks to precision: How accurately can a model predict or generate the desired output? For AutoGPT, accuracy in Natural Language Processing (NLP) shines brightly. The model has presented an impressive capability to generate coherent, topic-specific output, even when dealing with convoluted subjects, showing an edge over other NLP models.
Another potent aspect in the evaluation of AutoGPT’s performance is its understanding and reflection of the nuances of tone and style within generated texts. Often overlooked, the ability to capture these subtleties mimics the precision of human intelligence, matching, and in some instances surpassing rival models.
Transformative in the field of machine learning is the concept of zero-shot and few-shot learning capabilities. AutoGPT has ventured into this territory, demonstrating its ability to make accurate inferences from unseen data or with minimal examples. Consequentially, it outstrips other counterparts reliant on massive training data to accomplish similar tasks.
Efficient scalability, particularly as it relates to the size of the model and resulting output quality, is another pivotal performance measure. AutoGPT offers a substantive argument for how its performance scales with size. Large-scale models often exhibit limited quality improvement past a certain threshold. However, AutoGPT has successfully managed to circumvent this, showing consistent improvements in the quality of results as the model size increases.
On the front of image-to-text generation capabilities, AutoGPT has demonstrated an uncanny ability for machine perception of visual data, besting other models with its efficiency and accuracy in converting complex image data into meaningful text.
An unusual, yet salient point of comparison is AutoGPT’s adroitness at blending programming concepts with machine learning. Seemingly disparate fields converge within AutoGPT, which distinguishes it from other models, thereby increasing its range of applications.
However, all these advancements are not without their challenges and potential risks. As with any technology, ethical considerations loom large in the utilisation of AutoGPT. Mitigation of biased language generation and protecting misuse of the technology are all challenges that must be squarely addressed.
In conclusion, AutoGPT exhibits an impressive performance portfolio amongst other similar models. With unparalleled coherency in output, extraordinary nuance detection, remarkable efficiency in zero-shot learning, superior scalability, and an unusual capacity for programming-meets-AI, AutoGPT heralds the impending convergence of human language and the digital lexicon. As it stands, the formidable performance of AutoGPT propels its position to the forefront of machine learning models. This only paves an exciting runway for the future, into a world where digital language becomes increasingly indistinguishable from human language.
Applications and Limitations of AutoGPT
AutoGPT and its prowess in precision with machine learning and Natural Language Processing (NLP) have begun to pave the way for transforming the interplay between human language and technology. It goes beyond just a simplistic understanding of words or phrases, but delves into insightful interpretations of textual language, making this technology crucial to our cyber-physical future.
Its precision is further accentuated through its capability in generating coherent and topic-specific outputs. Unlike traditional models, AutoGPT exemplifies finesse, accurately deciphering human context, while ensuring that the generation of the text remains relevant and focused. Beyond the boundaries of just translating and summarizing, its application now spans to developing structured narratives which hold the potential to revolutionize sectors such as content creation and journalism.
Moreover, the preciseness of AutoGPT enables it to understand and reflect subtle nuances of tone and style. So, not only is it able to generate texts, but it can also echo particular stylistic properties tailored to a particular audience or genre. This trait has tremendous implications for fields such as marketing and communications, which require a specific tonal premise.
In the realm of learning capabilities, AutoGPT showcases promise through its prowess in zero-shot and few-shot learning capabilities. This implies that the model can efficiently understand tasks related to language without requiring explicit prior examples or guidance. Such capabilities hold profound implications for facilitating a personalized and seamless user experience ranging from personalized virtual assistants to adept chatbots.
When delving into scalability, the relationship between the size and result quality becomes apparent with AutoGPT. As the model size scales up, the qualitative superiority of its outputs becomes evident. Such efficient scalability paves the way forward for commercial viability and large-scale usability.
Deciphering visual inputs into textual aspects is another breakthrough area for AutoGPT, giving it image-to-text generation capabilities. This feature helps widen the ambit of AutoGPT applications, transcending language bounds and introducing machine perception, underscoring the promising potential in sectors like assisted living technology and surveillance.
The intersections of programming concepts and machine learning have been effectively blended, where AutoGPT’s prowess lies not only in understanding but in the execution of programming-related tasks. Such advances suggest potential future applications in software development, programming tutoring, and automated debugging.
Nevertheless, embracing such transformative technology comes with its share of risks, challenges, and ethical considerations. Threading on the cautious side, these limitations encompass data privacy concerns, the potential misuse of generated content, ethical issues in unbiased learning, and the need for regulation in automated content.
In sum, AutoGPT is holding the influential torch as a pioneer, signifying the impending convergence of human language and the digital realm. Despite the challenges, its potential remains indisputable, helping clear the brink for an exciting erudition of linguistics, technology, and their resonating interplay.
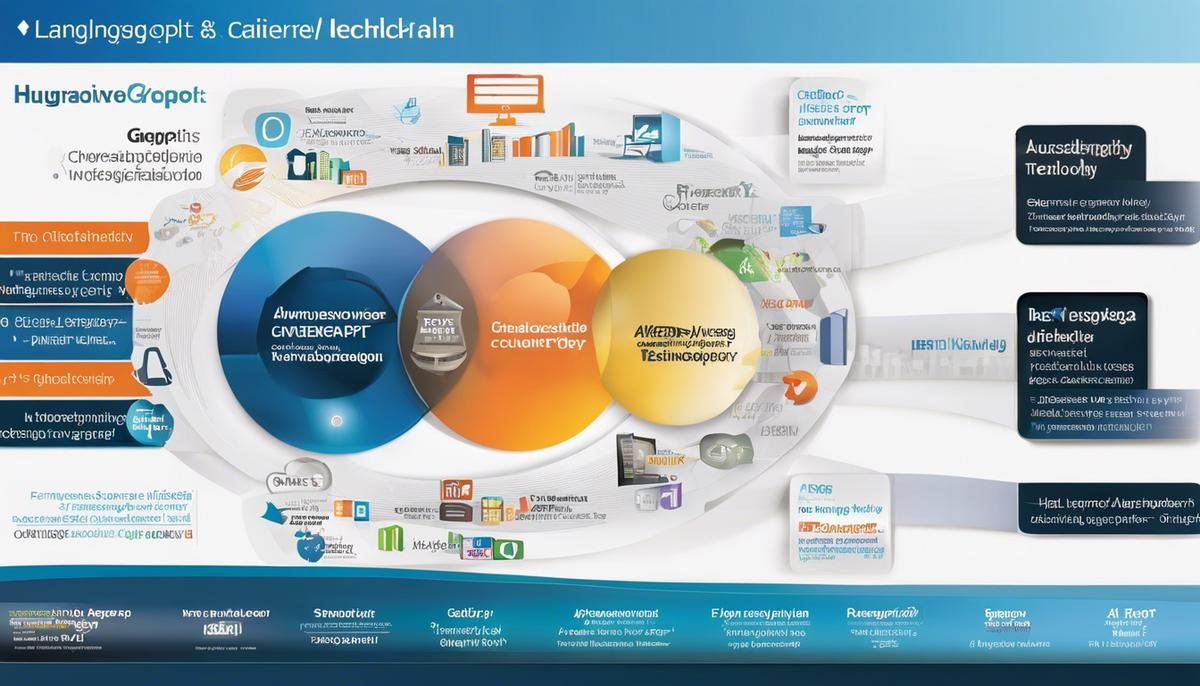
Future Directions for AutoGPT
Looking ahead, there remains an intriguing landscape of research opportunities and developments that could further augment the power and utility of AutoGPT. The current precision of models in the realm of machine learning and Natural Language Processing (NLP) is remarkable. However, as scientists often do, we look for improvements. Achieving higher accuracy rates with AutoGPT can open up new vistas for the future of not just text generation, but every mode of human-computer interaction.
The ability of the model to generate coherent and topic-specific outputs is another area ripe for exploration. While AutoGPT holds the key to translating the complexity of human languages, the challenge remains to make its output more tailored to the intricacy of individual topics. This can lead to more precise responses that take into account the specific requirements and nature of a topic, thereby fine-tuning the model’s performance.
The phenomenon of AutoGPT reflecting subtle nuances of tone and style in the generated texts is an exciting prospect. Currently, style and tone adjustments in machine learning models are rather generic and less adaptable. If future research concentrates on teaching AutoGPT about the difference in communication styles across varied contexts, it can lead to a quantum leap in its natural language generation capabilities.
AutoGPT’s ability in harnessing zero-shot and few-shot learning, which hold the promise of enabling the model to produce reliable results while trained on a minor fraction of examples, represents a new frontier in machine learning. This could effectively break the current dependency on vast troves of training data, a development that has massive implications for efficiency and scalability of machine learning models.
Moving towards the intersection of images and text opens up another realm of possibilities. By enhancing AutoGPT’s image-to-text generation capabilities, it could mark a significant advance in the field of machine perception, encompassing areas such as visual captioning and visual question answering.
The confluence of programming concepts and machine learning also outlines a promising, albeit challenging path. This blending can be the genesis of a new generation of NLP tools, placing an unprecedented level of control and potential in the hands of developers.
Amidst all the promise and potential, one must not overlook the implications and the necessity for ethical considerations in the AI realm. The inherent risks and challenges, such as misinformation and language biases, need proper mitigation strategies to prevent misuse. Continued research and development in these areas can navigate the path to the responsible use of this transformative technology.
One can envisage a future where a system like AutoGPT weaves its way into mainstream digital narrative, bridging the divide between the organic complexity of human language and the binary simplicity of digital language. While this future poses significant challenges, it equally presents immense possibilities to help shape the next generation of communication and interaction across digital landscapes.
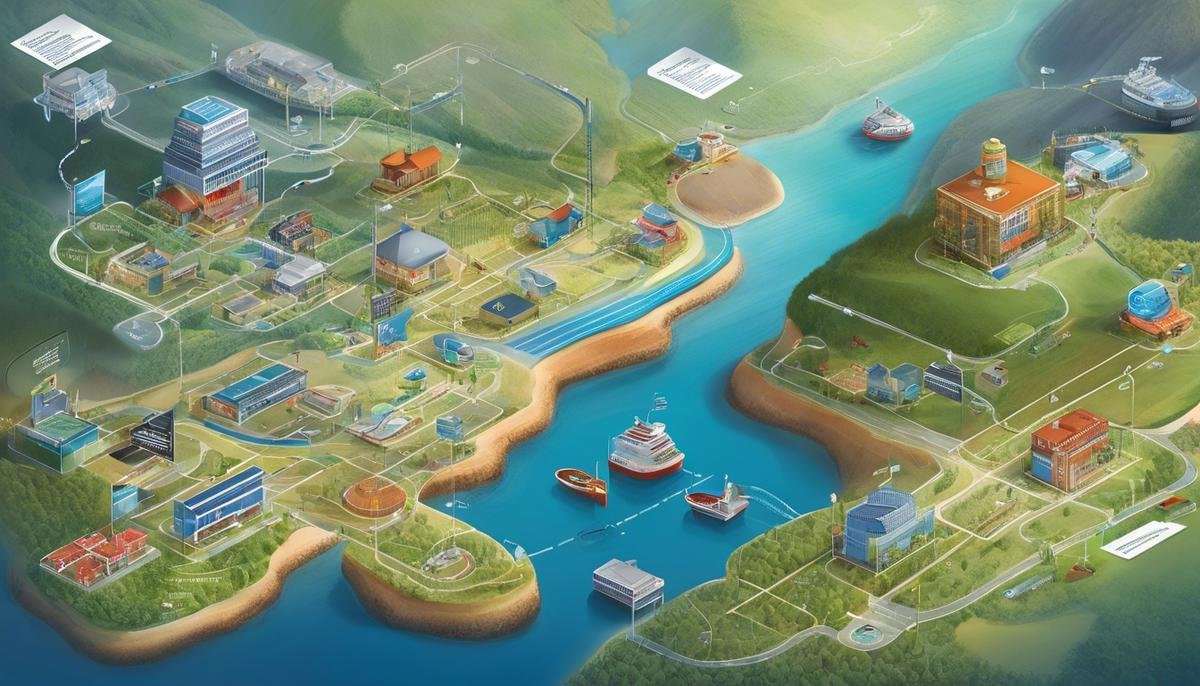
The future undoubtedly holds intriguing possibilities for AutoGPT, shaping and reshaping its applications and optimisations. While it currently stands as a potent avenue for executing language-related tasks, it’s essential to lend clarity to the myriad areas that demand further refinement. Comprehending its present operations, juxtaposing it with other models, and scrupulously understanding its real-world implications contribute to form a precise understanding of its existing latitude and potential trajectory. However, the true power of AutoGPT lies in the realm of the unforeseen, the possibilities it could unlock that we have yet to envisage. Harnessing its potential wholly requires not just comprehending what it is today, but also what it could be tomorrow.