Predictive analytics – a term often intertwined with modern industries, and rightfully so, as it holds the key to future insights. Balancing between the realms of technology, statistics, and business acumen, predictive analytics generates practical utility in making informed decisions, which impact various industries, from healthcare to finance. It provides a scientific approach toward foreseeing future outcomes based on historical data. This discussion provides an overview of predictive analytics, its varied applications, the pros and cons, and the necessary steps to implement it in business. By the end of it, you should have a firm grasp on this invaluable tool serving today’s data-driven world.
Definition of Predictive Analytics
Definition: Unfolding the Concept of Predictive Analytics
Predictive analytics is the process of using data, machine learning techniques, and statistical algorithms to predict outcomes based on historical patterns and trends. It is a category of data analytics aimed at making predictions about future outcomes based on historical data and analytics techniques such as statistical modeling and machine learning.
At its core, predictive analytics harnesses the power of data to give businesses, healthcare institutions, financial organizations, and even government agencies a clear foresight into what might occur in the future. It’s like constructing a systematic roadmap to future events based on data-driven evidence, rather than gut feelings or conjectures.
Working Mechanism: Making Sense of the Past to Predict the Future
The fundamental working principle of predictive analytics revolves around an array of techniques from data mining, statistics, modeling, machine learning, and artificial intelligence to analyze current data and make predictions about the future.
To make a prediction, predictive analytics uses an existing dataset to create a mathematical model. This model is then used to predict future behavior within a set range of outcomes. Essentially, the model is used to predict or estimate an unseen (future) situation while maintaining statistical information about the prediction.
Importantly, predictive analytics is not about one-size-fits-all solutions. Instead, different models are generated for different problems, and predictive power can vary based on the quality of data used and the appropriateness of the model to the situation at hand.
Core Components: The Building Blocks of Predictive Analytics
The core components of predictive analytics include data collection, data analysis, statistical analysis, predictive modeling, and predictive machine learning.
Data collection involves gathering raw data that will be prepared and analyzed. Once the data is collected, data analysis is carried out to clean, transform, and model data in order to discover useful information, suggest conclusions, and support decision-making.
Statistical analysis, on the other hand, deals with the collection, examination, summarization, and interpretation of numerical data to detect underlying patterns and trends.
Predictive modeling, as previously discussed, uses statistics to predict outcomes. Most often, the event one wants to predict is in the future, but predictive modelling can also be applied to any type of unknown event, regardless of when it occurred.
Finally, predictive machine learning involves teaching a computer to parse data, learn from it, and then make predictions and decisions based on that information. This area is a subfield of artificial intelligence where the idea is to allow machines to learn from data so as to forecast future trends with high accuracy.
Understanding Predictive Analytics and Its Significant Roles
Predictive analytics plays a crucial role in our fast-paced world, furnishing organizations with the means to decode substantial volumes of data, recognize patterns, understand customer behavior, improve overall efficiency and predict potential trends in the future.
For instance, businesses use predictive analytics as a proactive tool for detecting fraud, streamlining marketing campaigns, enhancing operations, and mitigating risk. In the healthcare sector, predictive analytics serves as a guide for predicting disease trends and facilitating preventative measures. Therefore, predictive analytics empowers various industries to make well-informed, forward-thinking decisions.
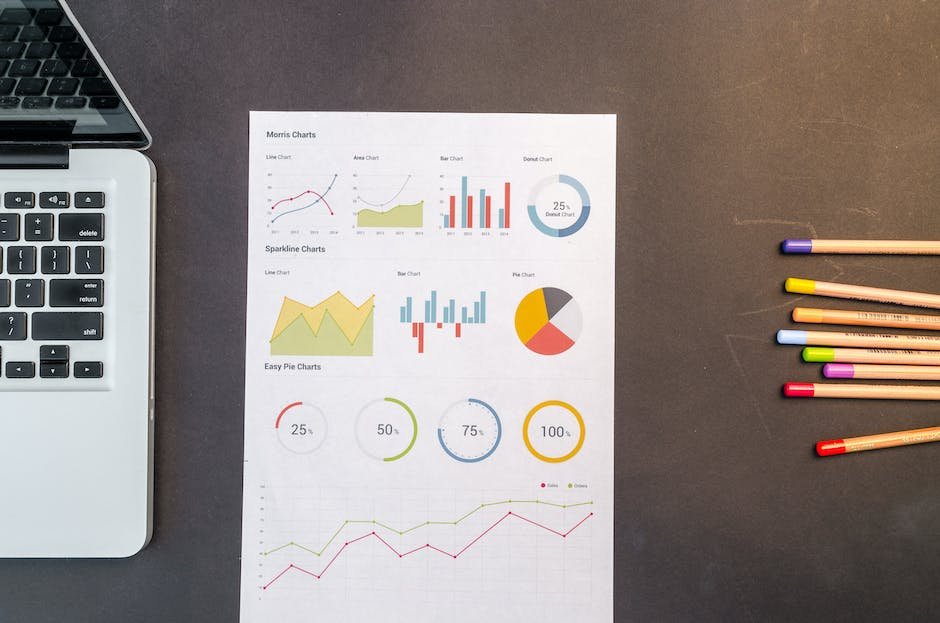
Use Cases of Predictive Analytics
The Vital Role of Predictive Analytics in Healthcare
In healthcare, predictive analytics acts as an invaluable tool, enabling providers to foresee patient needs and avert negative outcomes. Medical facilities harness the power of predictive algorithms to recognize patients at high risk, assisting in the management of chronic ailments and preventing recurrent hospital admissions.
Moreover, predictive analytics contributes to customized care by pinpointing elements that could affect a patient’s wellbeing, from lifestyle habits to genetic indicators. This includes calculating a patient’s probability of developing chronic diseases like heart disease or diabetes, thereby streamlining early intervention.
Finance: Predictive Analytics for Risk Management
In finance, predictive analytics assumes a significant role in reducing risk and maximizing profits. Banks, for instance, frequently use predictive analytics to assess the credit risk of individuals applying for loans.
Insights from these models can help banks identify potential defaulters and make informed decisions on loan approvals or credit limits. Brokerage firms also use predictive models to predict stock market trends and guide investment decisions.
Marketing: Predictive Analytics for Targeted Advertising
In the marketing field, predictive analytics has revolutionized advertising strategies. By analyzing customer behavior, preferences, and demographics, marketers can anticipate customer needs and personalize their marketing campaigns.
For instance, e-commerce companies use predictive models to recommend products based on a customer’s browsing history and prior purchases. These insights help businesses not just in securing repeat purchases, but also in identifying up-sell and cross-sell opportunities.
Supply Chain: Predictive Analytics for Optimized Logistics
Supply chains also benefit immensely from predictive analytics. Predictive models can analyze data from every phase of the supply chain – from sourcing raw materials to delivering finished products – shedding light on potential roadblocks, inefficiencies, and opportunities for cost savings.
These models can forecast demand for products, helping businesses balance inventory levels and avoid stockouts or overstock. They can also predict potential delays in delivery and suggest optimal routes for transportation.
Public Sector: Predictive Analytics for Safer Communities
Even the public sector has incorporated predictive analytics into operation. Several police departments in the U.S. use predictive models to anticipate crime hotspots and optimally allocate resources.
By analyzing historical crime data and factors like population density, unemployment rate, and season, these models can predict where crimes might occur. This can allow police departments to take preventive measures and ensure safer communities.
Sports: Predictive Analytics for Enhanced Performance
Predictive analytics has also found a place in sports, helping teams optimize player performance and strategize game plans. Coaches use data on player statistics, opponent strategies, and even ambient factors like weather or crowd noise to determine game strategies. These predictions can influence player selection, game tactics, and training programs, and ultimately improve team performance.
Closing this train of thought, predictive analytics stands as a multifaceted tool that has the capacity to entirely remodel frameworks and eventual outcomes in several industries. Given its expertise in parsing through historical data to anticipate potential future trends, predictive analytics emerges as an essential resource in our increasingly data-focused society.
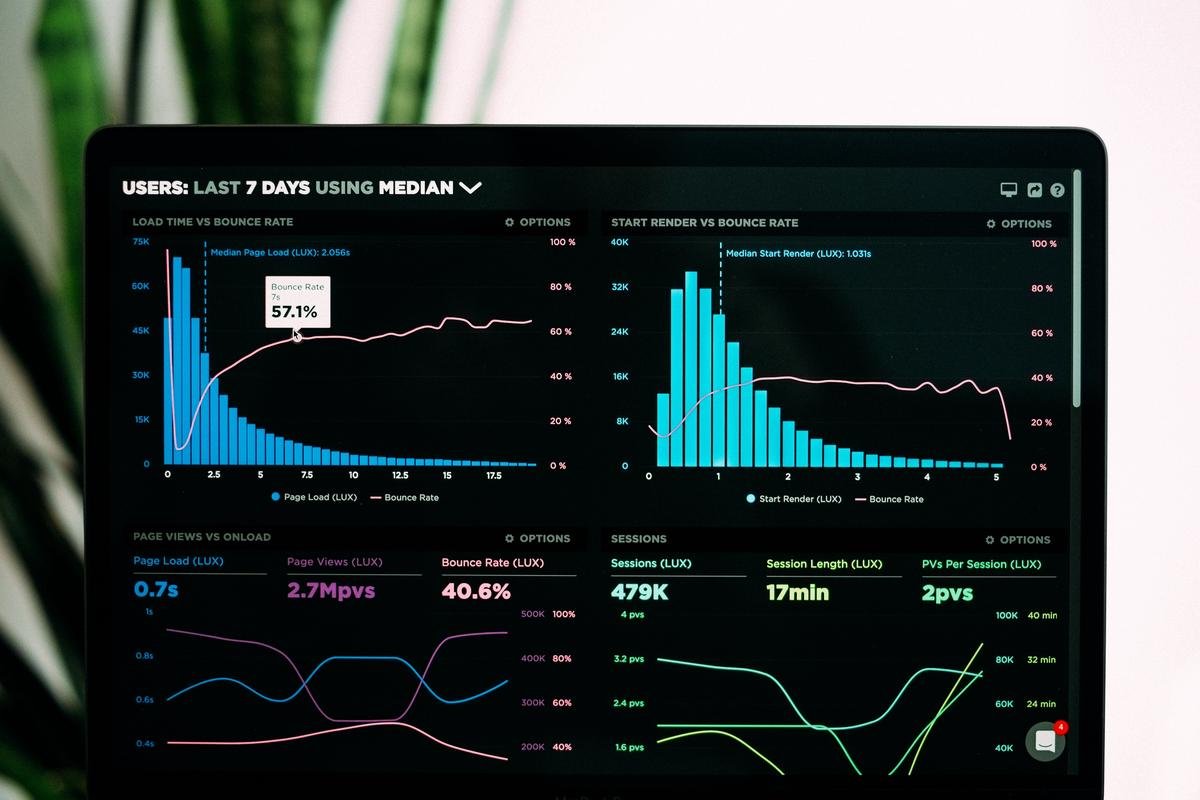
Photo by lukechesser on Unsplash
Benefits and Limitations of Predictive Analytics
Deep Dive into Predictive Analytics
Comprehensively, Predictive Analytics is a branch intertwined with numerous statistical methods hailing from machine learning, data mining, and predictive modeling. This genre of analytics employs various sophisticated techniques such as artificial intelligence, statistical algorithms, and predictive modeling with the aim to project unforeseen future happenings. In the business realm, predictive analytics have been extensively utilized to identify patterns derived from both past and present data, and such insights subsequently enable a projection of potential future occurrences.
Benefits of Predictive Analytics
Predictive analytics not only informs decision-making but enables proactive future-oriented strategic planning. Businesses can better anticipate the needs and behaviors of customers and make well-timed, personalized offers to particular segments, increasing the efficiency of their marketing strategies. Additionally, it can also help in managing resources better by predicting the demand and supply chain trends.
Predictive modeling can also be highly effective for risk mitigation. Credit risk models are a popular application of predictive analytics, helping financial institutions predict the likelihood of a customer defaulting on a loan. Similarly, insurance companies utilize predictive analytics to assess the risk associated with an insurance policy and accordingly determine its pricing.
Predictive analytics has also emerged as a potent tool in the field of healthcare, helping forecast the outbreak of diseases and enabling preventative action. For instance, predictive models were used extensively during the COVID-19 pandemic to anticipate the spread of the virus.
Constraints of Predictive Analytics
While the applications of predictive analytics are vast and beneficial, it is important to factor in its inherent limitations. The effectiveness of predictive analytics is directly dependent on the quality, extent, and relevance of the data used. Inaccurate or incomplete data can lead to flawed predictions, leading to misguided decision-making.
Predictive analytics poses significant privacy concerns as it requires extensive data collection. The utilization of personal information can lead to ethical dilemmas over privacy infringements. Hence, it is crucial to have robust data governance mechanisms to mitigate these issues and maintain customer trust.
Another limitation of predictive analytics is the assumption that future trends will mirror past and current trends. This assumption may not always hold true, especially in cases of unforeseen events that drastically alter fundamental patterns. Predictive algorithms also need to be updated and tweaked regularly to adapt to evolving trends, necessitating ongoing investment and oversight.
Additionally, using predictive analytics requires technological expertise to manage complex tools and interpret analytical outcomes accurately. Businesses need to invest in developing or hiring skilled resources, potentially escalating operational costs.
An Introductory Look at Predictive Analytics
Predictive analytics presents itself as a beneficial capability for insightful decision-making, risk management, and forecasting future happenings. It brings along a myriad of positive aspects, with certain restrictions such as data quality, privacy issues, and technical complexities that businesses need to be mindful of. By integrating predictive analytics within a larger strategic framework, organizations can strike a balance that may help unearth significant growth avenues while effectively curbing the associated risks.
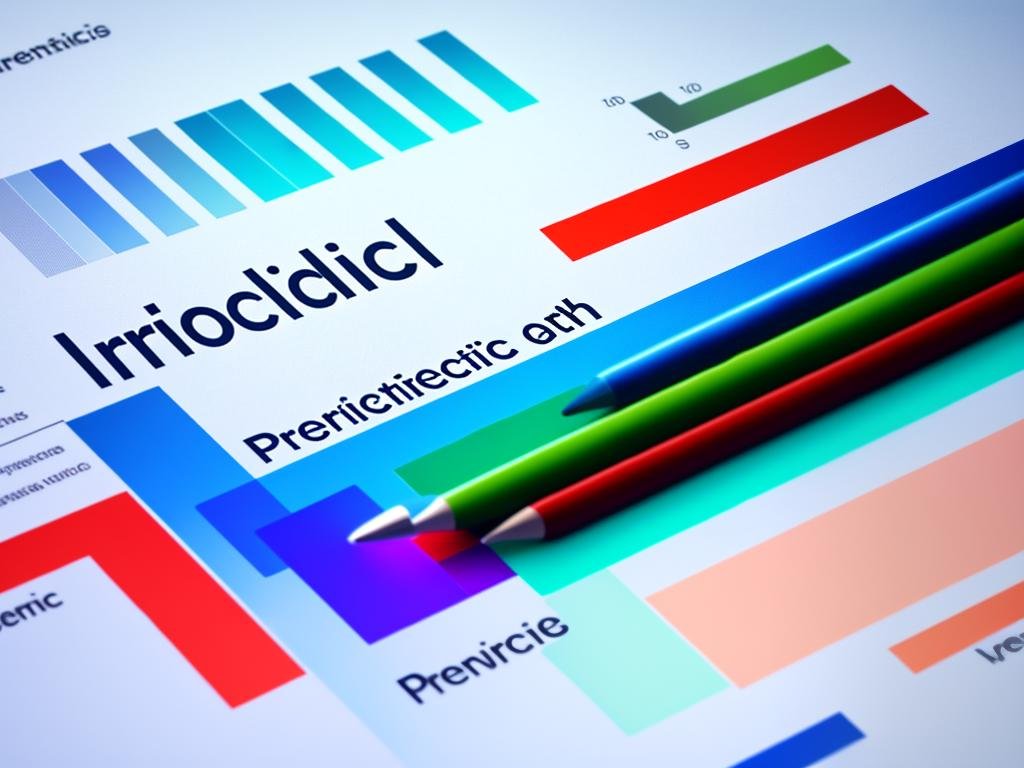
Steps to Implementing Predictive Analytics
Decoding the Concept of Predictive Analytics
Predictive Analytics is a sophisticated branch of analytics geared towards predicting future outcomes. It does this by analyzing historical data through robust techniques such as statistical modeling and machine learning. The goal of predictive analytics isn’t to pinpoint what’s bound to happen next, but rather to predict potential happenings under some degree of uncertainty. It gives a probabilistic estimation about the likelihood of a future event.
Step 1: Defining Project Outcomes
The first step in implementing predictive analytics is to clearly define the outcome of the project. This could be an increase sales, reduce customer churn, or identify fraudulent transactions. It is important to set realistic expectations that align with the objectives of your business.
Step 2: Data Collection
The next step is to collect relevant data that will be used to make the predictions. Data could be collected from various sources like transactional data, customer data, operational data or external data such as demographic or social media data. A large amount of data is necessary for predictive analytics.
Step 3: Data Cleaning
Data cleaning is an important step in the predictive analytics process. This involves cleaning up inconsistencies, dealing with missing values, and removing duplicates in the data. The success of the predictive model largely depends on the quality of the data used.
Step 4: Data Analysis
The collected data is then analyzed using statistical techniques to identify trends, patterns, and relationships within the data. This step involves exploring the data using descriptive and graphical techniques to understand the structure and relationships within the data.
Step 5: Implementing Predictive Models
Based on the results of the data analysis, a predictive model is built using data mining or machine learning techniques. This model is used to predict future outcomes. There are different types of predictive models like regression models, decision trees, and neural networks that can be used depending on the type of data and the business objective.
Step 6: Model Validation
Model validation is a crucial step to test the reliability and accuracy of the predictive model. This involves applying the model to a testing data set and comparing the model’s predictions to the actual outcomes.
Step 7: Deployment of the Model
Once the predictive model is validated, it can be deployed into the production environment for real-time or batch use. This can often involve integrating the model with existing systems or processes.
Step 8: Model Monitoring
The predictive model should be constantly monitored to ensure it is providing accurate predictions. Over time, changes in external factors may affect the model’s accuracy. If this happens, the model may need to be retrained or adjusted.
In conclusion, implementing predictive analytics is not a one-time event but a continuous process. It requires a solid understanding of the business objectives, a large amount of quality data, and appropriate statistical tools and techniques. With accurate predictive analytics, businesses can make informed decisions and increase operational efficiency.
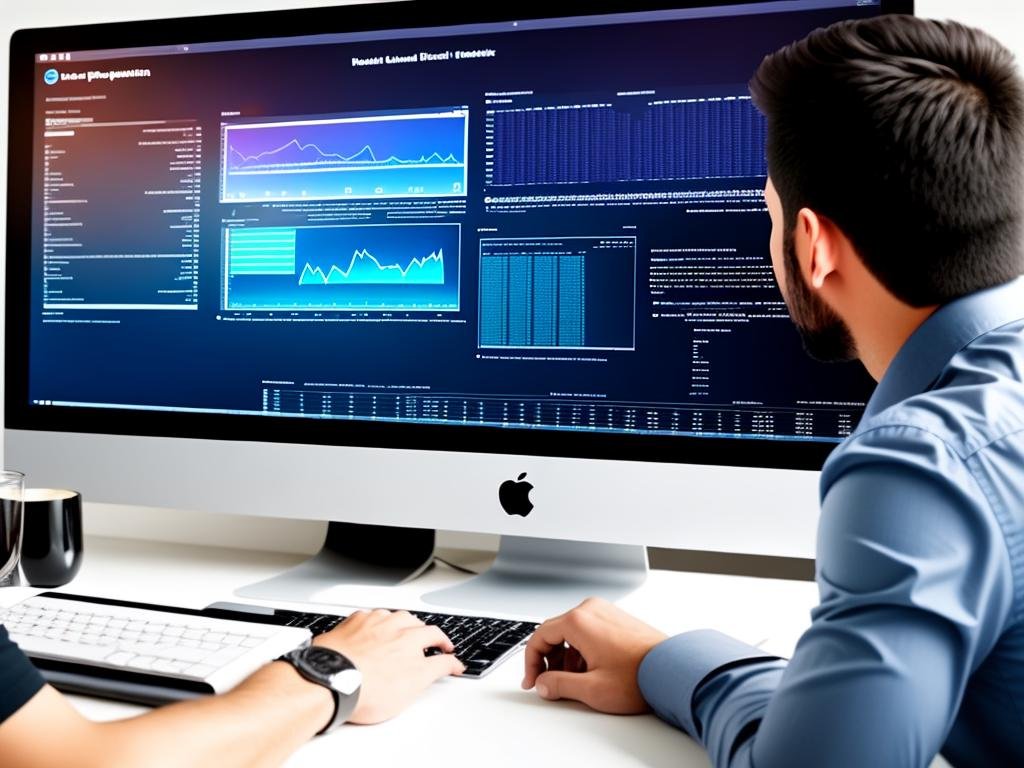
As the reliance on data continues to grow, predictive analytics escalates to being more than just a trend, morphing into a absolute necessity. It’s a union of statistics, artificial intelligence, and data mining, transforming raw data into valuable foresight. While it has significant benefits like aiding choice-making, predicting future trends, and mitigating risks, the limitations of data quality and privacy issues should not be ignored. Regardless, mastering the steps to implement predictive analytics could well be the difference in the evolution of your business, as being able to predict, plan and thus prevail in the competitive market is a defining feature of successful industries. The future undeniably belongs to those willing to embrace predictive analytics for their decision-making processes.