Telecom AI and Its Business Value
Employing state-of-the-art AI technologies, Ericsson is transforming performance in the telecom industry. AI tools actively enhance mobile networks, improving efficiency and customer experiences. Advanced machine learning techniques are being used for tasks like resource allocation, traffic prediction, and real-time anomaly detection, making networks faster, smarter, and more reliable.
Network automation maximizes operational efficiency by reducing manual tasks, cutting costs, and boosting productivity. AI-driven resource allocation optimizes data routing, reducing congestion and enhancing bandwidth and latency in high-demand areas. This automation allows CSPs to dynamically adjust to new situations, maintaining peak performance without constant human oversight.
AI enhances customer experience through cognitive tuning and optimization. Live traffic data helps pinpoint network issues quickly, ensuring users encounter fewer disruptions. Explainable AI models offer transparency in decision-making processes, helping CSPs understand and trust AI-driven suggestions for network adjustments.
AI is evolving business models and use cases. 5G network slicing allows for the creation of customized service plans. Generative AI applications dig deep into behavioral data, enabling personalized services adapted to specific customer needs. This innovation opens new revenue streams while enhancing user satisfaction.
AI techniques like augmented MIMO sleep allow networks to conserve energy without sacrificing performance. Predictive traffic modeling adjusts power use dynamically, reducing operational costs and environmental impact. This technology significantly lowers energy consumption, making networks greener and cheaper to run.
Network security is bolstered through AI applications that detect and prevent threats. AI algorithms actively monitor for unusual patterns, identifying potential breaches or fraud quickly. These systems can implement defensive measures autonomously, safeguarding user data and maintaining network integrity.
Ericsson's AI is also making strides in remote robotics. AI-driven zero-touch operations enable automatic adjustments based on real-time needs, significantly reducing human intervention. These advancements are paving the way for increasingly autonomous network management, bringing about seamless and efficient operations.
In conclusion, AI technologies are reshaping the telecom landscape, driving performance, operational efficiency, customer satisfaction, and sustainability. Ericsson's comprehensive AI solutions enable telecom networks to thrive, adapting quickly to evolving demands and delivering outstanding results.
Zero-Touch Operations
Zero-touch operations are revolutionizing network management by leveraging AI to achieve autonomous functionality, minimizing human intervention. With zero-touch operations, routine tasks such as network configuration, optimization, and maintenance are handled autonomously by AI-powered systems. This vastly reduces the need for manual activities and accelerates the speed at which networks can adapt and respond to changing conditions.
AI-driven zero-touch operations allow for seamless management of network resources, recognizing and rectifying issues in real time. By continuously monitoring network health, these systems can predict and prevent potential failures before they impact user experience. When an anomaly is detected, AI systems can implement corrective actions automatically, ensuring uninterrupted service and minimizing downtime.
The autonomy brought by zero-touch operations translates to enhanced business agility. Telecommunication service providers (CSPs) can deploy and scale network services quickly, adapting to new market demands and technological advancements with ease. This agility is crucial in the rapidly evolving telecom landscape, where staying ahead of the curve is essential for sustaining competitive advantage.
Zero-touch operations enable CSPs to optimize network performance proactively. AI algorithms can anticipate network load changes and dynamically allocate resources to where they are most needed, ensuring optimal performance even during peak usage times. The result is a network that is highly reliable and extremely efficient in its use of resources.
The elimination of manual intervention enhances the scalability and flexibility of network operations. With AI handling the complexity of network management, CSPs can focus on strategic initiatives and customer-centric innovations. Zero-touch operations support a more hands-off approach, freeing up human resources for higher-level decision-making and creative problem-solving.
Ultimately, zero-touch operations signify a paradigm shift, fostering an environment where networks are self-sustaining and self-optimizing. This autonomous approach ensures CSPs can maintain high service levels and quickly address any emerging challenges or opportunities.
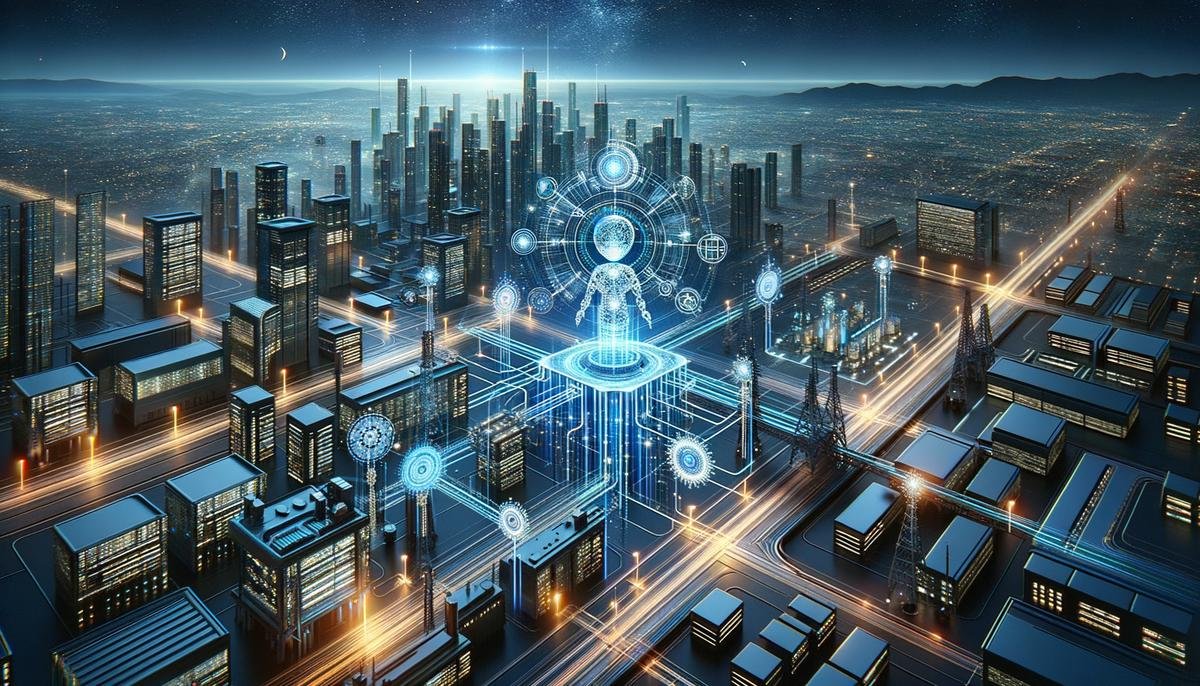
Trustworthy AI
Trustworthy AI begins with transparency, which is achieved through explainable AI models. These models allow AI systems to provide clear, understandable explanations of their decision-making processes, making it easier for operators to trust and verify AI-driven outcomes. By demystifying how AI reaches its conclusions, explainable AI builds confidence among CSPs and end-users alike, ensuring that these technologies are viewed as reliable and accountable partners in network management.
Human oversight remains a vital component in trustworthy AI. While AI technologies can autonomously handle many tasks, human expertise is essential to oversee AI operations and intervene when necessary. This oversight ensures that AI implementations align with ethical standards and operational goals. It also adds a layer of accountability, where human operators can scrutinize AI decisions, thus fostering a symbiotic relationship between AI-powered systems and human expertise.
Security is another cornerstone of building trust in AI. With the increasing sophistication of cyber threats, AI systems must be designed with robust security measures to protect against vulnerabilities. This includes:
- Secure coding practices
- Regular security audits
- Implementation of advanced threat detection algorithms
By preemptively identifying and mitigating risks, AI systems can protect sensitive data and maintain the integrity of telecom networks, reinforcing trust among users.
Built-in safety mechanisms further elevate the trustworthiness of AI in telecommunications. These mechanisms ensure that AI systems adhere to predefined safety protocols, preventing them from making decisions that could jeopardize network stability or user security. Such safety measures act as safeguards, automatically correcting anomalous behavior and alerting human operators to potential issues. This preemptive approach enhances the reliability and safety of AI systems, making them dependable tools in complex network environments.
To realize the full potential of AI in the telecom domain, establishing ethical guidelines and governance frameworks is essential. These frameworks set the standards for AI usage, ensuring that technologies are developed and deployed in a manner that respects privacy, fairness, and accountability. By adhering to these principles, Ericsson aims to create AI systems that perform effectively and ethically, thus securing long-term trust from all stakeholders.
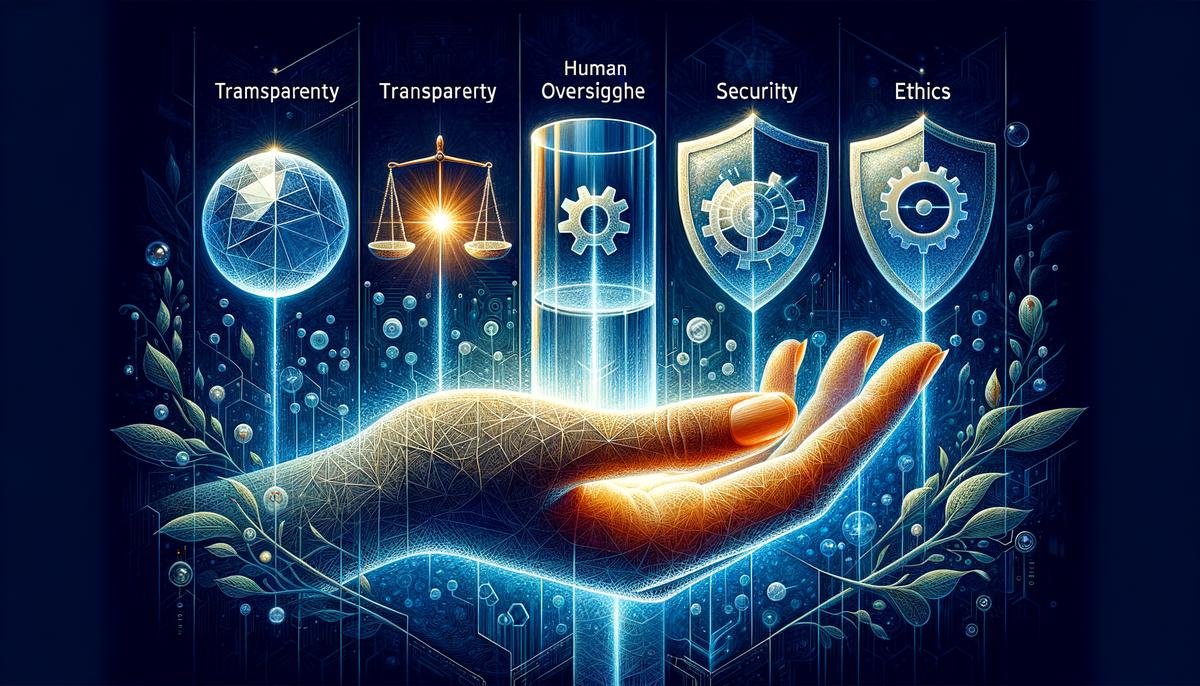
AI in Network Optimization
AI in network optimization is revolutionizing telecom operations by improving performance, resource management, and quality of service. Advanced AI technologies are applied at various stages, ensuring networks are faster, more efficient, and resilient to challenges.
Dynamic resource allocation represents a cornerstone in AI-driven network optimization. AI leverages powerful algorithms to dynamically allocate resources based on current network conditions and usage patterns. This adaptive approach optimizes the distribution of bandwidth, computational resources, and spectrum usage, ensuring that the network operates efficiently under varying loads. For example, during peak traffic periods, AI can redistribute resources to high-demand areas, thus preventing congestion and maintaining optimal performance.1 Conversely, in low-usage periods, it can reduce resource allocation to save energy and operational costs.
Anomaly detection is another critical application of AI in network optimization. Telecom networks generate vast amounts of data, and within this data, patterns can indicate normal operations or signal potential issues. AI systems, equipped with machine learning capabilities, continuously analyze this data to detect anomalies that may signify faults, performance degradation, or security threats. By identifying these anomalies in real time, AI enables proactive maintenance and swift resolution of issues, thereby minimizing downtime and ensuring a consistent user experience.
Quality-of-service (QoS) optimization is fundamentally enhanced through AI, improving how networks deliver consistent, high-quality experiences to end-users. AI-driven systems monitor key performance indicators (KPIs) such as latency, jitter, and packet loss, dynamically adjusting network parameters to optimize these metrics. For instance, machine learning algorithms can predict traffic bottlenecks and reroute data to less congested pathways, ensuring steady service quality even during high traffic conditions.2 This real-time adaptability maintains and improves the user experience, keeping network performance aligned with business objectives and user expectations.
AI facilitates predictive maintenance, which is a game-changer in network optimization. By predicting potential failures before they occur, AI empowers CSPs to address issues preemptively, avoiding service disruptions and costly emergency repairs. Predictive analytics use historical and real-time data to forecast where and when maintenance should be performed, optimizing operational efficiency and extending the lifespan of network components.
In integrating AI into network operations, Ericsson exemplifies how leveraging these advanced technologies transforms network performance. Their solutions offer end-to-end enhancements, from core networks to edge devices, ensuring every component operates at peak efficiency. This holistic approach ensures that CSPs can extract maximum value from their existing infrastructure while being well-prepared for future advancements.
AI and Energy Efficiency
AI has the potential to revolutionize energy efficiency in telecom networks. By integrating AI-driven solutions, Ericsson optimizes energy consumption, addressing both operational costs and environmental sustainability.
One notable technique is augmented MIMO sleep. Multiple-Input Multiple-Output (MIMO) technology, widely utilized in modern networks, involves the use of multiple transmitters and receivers to improve communication performance. However, maintaining these multiple active channels consumes significant energy. AI algorithms can predict traffic patterns and autonomously adjust the number of active MIMO channels in real-time, switching off unnecessary antennas during low traffic periods. This predictive power ensures that energy is conserved without compromising network performance.
Intelligent energy optimization leverages AI to undertake a comprehensive analysis of the network's operational data, identifying areas where energy use can be minimized. By analyzing historical usage patterns, current traffic loads, and predictive models, AI systems can dynamically adjust network parameters to optimize energy consumption. For example, during off-peak hours, AI can reduce the power to underutilized network components, lowering overall energy usage without affecting service quality. Such measures cut operational expenses and contribute to broader sustainability initiatives by reducing the network's carbon footprint.
Ericsson's AI solutions enable continuous monitoring and fine-tuning of energy expenditure across diverse network components. Machine learning models can pinpoint inefficiencies and suggest more energy-efficient configurations, promoting a sustainable operational approach. These AI-driven adjustments ensure that every watt of energy expended is used optimally, enhancing the environmental stewardship of telecom networks.
Predictive traffic modeling is another significant AI application in energy management. By forecasting future network usage based on various data inputs, AI can pre-emptively adjust network resources to meet anticipated demand efficiently. This proactive approach helps in preventing over-provisioning, which often leads to unnecessary energy consumption. Instead, resources are allocated precisely where and when needed, maintaining service quality while optimizing power usage.
In practice, these AI techniques have demonstrated substantial energy savings. For instance, in proof-of-concept trials, AI-driven energy optimization solutions have shown up to 15% reductions in energy operating expenses (OPEX)1. The ability to conduct real-time adjustments and implement energy-saving strategies autonomously means that these benefits can be sustained over the long term.
The impact of AI in energy efficiency extends to the granular level of individual network elements. For example, AI can manage the energy usage of radio access networks (RAN), which are among the most power-hungry components of telecom infrastructure. By employing advanced AI algorithms, Ericsson has seen success in reducing energy expenditure through smarter RAN operations, such as AI-driven sleep modes and load balancing.
Additionally, AI-driven energy efficiency measures align with the growing corporate emphasis on sustainability. As regulatory pressures and consumer expectations for greener practices rise, telecom operators using AI for intelligent energy management can reduce costs, improve operational efficiency, and enhance their sustainability credentials. This dual benefit reinforces the strategic value of investing in AI technologies for energy optimization.
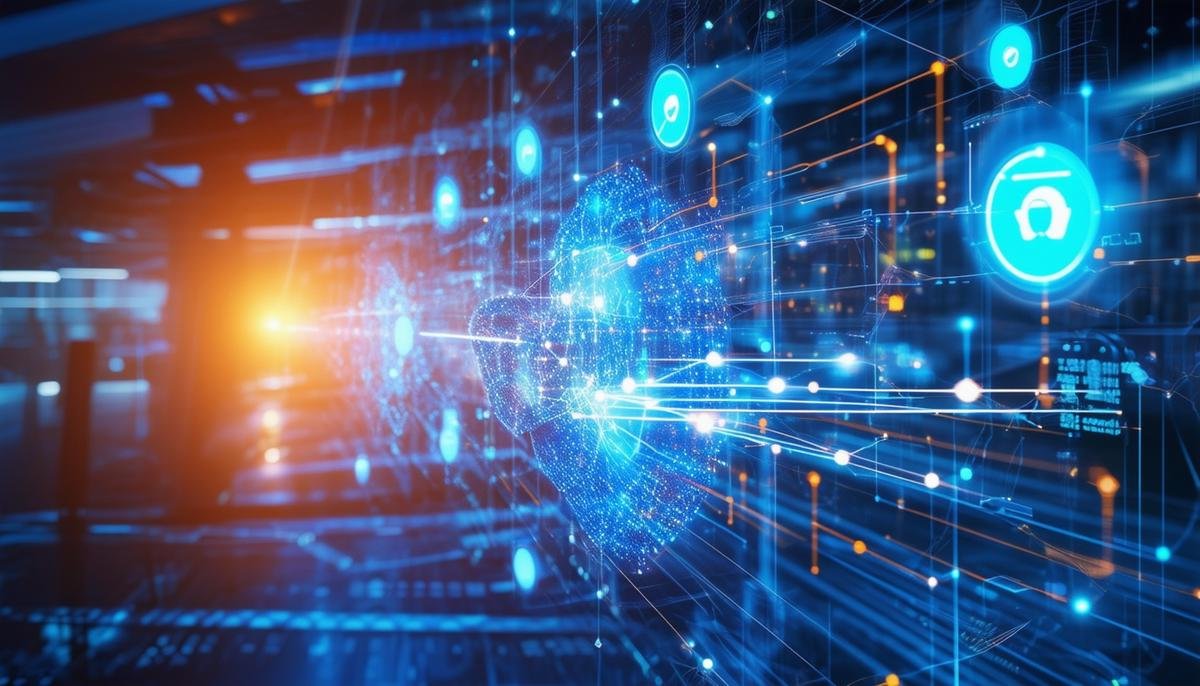
AI-Driven Security Enhancements
AI plays a pivotal role in enhancing network security within the telecommunications sector, providing sophisticated tools to detect and prevent cyber threats, mitigate fraud, and implement zero trust architecture principles. These advanced measures are critical in safeguarding the integrity and reliability of modern telecom networks amidst an escalating landscape of digital threats.
AI's ability to detect and prevent cyber threats with accuracy and speed is at the forefront of AI-driven security. Traditional methods of threat detection often rely on predefined rules and periodic system checks, which may miss emerging or novel threats. AI, on the other hand, employs continuous monitoring and machine learning algorithms to analyze vast volumes of network data in real-time. This capability allows AI systems to identify unusual patterns or anomalies that could indicate a cyberattack, such as:
- Sudden spikes in traffic
- Unusual access configurations
- Data exfiltration activities
Machine learning models, trained on historical data and real-world threat scenarios, can differentiate between legitimate activities and potential security breaches with high precision. When a threat is detected, AI systems can autonomously initiate defensive measures, such as isolating affected segments of the network, blocking suspicious IP addresses, or alerting security personnel for further investigation. This automated response reduces the latency in addressing threats, minimizing potential damage and ensuring continuous protection.
Fraud detection also benefits from AI's capabilities. Telecom networks are frequent targets of fraudulent activities, including SIM swap fraud, unauthorized account access, and billing fraud. AI algorithms can analyze user behavior patterns to detect anomalies indicative of fraud. By monitoring aspects like call patterns, usage frequency, and location data, AI systems can flag unusual activities that deviate from typical user behavior. For instance, a sudden change in call destinations or an unexpected roaming activity may trigger an alert for potential fraud. These insights enable telecom operators to take proactive measures, such as verifying user identities or temporarily suspending suspicious accounts to prevent financial losses and protect customer data.
The implementation of zero trust architecture is transformed through AI's advanced monitoring and authentication capabilities. Zero trust operates on the principle that no user or device, whether inside or outside the network, should be inherently trusted. Every access request must be authenticated and continuously verified. AI enhances zero trust by integrating micro perimeter protection, mutual session-based authentication, and continuous monitoring specifically for 5G networks.
AI-driven systems dynamically assess the security posture of each device and session, ensuring continuous compliance with security policies. This includes monitoring configuration settings, application behavior, and network traffic for potential vulnerabilities or breaches. If an anomaly is detected, AI systems can enforce stricter access controls, initiate multi-factor authentication, or even revoke access to safeguard the network. By maintaining a holistic view of network security, AI ensures that every interaction is scrutinized and validated to prevent unauthorized access and mitigate risks.
Furthermore, AI's role in zero trust architecture extends to the detection of sophisticated threats like false base stations, which can intercept and manipulate mobile communications. AI systems can identify the unique signatures of legitimate base stations, distinguishing them from rogue entities attempting to breach network security2. This capability is crucial for maintaining the integrity and confidentiality of communications within 5G networks.
Incorporating AI into network security practices enhances protective measures and aligns with regulatory requirements and industry standards. Telecom operators can leverage AI to ensure compliance with data protection regulations and mitigate the risk of data breaches. By embedding AI into their security frameworks, CSPs demonstrate a commitment to safeguarding user data and building trust with customers and stakeholders.
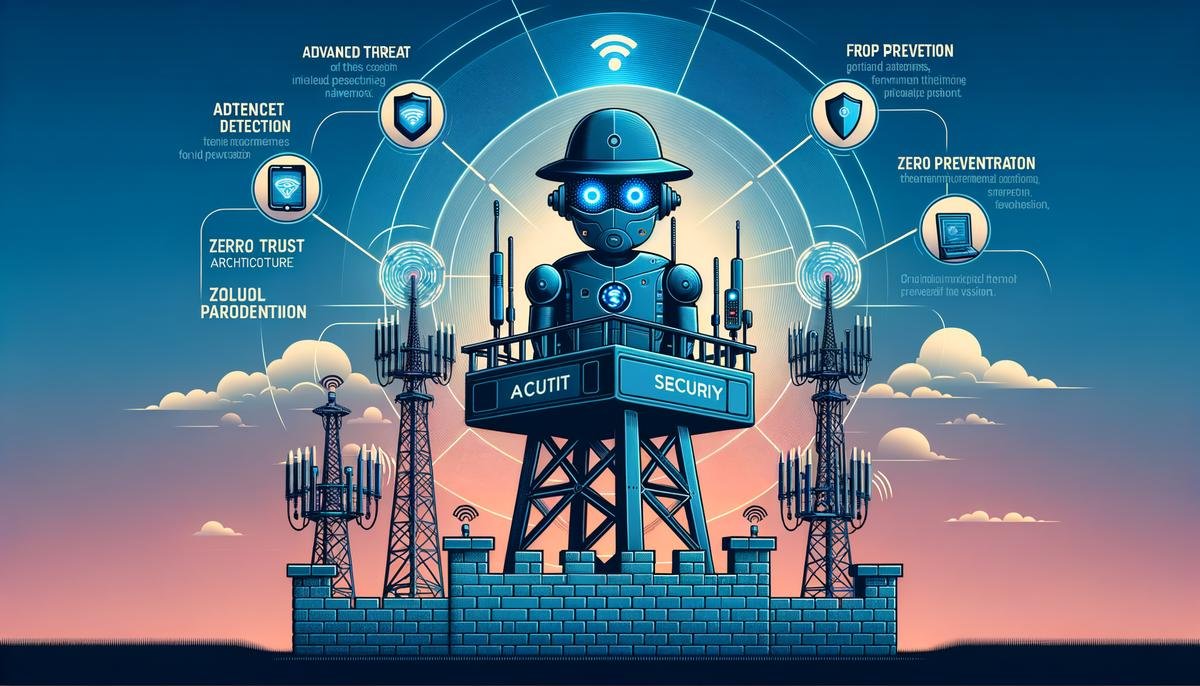