As we stand on the precipice of a technological revolution, Google Bard emerges as a beacon of artificial intelligence’s potential, as well as a mirror reflecting the complexities and challenges this technology must navigate. Venturing beneath the surface of its conversational prowess, we unravel the deep-seated technical roots which underpin this AI phenomenon. From machine learning algorithms to neural network architectures, and the expansive language models forming its core, each element is pivotal in constructing the vibrant tapestry of Bard’s capabilities. Nonetheless, understanding these technical cornerstones brings to light a landscape riddled with challenges. These hurdles include formidable constraints like raw processing firepower, the pivotal yet sometimes flawed quality of input data, and intrinsic model biases that shape every outcome.
The Technical Foundations of Google Bard
The design of Google Bard is anchored in the sophisticated realm of artificial intelligence, specifically leveraging a framework known as generative AI. At its heart, the system is driven by models which are a subset of machine learning, called language models. These computational structures are adept at predicting and generating human-like text, drawing on extensive databases of written language.
The primary technical principle is natural language understanding, which is a computer’s ability to comprehend human language in the way it is naturally spoken or written. To achieve this, Google Bard makes use of transformer-based architectures. This innovation, originally showcased in models such as BERT and GPT, exhibits a novel approach called attention mechanisms that enable these models to focus on different parts of the input data, much as a human would when trying to understand or create a coherent narrative.
Another key component is context retention, which is essential for producing responses that are not only grammatically correct but contextually relevant across several exchanges. Google Bard employs advanced techniques to maintain context by using its vast knowledge base to embed a consistent theme or topic within an ongoing conversation, despite the complexity this involves.
The underlying system is also fine-tuned through a process called reinforcement learning from human feedback (RLHF). In this process, the model is initially trained with large datasets and then refined through iterations based on human feedback to align its outputs more closely with human values, preferences, and nuances in language.
A related principle is the scalability of knowledge. Google Bard is designed to be dynamically updated with information, which means it is consistently trained on new data to expand its knowledge base. This is crucial in keeping the AI current, as it must understand and generate text that reflects the latest events, discoveries, and colloquialisms.
Lastly, ethical considerations are woven into the fabric of Google Bard’s design. The developers of such systems must be ever vigilant about biases that could be encoded within these language models. This involves both the identification and mitigation of inadvertent biases from the training datasets and extends to ensuring privacy and user safety in the generated content.
Google Bard’s infrastructure demonstrates an intersection of profound language understanding, context retention, continual learning, and ethical responsibility. The technical prowess of this system marks a significant stride forward in artificial intelligence adventures, promising to have far-reaching implications across various fields that make use of conversational AI.
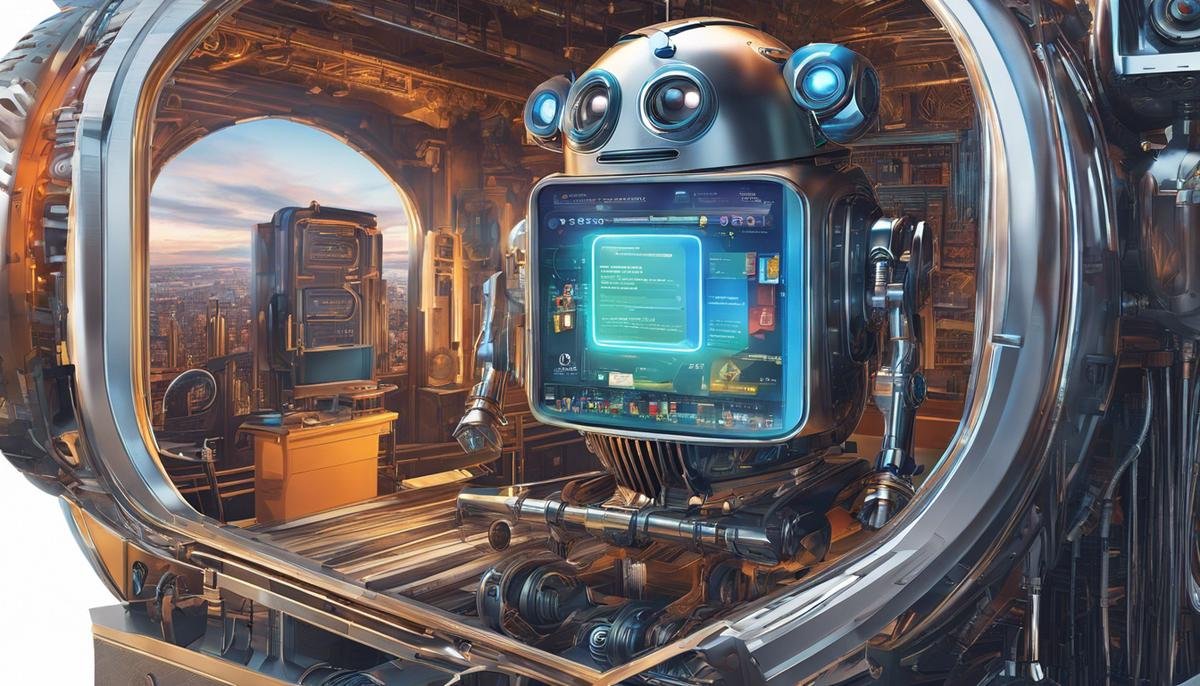
Data Dependency and Quality Issues
To comprehend how data quality and sourcing constraints may circumscribe the potential of Google Bard, it is important to delve into the manner in which data organs form the lifeblood of generative AI systems. These advanced entities are nourished by vast food chains of information—datasets meticulously compiled, annotated, and cleansed to facilitate the training of machine learning models.
In the realm of generative AI, the axiom “the quality of an output is only as good as the quality of the input” holds significant sway. Data quality pertains to the accuracy, relevance, and timeliness of the information consumed by AI models. For Google Bard, specifically, subpar data quality could result in the generation of misinformation, rendering the intelligence offered less reliable. Further, with a model that learns through exposure to pre-existing text, the risk of propagating entrenched inaccuracies or outdated information becomes not just possible, but likely, without vigilant oversight of data quality.
Sourcing constraints present a parallel challenge. The fuel of Google Bard’s knowledge reliance—diverse and authoritative data sources—is circumscribed by availability and accessibility. A paucity of robust information from specialized domains could lead to the underrepresentation of niche fields or emerging topics within its responses. With restrictions or limitations on access to certain databases, possibly due to licensing or privacy concerns, the model’s breadth and depth of understanding might be compromised. Thus, where sourcing constraints are in place, the AI’s capacity to provide knowledgeable discourse on less common subjects could be markedly diminished.
Moreover, sourcing constraints are not merely external hurdles; they are also shaped by internal policy decisions concerning data governance. The selection process that determines which sources are deemed credible and which are not is fraught with subjective judgement. For instance, biases could inadvertently seep into the framework if the source curation lacks inclusivity or a representative spectrum of viewpoints.
In essence, generative AI systems such as Google Bard are remarkable feats of computational ingenuity, but they are not without their Achilles’ heel. Data quality and sourcing constraints can undermine the veracity and the variety of the intelligence dispensed by these digital oracles. Optimization of data handling practices and exploration of a broader galaxy of sources are pivotal in order to ensure that generative AI remains a trustworthy and comprehensive companion in the information age.
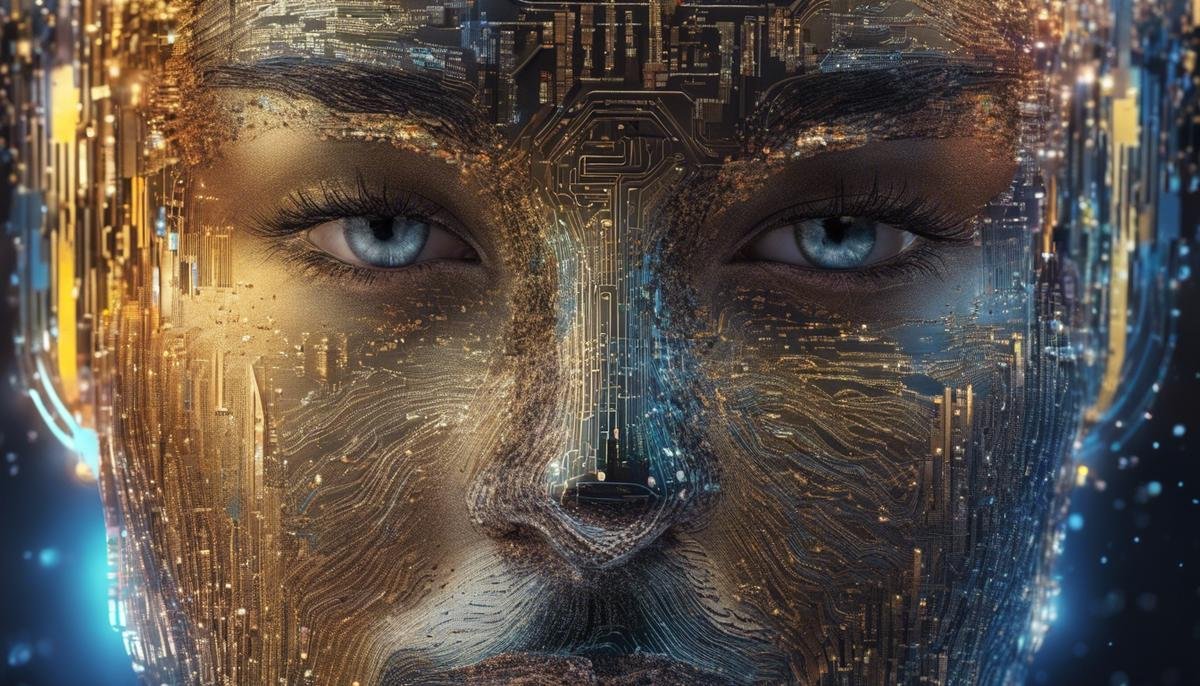
Understanding Google Bard’s AI Ethics
The Ethical Dilemmas of Content Creation with Google Bard
In the wake of technological advancements in the realm of artificial intelligence (AI), Google Bard emerges as a forefront generative AI, tasked with the creation of seemingly sentient dialogues and narratives. Despite its sophisticated architecture and machine learning prowess, Google Bard faces a plethora of ethical challenges beyond those traditionally associated with computational systems.
One paramount ethical conundrum resides in the domain of content creation autonomy. The capacity of Google Bard to craft content that may influence public opinions, shape social discourse, or even manipulate behaviors harbors a responsibility of monumental scale. The degree to which a generative AI like Google Bard should be permitted to generate content without direct human oversight stands as a contentious ethical debate. Questions arise regarding the autonomy limits and the necessary safeguarding against the AI’s inadvertent perpetuation of harmful narratives or ideologies.
Additionally, the issue of accountability emerges in cases of erroneous or detrimental information disseminated by the AI. Considering the ability of systems akin to Google Bard to reach vast audiences instantaneously, determining who bears the onus for the repercussions of misguided outputs becomes crucial. Is it the creators, the custodians, or the machine itself? The lines of accountability often blur, and the clear designation of responsibility remains a topic requiring urgent and meticulous consideration.
Moreover, as Google Bard learns and evolves from the copious data streams it ingests, the potential for intellectual property infringement presents itself. Content generated by the AI could inadvertently mimic human-created works without attribution. This occurrence brings forth a host of ethical and legal implications, challenging the boundaries of copyright law and the protection of original content within the digital landscape.
Another ethical quandary lies in the effect of commodification on the output of conversational AI. As enterprises increasingly employ such technologies for profitability, ethical concerns regarding the commodification of conversation and the phenomenon of deception, where users may be misled to believe they are interacting with a human rather than an AI, come to the fore. The delicate balance between technological innovation and the preservation of authentic human interactions demands careful deliberation.
Finally, the normalization of interactions with AI agents, such as Google Bard, cultivates a societal landscape where the delineation between human and AI conversation becomes increasingly obscured. This reality beckons critical exploration into the psychological and social implications of such integrations. The potential for reduced human contact and the consequent effects on interpersonal skills and empathy within society are of paramount consideration.
In sum, these ethical challenges present complex, interrelated issues that compound as AI technologies, like Google Bard, continue to advance and proliferate. The ethical dilemmas are not purely academic but are matters with tangible consequences on individuals, societies, and the very fabric of human interaction. It is incumbent upon the scientific community, legislators, and society at large to engage in robust, proactive discussions to navigate these ethical quandaries and to ensure the responsible integration of artificial intelligence into the social milieu.
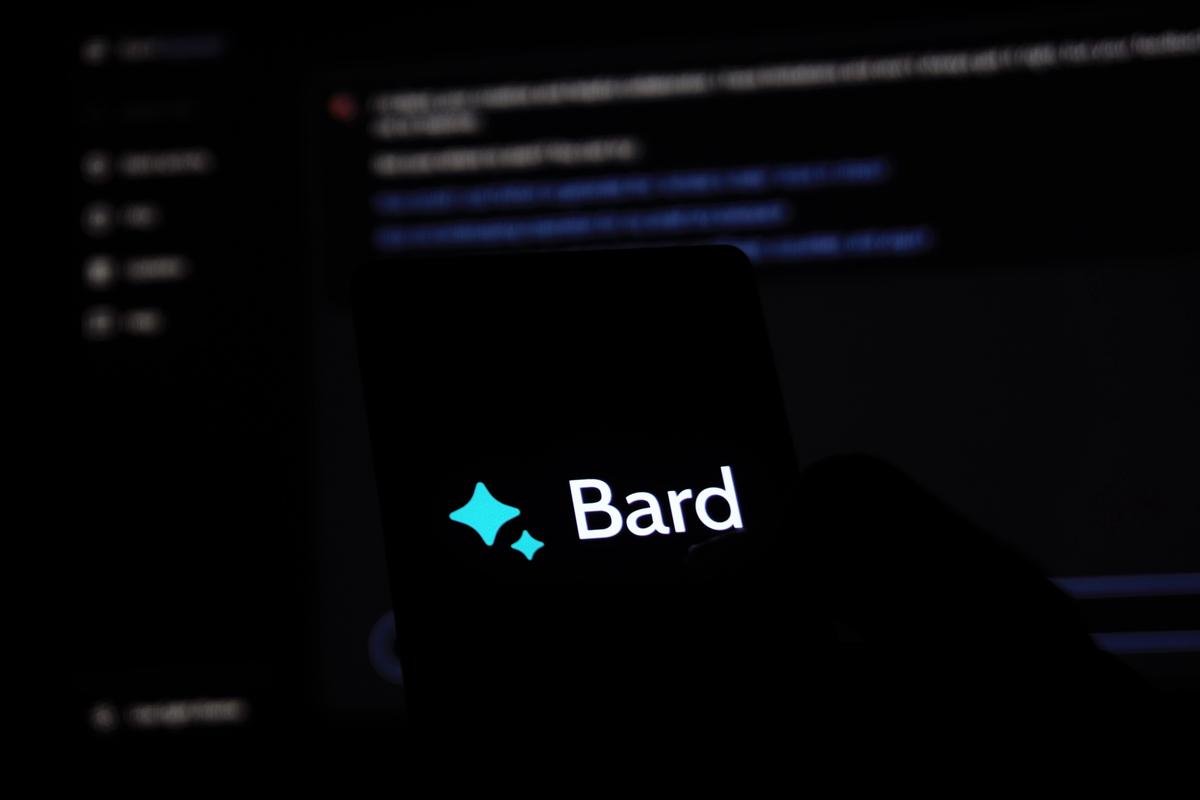
Photo by iammottakin on Unsplash
User Interaction Constraints
Understanding the Limitations of Google Bard’s User Interaction
The realm of user interaction with artificial intelligence, such as Google’s Bard, is subject to crucial limitations that define the extent to which these advanced conversational systems can genuinely fulfill the multifaceted demands placed upon them by end-users. Given the profound intricacies we have already explored—ranging from the nuances of machine learning to the societal implications of conversational AI—our focus now shifts to identify the constraints inherent within these systems.
Primarily, the user’s engagement with Google Bard is bounded by the inherent limitations of language processing. Despite being equipped with sophisticated algorithms, Bard may not seamlessly grasp the full spectrum of human emotion and subtlety in communication. What may be intuitively obvious to a person could potentially baffle the AI, impeding the natural ebb and flow of conversation.
Moreover, the depth and breadth of interactive potential are contingent on the robustness of Bard’s underlying data sets. Since the information domain it can access is finite and pre-selected, Bard might not be equipped to converse authoritatively on all topics, particularly the very latest developments or highly specialized subjects not well-represented in its data sources.
Another pivotal constraint relates to the interface modality through which interactions take place. Users primarily engage with Bard through text, which relinquishes the layers of meaning conveyed through tone, inflection, and body language in human-to-human interaction. This limited modality can result in a communication gap where intention and interpretation may misalign.
Handling ambiguity stands as a formidable challenge. Human language is riddled with it; words and phrases carry multiple connotations and meanings. While Bard’s algorithms strive to navigate these murky waters, it’s conceivable that the AI might err or falter in deducing the user’s intended meaning, particularly in more complex or less straightforward exchanges.
Google Bard must also operate within a framework that complies with legal and ethical norms. It’s designed not to create or distribute content that violates copyrights, incites harm, or perpetuates unlawful behaviors. These necessary restrictions, while ensuring essential legal and ethical compliance, can also put a damper on the freedom that users might experience in their conversational adventures.
The platform’s reliance on continual learning and updates poses a limitation of a temporal nature. Human knowledge evolves rapidly, and there can be a significant time lag before Bard can integrate new insights into its repertoire, potentially leaving users with outdated information in areas where currentness is critical.
Furthermore, the platform’s capacity for user interaction hinges on the assumption of digital equity—access to reliable internet and modern digital devices. In areas where such resources are scarce, the potential for interacting with Bard is dramatically reduced, thus excluding segments of the global population from benefiting from these technological advancements.
In conclusion, the boundaries of user interaction with Google Bard are shaped by a confluence of technological, legal, ethical, and socio-economic factors. In awareness of these delimitations, the relentless pursuit of enhancement in AI technology continues, guided by a profound understanding that such systems are but a facet of the broader human quest for knowledge, connection, and progress.
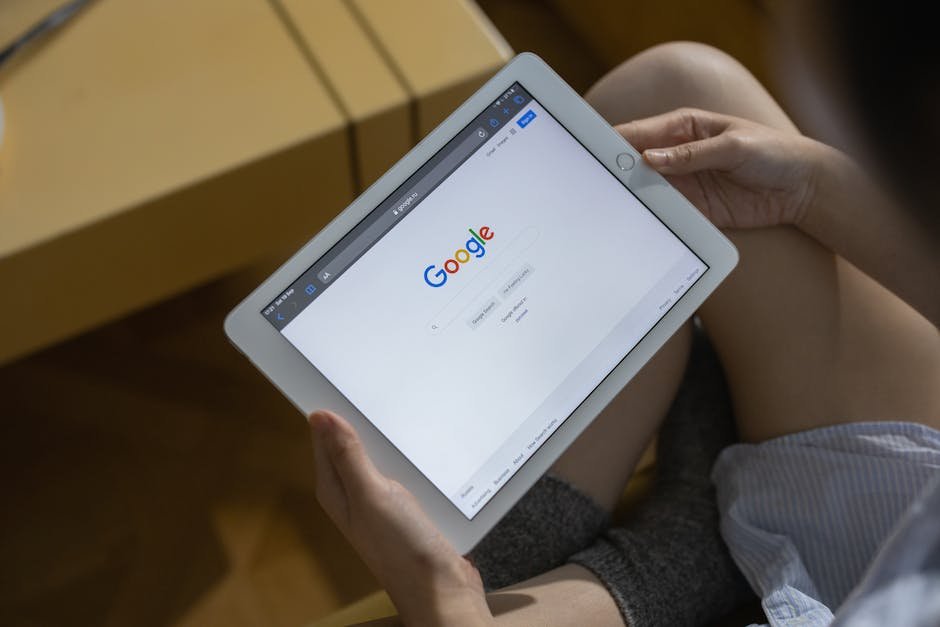
Limitations Imposed by Current Language Model Capabilities
Understanding the User Experience with Google Bard’s Conversational Abilities
As we delve further into the considerations surrounding language model capabilities—more specifically, the application of Google Bard—it is essential to explore dimensions of the user experience in this technological discourse. Google Bard, engineered to harness the power of language comprehension and generation to facilitate interaction, has an interesting dimension worth analyzing: the user engagement and satisfaction metrics.
Drawing from extensive research in the field, we recognize the critical interplay between user expectations and the language model’s interpretive accuracy. Given that users approach Google Bard with diverse communicative intents and varying levels of linguistic complexity, the model’s ability to ascertain user needs and respond with precision remains paramount. However, this interaction is not infallible—it is held hostage by the model’s training and its encapsulation of grammatical nuances, idiomatic expressions, and cultural references which users invariably inject into their queries and statements.
Moreover, there is the indispensable factor of responsiveness time. As interactions with Google Bard are digital and real-time, users anticipate immediate feedback, a pace at which even the most advanced AI systems need to sustain efficiency. This becomes pivotal in maintaining user engagement, as delays or prolonged processing can lead to frustration and attrition—a concept well-understood in the discourse of user-centric design and human-computer interaction research.
One cannot overemphasize the importance of system adaptability in encountering non-standard language use cases such as slang, code-switching, or emerging vernacular. The human language is a testament to diversity and evolution, requiring Google Bard to continuously adapt beyond formal and conventional expressions. The scope of the language model must embrace the vast expanse of human creativity in language deployment, an area ongoing research is arduously working to accommodate.
In evaluating these interactions, we also recognize the potential for educational applications. Google Bard’s interpretative scope directly influences its aptness as a tool for knowledge dissemination and learning enhancement. It is to be noted that learners gravitate towards technology that offers immediate, correct, and comprehensible assistance in their educational endeavors. The ability of AI-driven platforms to provide just-in-time information and clarify concepts contributes significantly to personalized learning experiences, fostering an environment conducive to knowledge gain and skill development.
In conclusion, the parameters defining the user experience with Google Bard hinge upon the fluid and adaptive nature of human-language interaction and the model’s proficiency to accommodate this dynamism. As the interface serves as a nexus between human curiosity and machine-provided information, the continued refinements to improve interactive performance are crucial, ultimately determining the utility and success of Google Bard in daily use. Continuous evaluation and enhancement of these ever-evolving systems are thus imperative to meet the increasing demands of users in a world where information is not only sought-after but expected to be at the fingertips of any inquiring mind.
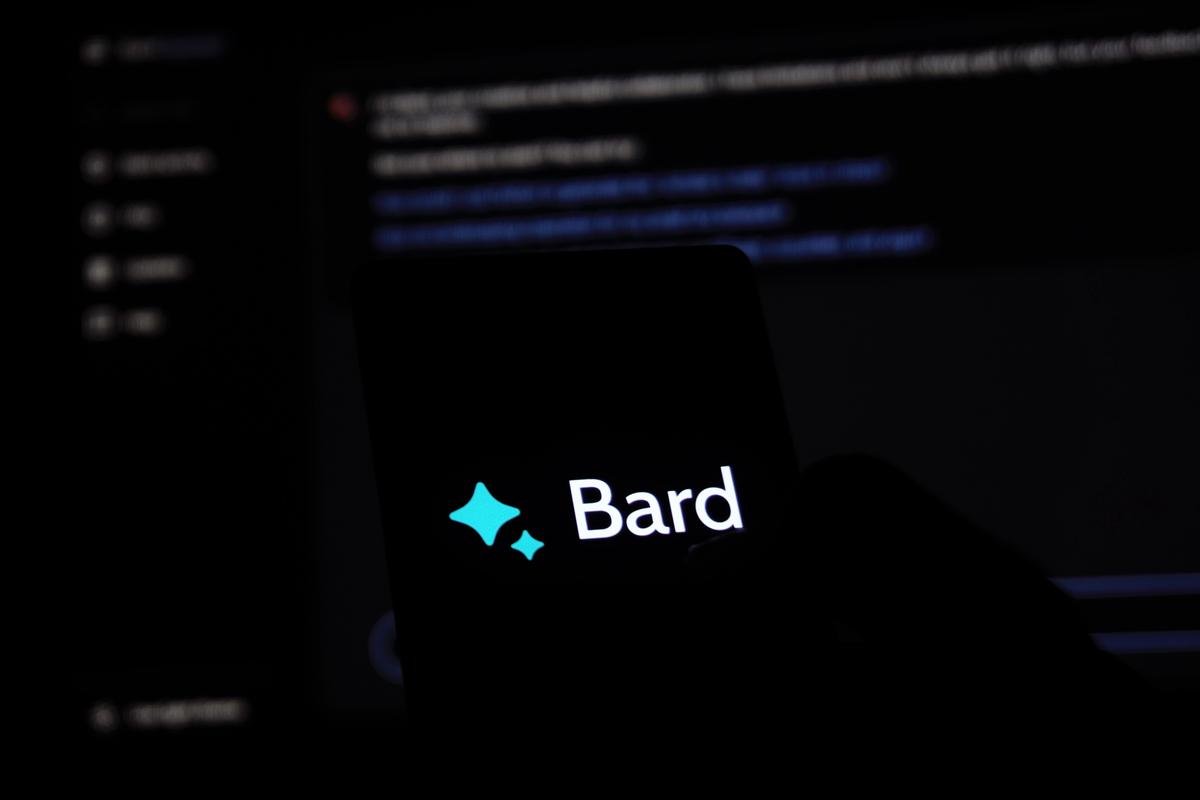
Photo by iammottakin on Unsplash
Future Directions and Potential Advancements
Augmenting Google Bard: Future Pathways to Enhanced Conversational Experiences
The advent of conversational AI platforms like Google Bard has incited transformative shifts in human-computer interaction, laying a foundation for an era where seamless conversation with machine intelligence is not just fantastical but an everyday reality. Given its current capabilities, it’s imperative to explore potential advancements that can further align Google Bard with the vast complexity of human language and the intricate tapestry of user expectations.
- Enhanced Interpretive Precision: The interface between user intents and the AI’s interpretive mechanisms is continually evolving. Future enhancements must focus on heightening the precision of the AI’s interpretive capabilities to align seamlessly with user expectations. This involves developing more sophisticated sentiment analysis to grasp the emotional undercurrents of exchanges and to respond with appropriate empathy or clarity.
- Negotiating Linguistic Nuances: Current systems, whilst adept, may falter with the nuanced cadences of human language, including the use of idioms, colloquialisms, and culturally rooted expressions. Advancing the nuances of language processing to comprehend and utilize such expressions effectively is a formidable pursuit, which requires a deeper engagement with linguistic diversity and cultural context.
- Real-Time Engagement Optimization: Response latency can be a deterrent, causing user abandonment. Upcoming technological endeavors are likely to aim at optimizing the system’s speed without compromising the quality of interactions. Ensuring near real-time responsiveness can significantly bolster user engagement, offering interactions that are as immediate and natural as those among humans.
- Adapting to Linguistic Diversity: Language is a living, breathing entity; new words, phrases, and even entire dialects emerge continually. The inflexibility towards non-standardized uses of language can be restrictive for users. Future iterations of Google Bard may incorporate more pliable language use frameworks, learning from regional dialects, jargon, slang, and new linguistic paradigms as they come into existence.
- Bard as an Educational Ally: The convergence of AI and education presents uncharted territories rich for exploration. Sharing accurate information swiftly is paramount, and Google Bard has the potential to evolve into a premier learning interface. With advancements in factual accuracy and tailored educational responses, Bard can leverage its vast repository of information to become not only a conversational partner but also an indispensable educational resource.
- Dynamic Benchmarking for AI Proficiency: The landscape of user demands is as mutable as the tides. Hence, the continuous evaluation and iterative upgrades are critical for Google Bard to stay relevant and meet the ever-evolving needs of users. Systems that benchmark their performance in real-user environments and adaptively adjust their algorithms will maintain a competitive edge and provide sustained value.
Summarily, Google Bard, like all pioneering technologies, is on an evolutionary trajectory marked by iterative enhancements and breakthroughs. Future advancements aim to not only refine the technical aspects of the platform but also to imbue it with an adaptive finesse that resonates with the richness and fluidity of human expression. Emphasis on context, cultural relevancy, real-time adaptability, and educational prospects will be pillars guiding the future iterations of Google Bard, as it becomes increasingly integrated into the fabric of daily life. The nexus between technological potential and human-centric design will likely shape the destiny of this conversational AI, heralding a future where digital conversations may be indistinguishable from those with another sentient being.
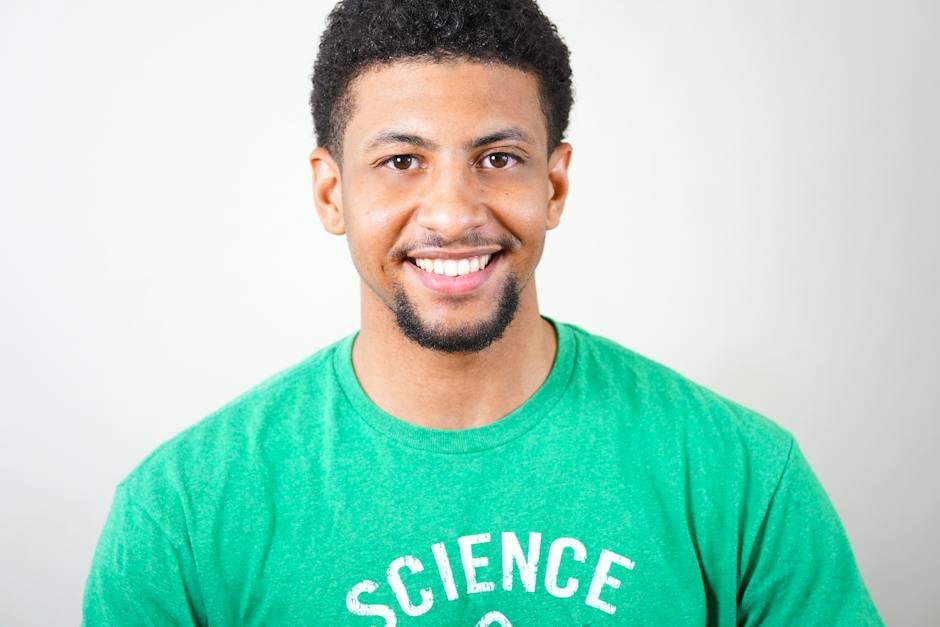
The narrative of Google Bard traverses a terrain lined with promise and uncertainty. Each stride forward underscores not just the marvels of current technological achievements, but also the sharp need for continuous innovation. Safeguarding ethical integrity and advancing the frontiers of language comprehension stand as colossal undertakings for the engineers and ethicists forging this path. It is through relentless pursuit of improvement that the limitations of today, from bias to misinterpretations, will serve as the guiding beacons towards a more enlightened AI future—a future where interactions with artificial intelligences like Bard are as seamless and enriching as those with our fellow humans.