In the rapidly evolving world of artificial intelligence, stumbling upon innovative, groundbreaking technologies such as GANs (Generative Adversarial Networks) and Dall-e is the new normal. However, these advancements often come accompanied by intricate concepts that pose challenges for most of us to grasp. As technology sinks its roots deeper into our lives, understanding these complex systems becomes vital. While GANs revolutionized machine learning with its unique duel-based architecture, Dall-e took a leap further, exhibiting an ability to generate high fidelity images from a mere textual description. This essay aims to demystify these groundbreaking technologies, unravel their workings, and provide a comprehensive comparison to equip you with an in-depth understanding of AI like never before.
Understanding GANs (Generative Adversarial Networks)
The world of artificial intelligence (AI) is boundless and intricate, offering new discoveries and technological breakthroughs—each more impressive than the last. One of the most potent tools in AI’s arsenal is the Generative Adversarial Network, or GAN. Since its inception, GANs have significantly influenced the AI landscape, propelling it towards uncharted territories of innovation. So, let’s delve into the fascinating world of GANs, unraveling these ingenious structures’ mechanics along the way.
GANs function following a unique principle, embodied in a simple analogy—you may think of it as an art forger (the generator) pitted against a seasoned art detective (the discriminator). The forger continually tries to create impeccable replicas of priceless artworks, while the detective scrutinizes these replicas, distinguishing the genuine from counterfeit.
The generator is suitably fit with random noise as input and coaxes it into reproducing real data, such as images or speech. At each step, this synthesizer enhances the “forged” data’s authenticity. Simultaneously, the discriminator evaluates these synthesised data against actual instances, classifying them as ‘real’ or ‘fake.’ The process is iterative — the generator constantly endeavors to bamboozle the discriminator while the latter improves its detection skills.
Underneath these complex operations are two foundational principles: the Backpropagation and Minimax game theory. Backpropagation propels the learning of both the generator and discriminator by adjusting their parameters, ensuring they get better over time. Minimax game theory, on the other hand, emulates a zero-sum game whereby the generator and discriminator seek to outperform each other. The equilibrium is reached when the generator crafts impeccable replicas, and the discriminator can only guess, akin to a coin flip.
Since its introduction by Ian Goodfellow in 2014, GANs have profoundly impacted the AI field, serving as a cornerstone in multiple applications. The amalgamation of creativity and pattern spotting that GANs represent has been indispensable in creating realistic human faces—those you might see in modern video games or simulate voice—echoing the revolution in speech synthesis.
GANs have also reshaped the narrative in data augmentation, creating diverse data from limited instances. In addition, they help simulate potential scenarios in climate modeling, financial forecasting, and even healthcare.
In the end, one does not need a PhD to appreciate the fascinating workings and immense potential of GANs. They stand as a true epitome of artificial intelligence – embodying the field’s potential to bear revolutionary fruits and influence numerous aspects of human existence. Through continuous research and spirited exploration, these marvels of machine learning will undoubtedly unravel new prospects — painting an even more vivid picture of the future.
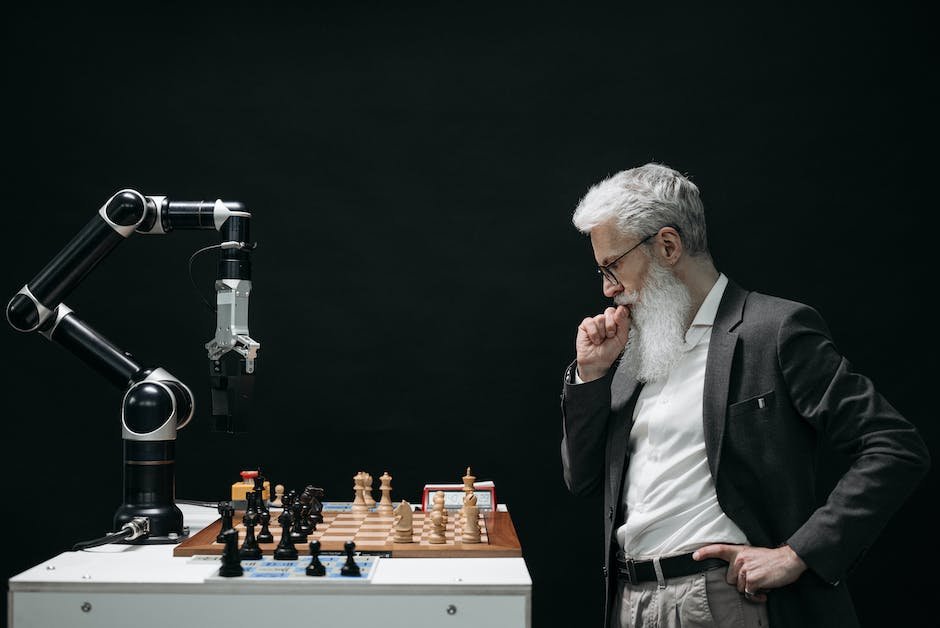
Introduction to Dall-e
Dall-e is a distinct marvel in the world of artificial intelligence developed by OpenAI, a research organization dedicated to sharing public goods beneficial for humanity. It is a unique application of GANs (Generative Adversarial Networks) the conceptual tool briefly discussed in the preceding discourse.
Named in admiration of the surrealist artist Salvador Dali and the Pixar movie character Wall-E, Dall-e is designed to generate images from textual descriptions, a task that elegantly interconnects the world of human language and visual perception. Unlike traditional GANs which solely generate images, Dall-e adds an intriguing layer of complexity by integrating the interpretation of textual inputs into its function.
Unveiled in January 2021, Dall-e leverages the algorithm of GPT-3, the third iteration of the Generative Pretrained Transformer, a machine learning model known for its outstanding capacity in understanding and generating human-like text. The exceptional feature in Dall-e resides in its power to interlock visual objects in a setting not previously seen together, thus creating an entirely new image with creative associations, a task that climaxes human creativity and machine learning dexterity.
Take for instance, the unusual request for an image of an “armchair in the shape of an avocado”. Dall-e crunches this text input with remarkable precision and creativity, producing a variety of avocado-shaped armchairs, a sight to behold.
In contrast to other AI models focused on algorithmic precision and numeric problem-solving, Dall-e illumines the potential for a nuanced artistic alliance between human creativity and machine output. It projects a future where AI could participate actively in creative processes, becoming explorative partners in areas such as design, art, and visual content production.
Furthermore, unlike conventional AI which analyzes existing data and makes predictions, Dall-e goes a step further to create. It breaks the barriers of what exists to what can be imagined, revolutionizing our understanding of AI capabilities.
On the flipside, Dall-e’s unprecedented capability births considerable ethical considerations. The potential for creative misuse, issues surrounding intellectual property rights, and the blurring line between human and machine-generated art are significant points to ponder.
Nevertheless, Dall-e stands in a unique position at the intersection of AI and creative prowess, defying prior limitations of machine learning. It’s a seminal demonstration of how future AI can push the boundaries of conventional applications, entering territories thought exclusive to human ingenuity.
This thrilling advancement in the study of artificial intelligence promises an exhilarating era ahead, where the critical line distinguishing human creativity from artificial intelligence becomes increasingly blurred. And so, the journey into uncharted territories of AI continues, with the humble hope of beneficial advancements and curious anticipation of the shape of things to come.
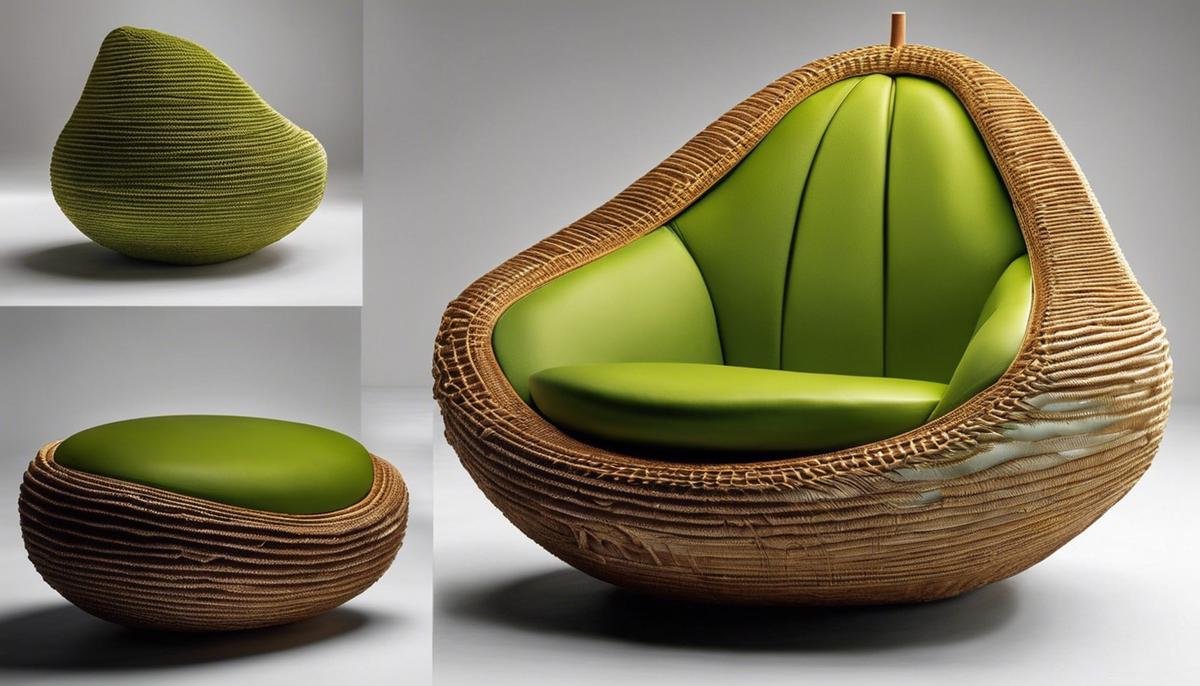
Comparing GANs and Dall-e
Moving on from the comprehensive understanding of GANs, let’s dive into the world of Dall-e. This AI model emerged as a fascinating tool well-disposed to the art of creative generation. Named after the famous surrealist painter Salvador Dali and Pixar’s animated film, WALL-E, Dall-e is both cleverly contrived and delightfully creative. The inspiration for this innovative model stems from the pressing need to explore the boundaries of artificial intelligence’s creative capacity.
While GANs are central to the operation of Dall-e, the latter carries a very specific purpose – generating highly unique images from textual descriptions. This intentional design is a stark divergence from earlier models that primarily focus on duplicating the real. Dall-e is built for the intrinsically difficult task of bridging the divide between text and image.
A standout feature of Dall-e is its integration of textual inputs into its function. This relies on a transformer-based language model known as GPT-3, a remarkable algorithm valued for its robustness and bidirectional capabilities. Leveraging this powerhouse, Dall-e is able to weave together visual elements and build newer image forms that are at once engaging and unheard of.
The illustrations birthed as a result of Dall-e’s creative operation are a testament to its uniqueness. From a multitude of armchairs shaped like avocados to oddball creatures never seen before, Dall-e illustrates tenuity and flair. It stands as manifest evidence of the potential residing in AI’s contribution to the creative processes.
This is not to suggest that Dall-e and conventional AI models are polar opposites. Instead, it’s more accurate to perceive Dall-e as an evolution, capitalizing on the foundational intricacies of AI and machine learning, and taking a step further into the realm of creativity.
As we revel in the brilliance of Dall-e and its capabilities, it’s necessary to glance at the ethical landscape it navigates. Concerns of potential misuse, copyright issues, and the likes are very real. Grappling with these considerations requires a fine balance between technological progress and ethical responsibility.
Though GANs and Dall-e may stand on slightly different grounds, they converge at the intersection of art and technology. Together, they exemplify the fusion of creativity and coding, undermining the long-held belief that machine learning is rigid and uncreative. Today, we’re experiencing the gradual blurring of lines between human artistry and machine creativity.
Despite both models having evolved in their own ways and having their own unique attributes, GANs and Dall-e cannot be easily pitted against each other in regards to superiority due to the differences in their primary objectives. While GANs are concerned with duplicating reality, Dall-e is designed for creative generation.
The advancements we’re witnessing with the influx of sophisticated AI tools like GANs and Dall-e are unprecedented. They foster not just deep excitement for what AI has accomplished so far, but also an eager anticipation for its future. The promise of endless possibilities serves as a reminder that we’re just dipping our toes into the vast ocean of what AI can do. It keeps us curious, engaged, and constantly questioning the amplitude of human imagination and machine intelligence.
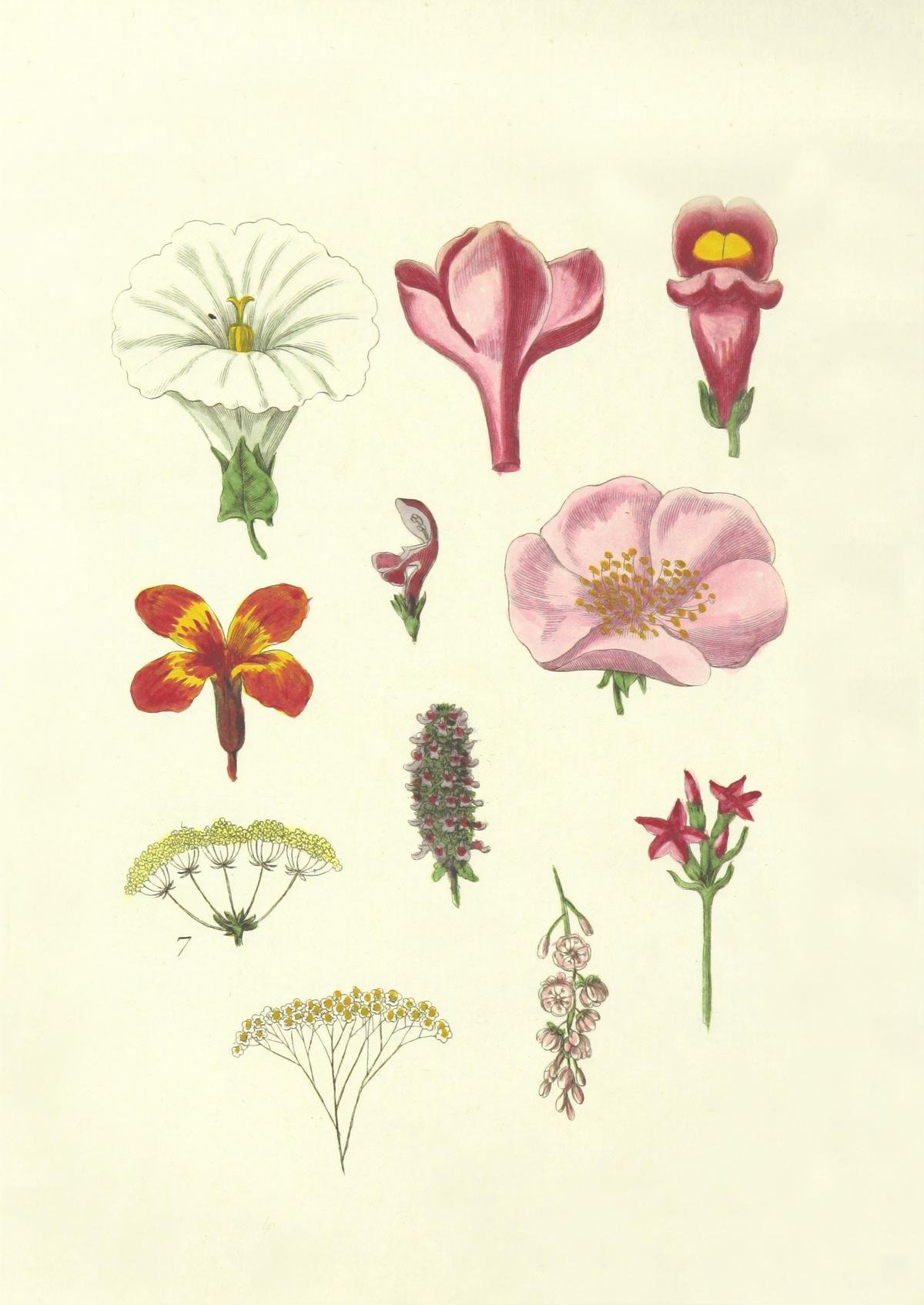
Photo by britishlibrary on Unsplash
Future Implications and Developments
The remarkable stride in the field of artificial intelligence (AI) continues to astonish us with its capabilities, responding to the ceaseless human endeavors to push boundaries into the unknown.
The implementation of Generative Adversarial Networks (GANs) has already caused a paradigm shift in how we perceive the world around us. Now, the emergence of Dall-e, a unique AI model, has projected a new vision for the future of creativity and design.
Dall-e, unlike the models traditionally based on GANs, focuses on generating unique, custom images from textual prompts. Drawing inspiration from the celebrated surreal artist Salvador Dali and the beloved Pixar character Wall-e, Dall-e’s name encapsulates the fusion of art and technology in its very core.
Its core function is to construct images from textual descriptions. Be it a text describing a two-story pink house shaped like a shoe or a sci-fi depiction of a futuristic city, Dall-e brings them alive in image form.
Integral to Dall-e’s operation is the seminal GPT-3 algorithm, which excels at comprehending and generating human-like text. It substantiates Dall-e’s ability to understand and recraft textual inputs into consequent visuals. Dall-e intersects textural understanding with visual elements, manifesting its capacity for creative output. This variegates the arsenal of AI, allowing it to participate in creative endeavors in ways previously unimaginable.
Notwithstanding its innovative capabilities, a keen eye must be had for the ethical implications Dall-e brings to the forefront. The fine line between original artwork and machine-generated content is getting fuzzy. As fascinating as it may be from a technological standpoint, the potential misuse of such technology for copyright infringement and other malevolent purposes should not be neglected.
The inception of Dall-e in the world of AI models has cemented its position at the intersection of creativity and technology. Traditional AI models and GANs primarily focused on data analysis and interpretation, while Dall-e, born of the same lineage, has demonstrated a distinctive capability towards creativity and design.
As with any frontier technology, various limitations are expected to be addressed going forward. However, what Dall-e has already achieved serves to expand the horizons of what machine learning can accomplish. It gives a hint of an AI-centric future where technology doesn’t just imitate creativity, but aids it, pushing the boundary and unleashing a new realm of possibilities.
The emergence of technologies like GANs and Dall-e not only redefines the scope of AI capabilities but opens opportunities for astonishing progress due in the not-so-distant future. The anticipation of what we can and should expect from AI is heightened, and with each advance, we come a step closer to envisioning a future where AI is not merely a tool but an integral component of our day-to-day life.
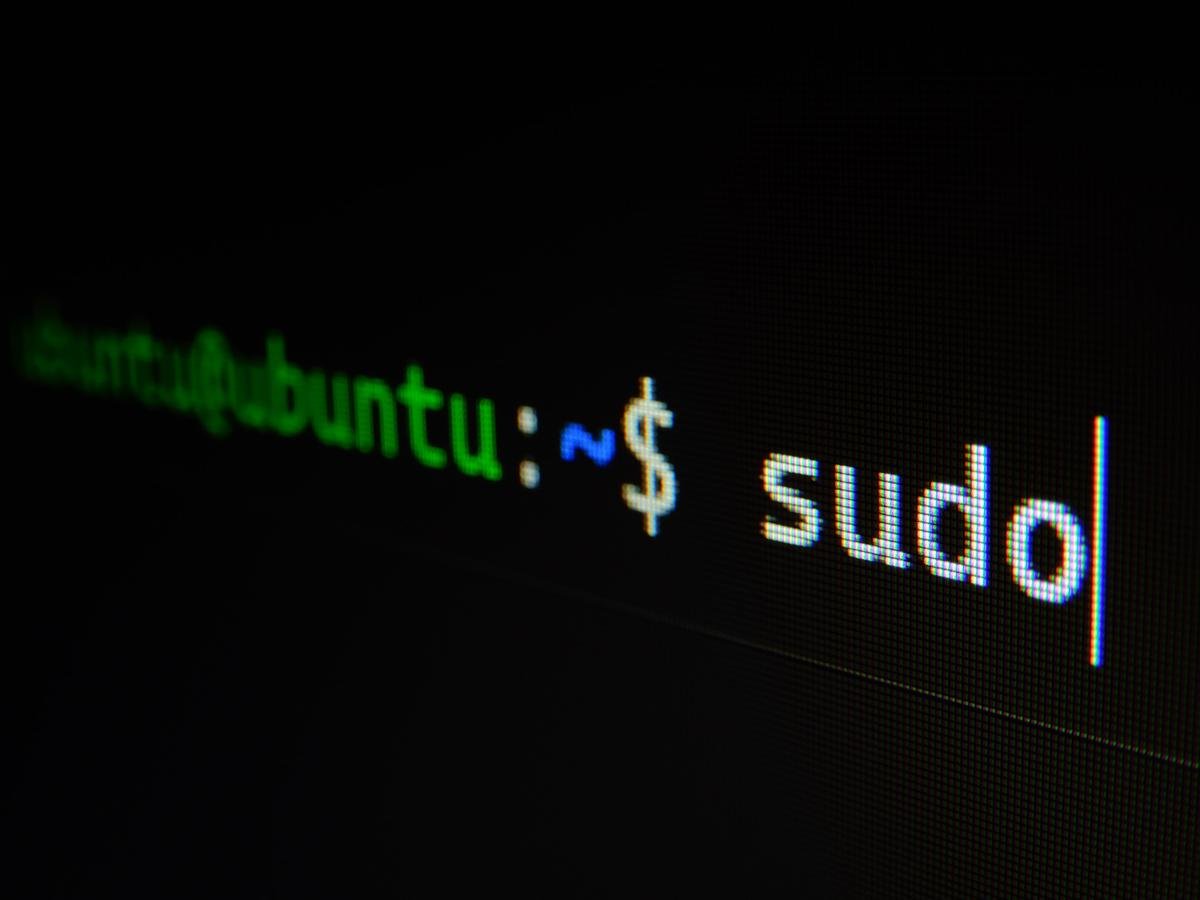
The fascinating exploration of GANs and Dall-e unravels a future where artificial intelligence evolves to become a crucial part of our daily existence, shaping industries and altering trends. It promises a landscape where breakthroughs occur at an unprecedented pace, massively impacting the realms of policy-making, ethical considerations, and AI education. Not merely confined to the world of technology, these AI models, with their strengths and weaknesses, are set to transform our perspectives, encouraging us to imagine an exciting yet challenging future that lies ahead of us. Thus, understanding them not only enriches our knowledge but also prepares us for a future that emerges, one AI innovation at a time.