ClimateAI’s Core Technology
ClimateAI integrates proprietary machine learning and AI modeling techniques, including physics-driven AI, to predict weather with high precision. Physics-driven AI processes vast amounts of climate data through intelligent algorithms that learn over time, assimilating datasets ranging from historical weather patterns to real-time climate variables.
ClimateAI also utilizes hybrid ML techniques that combine statistical methods and machine learning advancements to parse intricate climate scenarios and deliver nuanced forecasts. The models are trained with data from climate research institutes and outputs from ensemble models, ensuring predictive reliability.
Instead of relying solely on historical data, which may fall short in predicting new climate patterns influenced by global warming, ClimateAI’s tools dynamically adjust. They account for ongoing environmental shifts by recalibrating model inputs and weights in real-time.
Additionally, ‘foundation models’ add sophistication by generalizing across different applications without extensive reconfiguration. By training these models on mass weather and climate datasets, ClimateAI can customize outputs for various uses, from agricultural planning to emergency weather response strategies.
As science unravels the intricacies of climate variability, ClimateAI’s tools become increasingly important for industries affected by weather disruptions. The blend of AI sophistication and practical utility sets a higher bar for climate resilience, yielding insights that inform and empower actionable strategies in real-time scenarios.
Data Sources and Integration
ClimateAI incorporates data inputs from sources such as:
- European Centre for Medium-Range Weather Forecasts (ECMWF)
- Climate Hazards Group InfraRed Precipitation with Station data (CHIRPS)
- Global Historical Climatology Network daily (GHCNd)
The ECMWF provides atmospheric and oceanic datasets, CHIRPS introduces rainfall and temperature data, and GHCNd offers daily weather observations.
Integrating these diverse sources involves careful preprocessing. Anomalies and outliers are detected and rectified or excluded, ensuring accurate and representative model inputs. Data normalization aligns varying measures and scales across datasets, providing a uniform structure for machine learning processing.
Feature extraction then customizes the datasets into usable insights. Key patterns and indicators, such as temperature trends, humidity fluctuations, and atmospheric pressure changes, are distilled from the raw data and serve as critical variables for the predictive models.
By combining rigorous data integration techniques with cutting-edge AI, ClimateAI delivers weather and climate predictions that are forward-looking and continuously evolving with each new data input. This dynamic updating mechanism ensures the models remain relevant and authoritative, reflecting the latest climatic conditions.
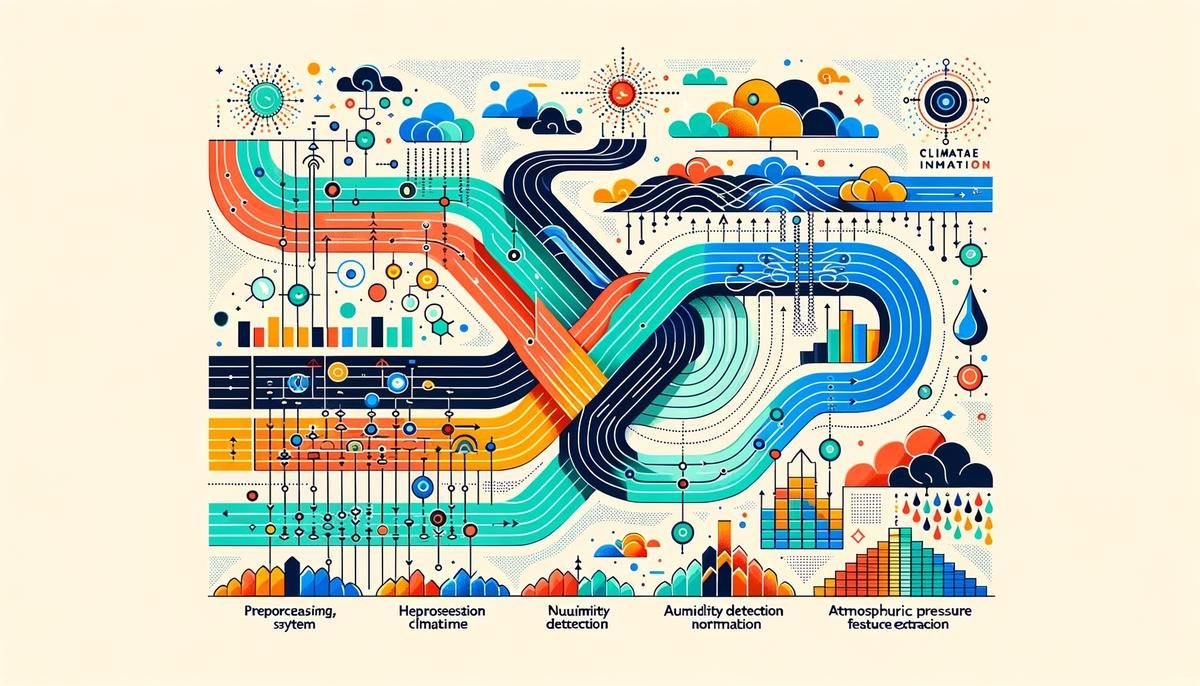
Impact on Different Sectors
Accurate weather forecasting by ClimateAI brings advantages to various sectors, including agriculture, retail, and energy.
Agriculture
In agriculture, refined weather models allow farmers and agribusinesses to plan more effectively. Knowing the likelihood of early frost or drought conditions enables better crop and water resource management, optimizing yields and contributing to sustainable farming practices.
Retail
The retail sector benefits from precise climate forecasting by adjusting inventory in anticipation of weather-related buying trends. Accurate predictions help align product availability with consumer demand and optimize shipping routes and schedules.
Energy
The energy sector, particularly renewable energy, utilizes ClimateAI’s predictions to maximize output and efficiency. Accurately forecasting sun and wind patterns allows energy companies to anticipate power production levels and identify potential disruptions, ensuring a steady supply and increased grid reliability.
The integration of ClimateAI’s predictive analytics extends into long-term planning within these sectors. In agriculture, long-range forecasts can determine the viability of cultivating particular crops in specific regions. For retail businesses, understanding seasonal trends and climate shifts can inform store location choices and marketing strategies. In energy, long-term climate trends are pivotal for positioning renewable energy infrastructure efficiently.
These adaptation strategies illustrate how companies leverage AI-driven weather and climate forecasting for a competitive edge, allowing industries to respond to and anticipate changes in an increasingly unpredictable climate landscape.
Risk Intelligence in Climate Adaptation
Risk intelligence plays a central role in adapting and mitigating the detrimental impacts of climate variability and extreme weather events. It involves anticipation, preparedness, and response strategies to efficiently address potential climate-related risks.
In infrastructure development, urban planners and engineers utilize risk intelligence from ClimateAI’s weather forecasts and climate scenario modeling to design resilient structures that can withstand severe climate events. This mitigates foreseeable hazards, ensuring durability and protecting investments and lives over extended periods.
Energy infrastructure development benefits from integrating risk intelligence into resource allocation and network design. ClimateAI predictive analytics guide the placement of power stations or grid layouts to circumvent areas susceptible to climate risks, securing uninterrupted power supply and optimizing repair and maintenance schedules.
In agriculture, ClimateAI enhances adaptability to volatile weather conditions and long-term climate changes. AI-driven predictive models allow agriculturalists to anticipate adverse weather patterns affecting crop yield, influencing decisions regarding planting schedules, irrigation practices, and crop selection.
On a broader scale, this intricate forecasting assists in managing agricultural input supplies proficiently. With predictable weather patterns and seasonal variations mapped out by AI, cost-effective resource management becomes feasible, reducing excess expenditure and enhancing sustainable farming practices.
The predictive insight from ClimateAI’s models builds the foundation for crop insurance and agricultural financing industries to adjust premiums and lending policies based on precise risk assessments. By lowering financial volatility due to weather uncertainties, the entire agricultural value chain benefits from enhanced stability.
The application of risk intelligence powered by ClimateAI’s forecasts in these fundamental sectors choreographs responses to immediate climate risks and underscores proactive planning for a sustainable future.
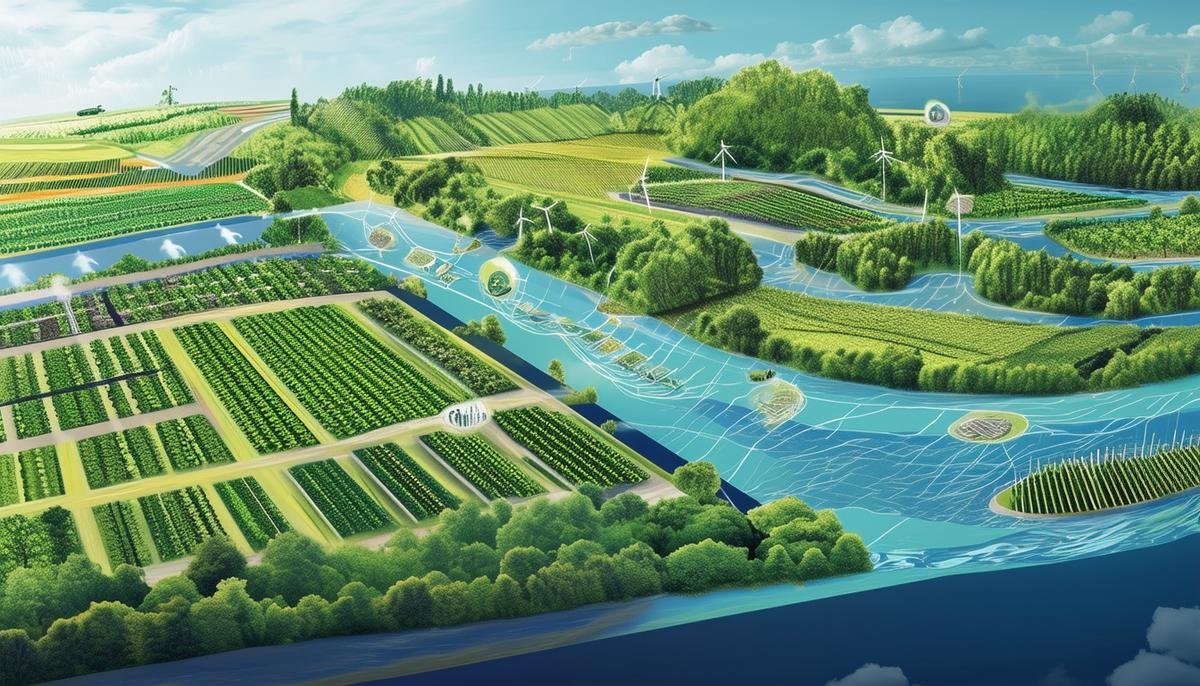
Real-world Applications and Case Studies
ClimateAI’s predictive technology has revolutionized agricultural operations, as showcased in various case studies.
Wheat Growers in Central Kansas
Wheat growers in central Kansas faced yield uncertainties due to unpredictable weather patterns. By leveraging ClimateAI’s hyper-localized climate predictions, farmers adjusted planting and harvesting schedules, leading to a 20% increase in crop yields within the first year of implementation.1 The economic impact was profound, reducing costs associated with wasted inputs and enabling bulk purchase discounts.
Premium Wine Producers in Napa Valley, California
In California’s Napa Valley, premium wine producers are sensitive to conditions affecting grape quality. ClimateAI provided micro-climate predictions that prevented harvests falling prey to unexpected mildew or frost. One vineyard modified its harvesting schedule based on AI predictions, avoiding an early onset of heavy dew and producing higher quality wine. This led to a 15% increase in revenue over the same fiscal period.2
Potato Farmers in Idaho
ClimateAI’s involvement in optimizing irrigation schedules is also noteworthy. A software pilot in Idaho used AI models to anticipate dry spells and heightened evaporation rates, aiding potato farmers struggling with water scarcity. The models accurately predicted local climate phenomena, optimizing irrigation periods to maximize water efficiency. This decreased water usage by 25%, reducing costs and improving compliance with conservation regulations.3
These real-world applications demonstrate how incorporating ClimateAI into agricultural practices transforms operational efficiency. The benefits include cost savings, environmentally sustainable practices, and improved yield quality, securing strong business advantages and sustainable sector futures.
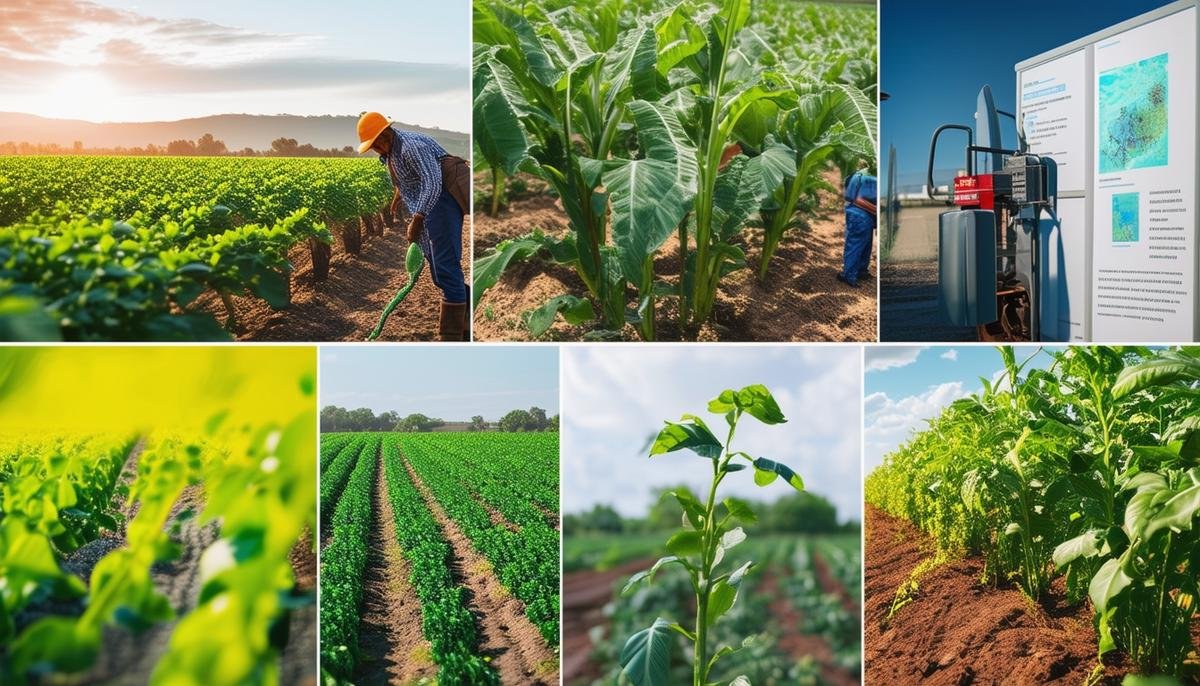
Future of AI in Climate Prediction
In the future, AI-driven climate prediction is expected to see significant advancements that improve accuracy and enable more integrated approaches to addressing climate vulnerability. This progress assumes that AI will consistently improve in areas such as decipherability, computational efficiency, and ethical alignment with environmental concerns.
One notable potential in enhancing AI meteorological models involves developing more sophisticated machine learning algorithms capable of superior self-correction and adaptation to new data. There will likely be a heightened focus on unsupervised learning protocols, where AI can discern patterns and make reliable predictions without human-labeled data. This improvement opens up scalability prospects for models that apply to previously unnoticed or undervalued climate factors.
As the volume of historical climate data grows and meteorological and satellite capturing technologies advance, the input quality for AI simulations will drastically improve. This increase in data quality enhances the learning depth of neural networks, enabling the generation of more detailed predictions on interdependencies and micro-climatic segments. Such advancements could potentially enhance planners’ abilities to address shifting patterns behind phenomena like El Niño cycles or rapid ice melts.
However, the path to advanced forecasts is complex, primarily due to the challenge of managing real-time data inflow while maintaining accuracy. This paradigm introduces operational and infrastructural costs linked to platform maintenance, which may exceed realistic budgeting forecasts for entities involved in climate research.
Alongside rising computational demands, there is an ethical consideration regarding the sustainability of AI’s own environmental impact. As climate science benefits from AI enhancements, it’s crucial to ensure that AI platforms don’t excessively contribute to carbon emissions or consume disproportionate resources. Future advancements must consider embedding energy-efficient processors and advocating for renewable energy use within server farms to address these ethical concerns effectively.
The opposition between AI’s energy requirements and its outputs may see potential resolution through advancements in AI energy efficiency and systemic changes across computational tasks. Forward pursuits could integrate hybrid models that blend classical physically driven forecasting techniques with robust machine learning models, offsetting intensive computations intrinsic to sole-use deep neural networks.
Progress in AI-driven climate prediction involves advancing scientific innovation, practical refinements in model applications, and responsibility towards ecological footprints. Enhanced predictive accuracy provides society with tools to better prepare for climate variability, contributing to the development of adaptable infrastructures, resilient agricultural practices, and conscientious energy utilization.
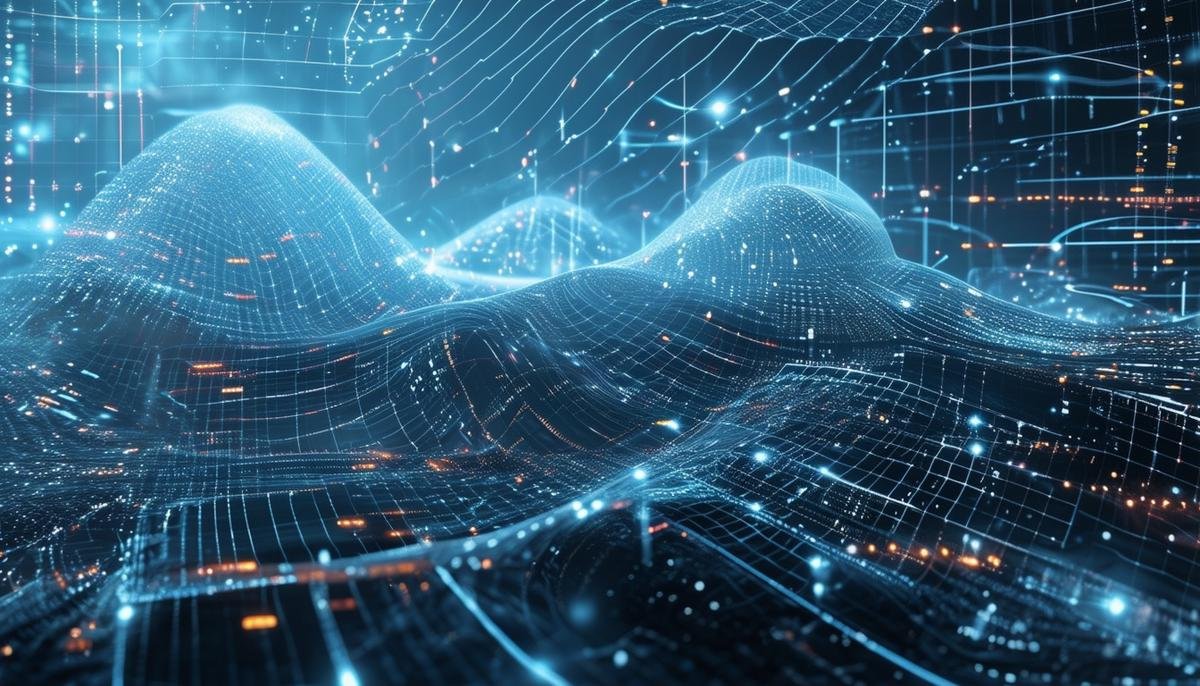
The impact of ClimateAI’s predictive technology goes beyond forecasts, providing industries with the tools necessary to adapt and thrive in an unpredictable climate landscape. This capability enhances operational efficiency and supports sustainable practices that are crucial for long-term resilience.
Some key benefits of ClimateAI’s technology include:
- Improved risk management: By providing more accurate and detailed climate predictions, businesses can better assess and mitigate potential risks associated with extreme weather events and changing climate patterns.
- Optimized resource allocation: With a clearer understanding of future climate conditions, industries can allocate resources more effectively, reducing waste and improving overall efficiency.
- Enhanced sustainability: By incorporating climate predictions into decision-making processes, businesses can adopt more environmentally friendly practices and contribute to global efforts to combat climate change.
The application of ClimateAI’s technology spans across various sectors, such as agriculture, energy, and infrastructure. For example, in the agricultural industry, farmers can use AI-driven insights to optimize crop selection, irrigation schedules, and pest management strategies based on predicted weather patterns1. This not only improves crop yields but also promotes the efficient use of water and other resources.
As the world continues to grapple with the challenges posed by climate change, the role of AI in climate prediction and adaptation will become increasingly critical. ClimateAI’s cutting-edge technology is at the forefront of this movement, empowering businesses and communities to build a more resilient and sustainable future.
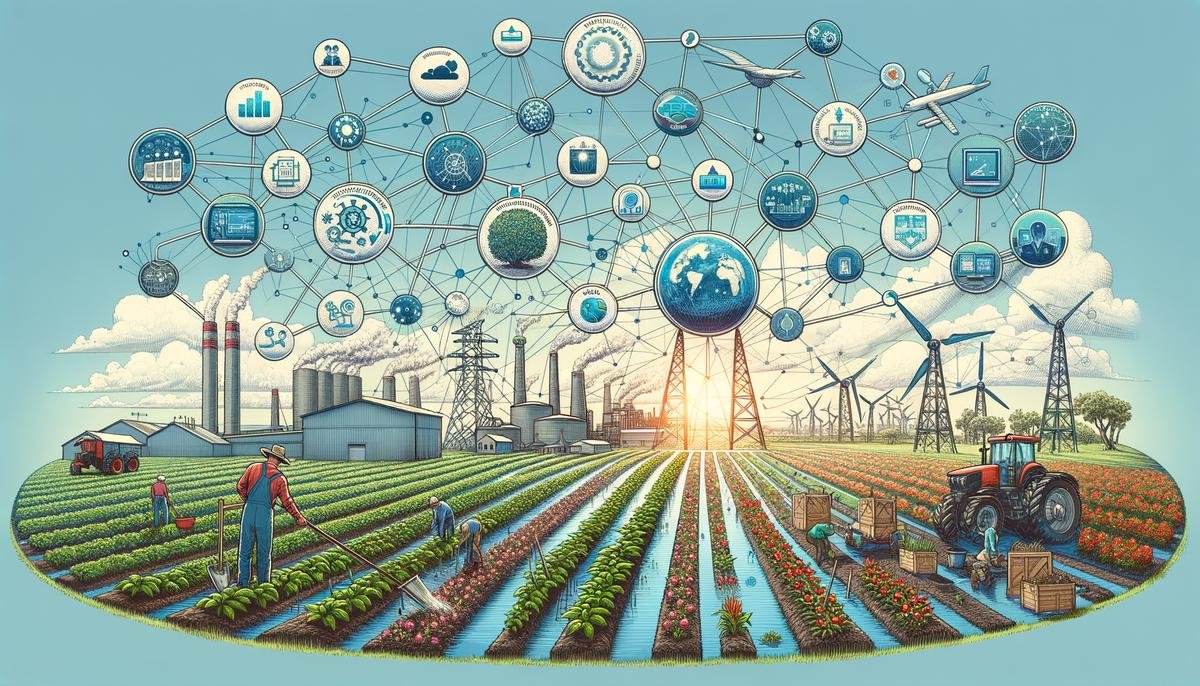