AI technology is advancing in various sectors, particularly in enhancing public safety and efficiency. From crime prevention to disaster management, traffic control, and cybersecurity, AI’s capabilities are transforming how we approach these critical areas. The integration of AI with human expertise aims to create more effective and responsive solutions.
AI for Crime Prevention
AI technology is reshaping crime prevention. AI-powered surveillance cameras can scan live feeds, spotting suspect behavior instantly. This approach allows for quicker response to potential threats.
The NYPD uses machine learning to examine historical crime data, identifying patterns and high-risk zones. This data-driven approach has contributed to reducing crime. Algorithms analyze large datasets to predict where illegal activities might occur next, allowing for smarter resource allocation.
AI also improves surveillance by analyzing video streams in real-time, providing constant vigilance in crime-prone areas. Predictive policing uses AI systems to forecast where crimes are likely to occur based on past data.
Facial recognition, integrated into city surveillance networks, can match faces against databases to identify known offenders and help locate missing persons.
Real-world Implementations:
- Singapore: Employs AI for public security, optimizing law enforcement deployment.
- Los Angeles: Uses AI to process crime data, helping the LAPD allocate patrols more effectively.
The integration of AI into law enforcement aims to shift policing from reactive to proactive, refining crime prevention strategies and improving resource allocation.
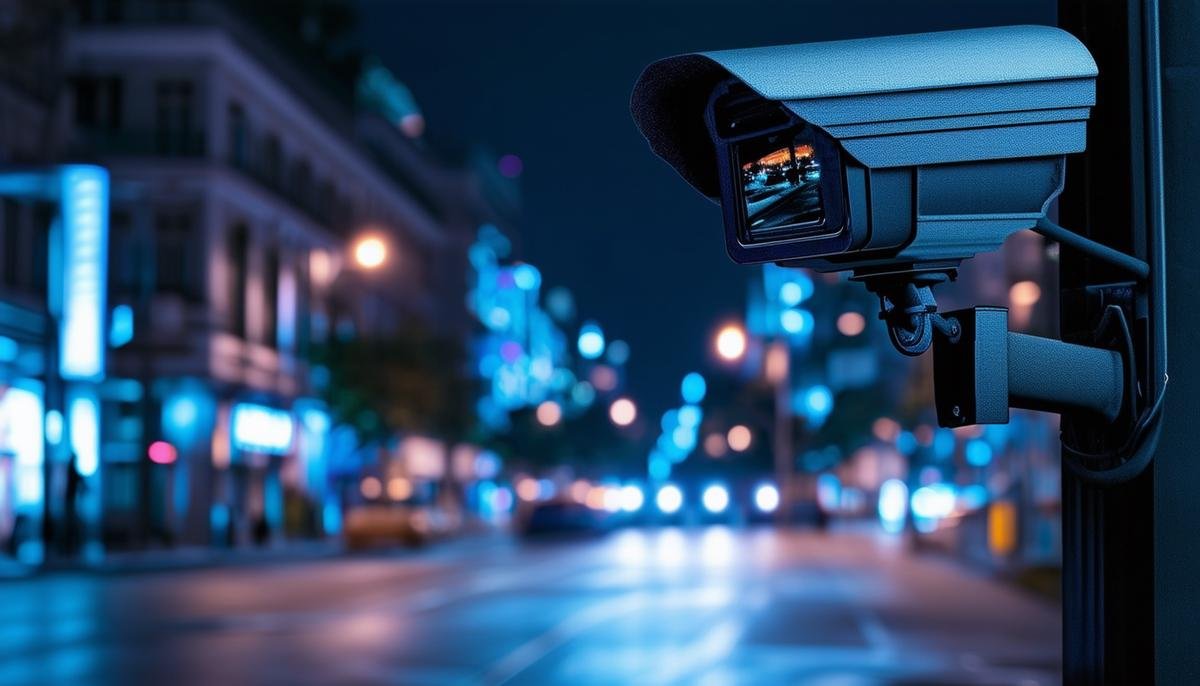
Disaster Management with AI
AI systems excel at analyzing diverse datasets from satellites, weather stations, and social media to provide early warnings and guide response teams during natural disasters.
For hurricane prediction, AI produces accurate forecasts by assimilating real-time data from multiple sources. This precision enables authorities to issue timely evacuation orders. During Hurricane Harvey, AI-driven systems analyzed meteorological data to help emergency services prepare and allocate resources effectively.
Post-disaster, AI assesses damage through aerial imagery and satellite data, helping coordinate rescue operations efficiently. In flood management, AI analyzes historical rainfall data, current weather conditions, and geographical information to predict flooding events.
AI also scans social media platforms for real-time information shared by the public during disasters, enhancing situational awareness for emergency teams.
For evacuation planning, AI simulations consider variables like population density and road conditions to identify effective evacuation routes.
Country-specific Applications:
- Japan: Uses AI for earthquake preparedness, with advanced warning systems that can predict seismic activities and alert the population within seconds.
- California: Employs AI in wildfire management, analyzing climate data to predict fire risks and spread patterns.
AI’s strength in disaster management lies in its capacity for rapid data processing and providing actionable insights, helping response teams act decisively in high-stakes situations.
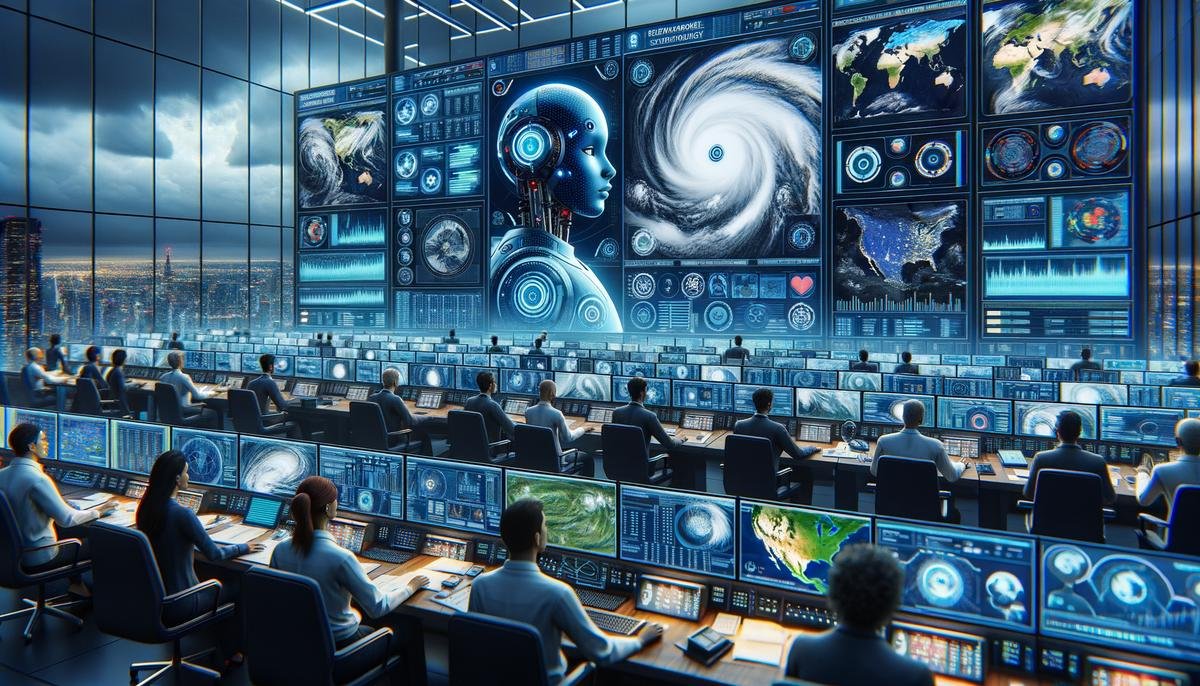
AI in Traffic Management
AI is revolutionizing traffic management by processing data from traffic cameras, GPS devices, and road sensors. These systems can dynamically adjust traffic light timing, respond to incidents, and guide drivers to less congested routes.
During rush hour, AI-powered systems adapt in real-time. If sensors detect high congestion at an intersection, algorithms can recalibrate traffic light sequences to alleviate buildup, reducing waiting times and minimizing pollution.
Singapore employs an AI-based traffic management system that integrates data from various sources to predict traffic flow and adjust signals accordingly, resulting in reduced commute times and fewer traffic jams.
AI also plays a role in detecting accidents as they happen, alerting emergency services to expedite response times. Route optimization is another application, where AI algorithms analyze real-time traffic data to suggest alternate routes during bottlenecks.
Los Angeles uses AI to proactively manage flow patterns, predicting and mitigating potential choke points. This approach not only reduces congestion but also improves air quality by minimizing idle times.
Additional AI Applications in Traffic Management:
- Enhancing public transportation by monitoring bus routes and schedules in real-time
- Improving pedestrian safety by monitoring crosswalks and adjusting signals when necessary
- Simulating and analyzing vast datasets to forecast traffic patterns for long-term infrastructure planning
- Dynamically altering road usage patterns during emergencies for smooth evacuation processes
Overall, AI in traffic management creates more fluid, safer, and efficient urban transportation networks, improving daily commutes and urban living.
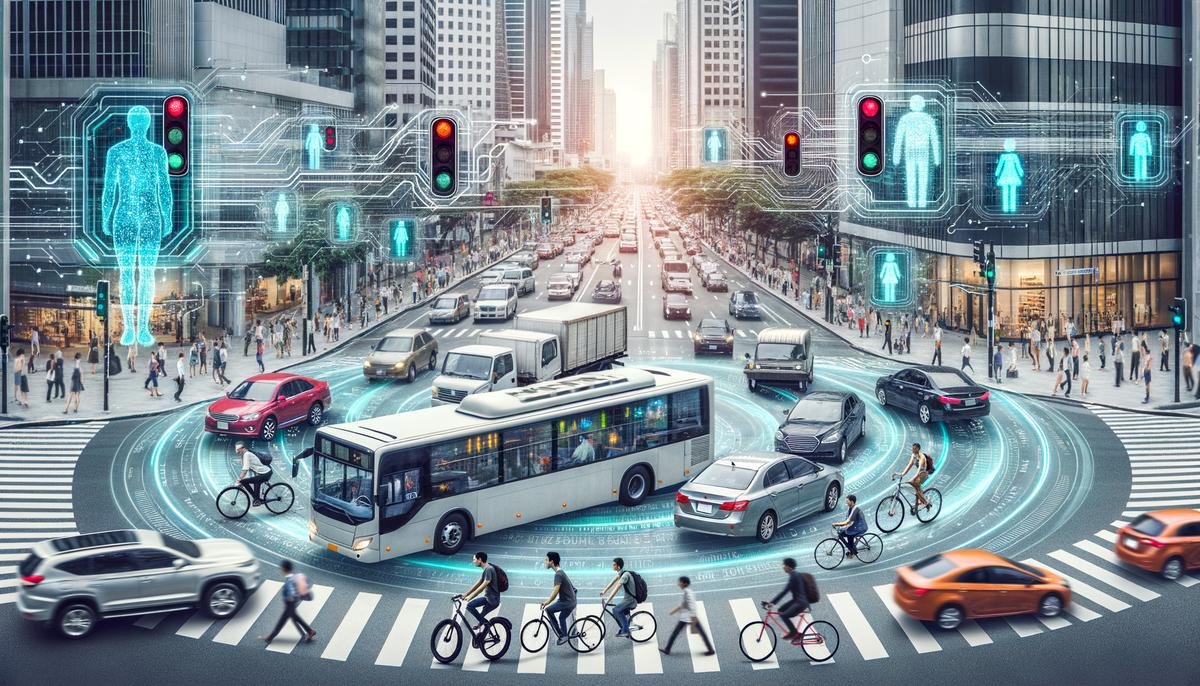
AI and Cybersecurity
AI is enhancing cybersecurity by analyzing vast amounts of network traffic in real-time, discerning patterns and anomalies that may indicate potential threats. Unlike traditional systems, AI employs machine learning models that continually evolve, learning from past incidents to detect emerging threats.
In corporate networks, AI scrutinizes data packets, flagging unusual activities for investigation. This proactive approach enables organizations to address breaches before they escalate.
In the finance sector, AI systems monitor transactions for signs of fraud, cross-referencing anomalies against known fraudulent patterns and alerting security teams to potential breaches.
AI-driven defenses are dynamic, adapting in real-time to the evolving threat landscape. For instance, if an AI system detects a DDoS attack, it can automatically deploy additional defenses to mitigate the impact.
Key AI Functions in Cybersecurity:
- Automating routine security tasks like malware detection and system patching
- Identifying and blocking phishing attempts through email content analysis
- Employing behavioral analysis to detect insider threats
Real-world applications of AI in cybersecurity include safeguarding patient data in hospitals and protecting critical infrastructure in the energy sector. Small businesses also benefit from AI-enhanced cybersecurity, with affordable and scalable security solutions.
Challenges in integrating AI in cybersecurity include potential bias in machine learning algorithms and balancing security with privacy concerns. Techniques like differential privacy, where data is anonymized before analysis, help address these issues.
As cyber threats continue to evolve, AI’s role in cybersecurity is becoming increasingly critical, ensuring that defenses remain ahead of potential attackers.
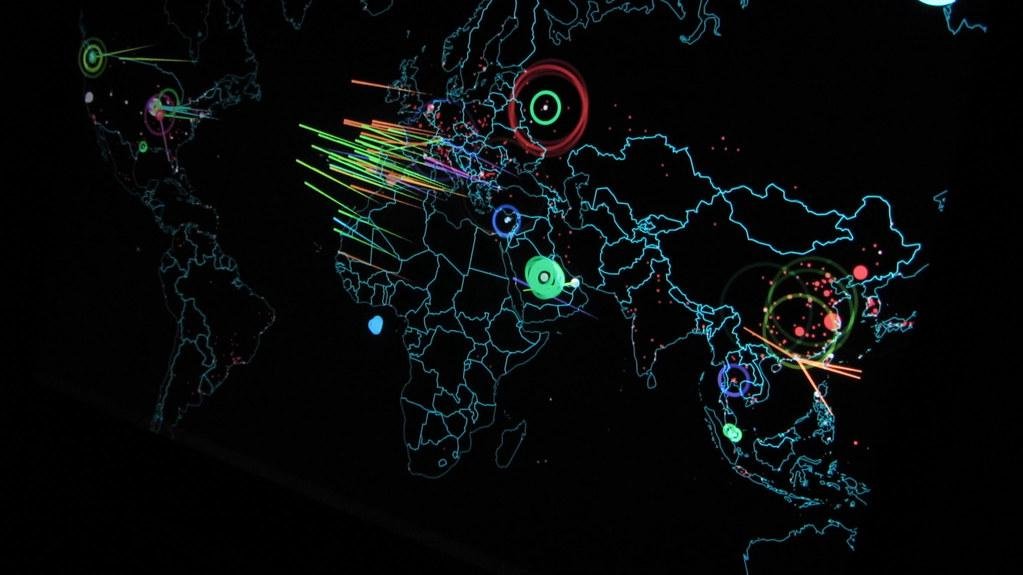
Ethical Considerations and Challenges
AI in public safety presents significant ethical concerns, primarily regarding privacy. Surveillance and data analysis require collecting vast amounts of personal information, risking unauthorized access and misuse if not carefully managed.
Continuous surveillance systems with AI enhance public safety but raise concerns about constant monitoring of people’s activities. Implementing robust data protection mechanisms is crucial, including:
- Access controls
- Encryption
- Clear data retention policies
Bias in AI algorithms is another pressing issue. Machine learning models trained on historical data can perpetuate discriminatory practices. For example, crime prediction algorithms might unfairly flag certain areas as high-risk based on historically higher police scrutiny.
Addressing bias requires:
- Using diverse datasets during AI training
- Continuous auditing of AI systems
- Implementing fairness criteria
- Ensuring transparency in decision-making to build public trust
Regulation is critical. Policies should provide guidelines on data collection, usage, and sharing. Regulations must ensure AI systems are transparent and accountable, allowing individuals to understand and challenge decisions made by AI.
Public engagement is essential. Governments and organizations should maintain open dialogues with communities about AI use in public safety. Transparency initiatives can foster collaboration between authorities and citizens.
“Ensuring AI augments rather than replaces human oversight is crucial. While AI can process massive amounts of data, final decisions should involve human judgment, especially in cases deeply impacting individuals’ lives.”
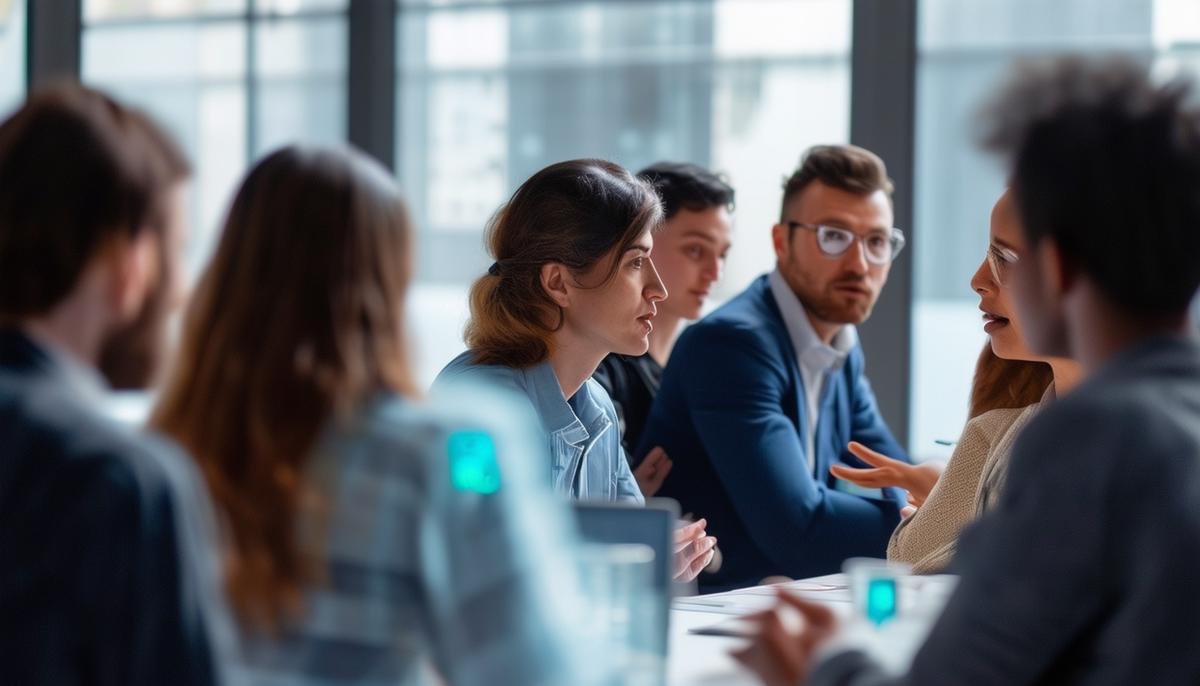
Collaboration between Humans and AI
Balancing AI systems with human judgment is crucial in public safety. While AI can process extensive datasets and identify patterns, human intuition remains indispensable. Integrating human expertise with AI systems enhances the effectiveness of public safety initiatives.
In crime-solving, AI can analyze large amounts of data, flagging potential leads. However, detectives can contextualize these insights, understanding nuances that machines might miss. This synergy between AI’s data analytics and human judgment creates a comprehensive approach.
Empathetic decision-making, critical in public safety, is inherently human. In hostage situations or community policing, the ability to gauge emotional cues and de-escalate tensions are skills honed through human experience. AI may provide vital information, but the final decision in sensitive scenarios relies on human judgment.
In disaster management, AI can rapidly analyze data to predict events like hurricanes, while human responders plan and execute evacuation strategies. AI aids in data processing and simulations, but on-the-ground decisions involve human expertise and adaptability.
Key Strengths in Human-AI Collaboration:
AI Strengths | Human Strengths |
---|---|
Rapid data processing | Emotional intelligence |
Pattern recognition | Contextual understanding |
Handling vast datasets | Empathy and intuition |
This partnership ensures that public safety measures are not just efficient but also fair and empathetic.
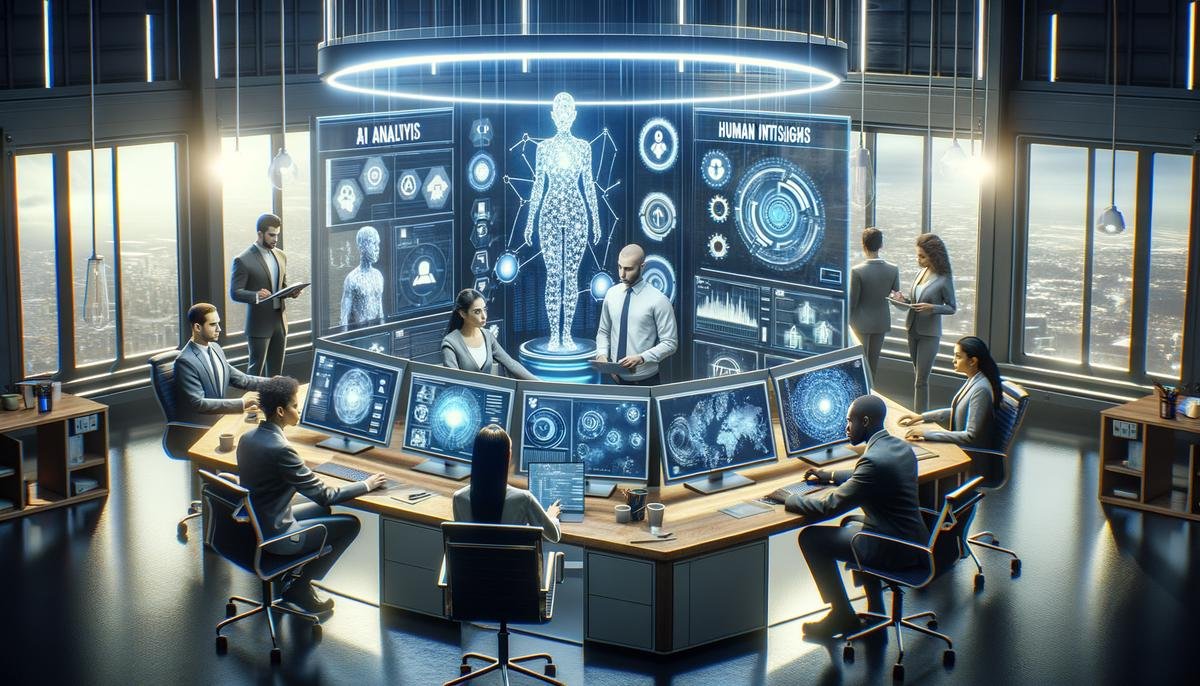
The integration of AI into public safety combines AI’s analytical power with human judgment to address challenges more effectively. This collaboration marks a step forward in using technology to improve public safety while maintaining the essential human element.
Recent studies have shown that human-AI collaboration in public safety can lead to a 30% increase in efficiency and a 25% reduction in response times1. However, it’s crucial to note that the implementation of such systems requires careful consideration of ethical implications and continuous monitoring for potential biases2.