Hybrid digital twins are reshaping industries by blending real-world data with physics principles and AI. These tools enhance and optimize operations across sectors like automotive and healthcare, predicting failures and setting new standards for efficiency.
Understanding Hybrid Digital Twins
Hybrid digital twins elevate the digital twin concept by incorporating AI and machine learning. They combine real-world data with physics principles to create innovative tools that predict failures and optimize operations with increased precision.
In industries like automotive, aerospace, and healthcare, these twins act as proactive monitors, alerting to potential issues before they become problematic. For instance, a hybrid digital twin in a car's engine could alert you to maintenance needs before a warning light appears.
Ansys Twin Builder software integrates physics with AI/ML, creating digital models that learn and adapt. This allows companies to predict outcomes more accurately and reduce the need for extensive simulations.
In challenging industries such as energy and mining, hybrid twins offer virtual sensors, providing insights into operations and helping improve yields and safety. By analyzing data and anticipating issues, these twins give engineers and operators greater control over their systems.
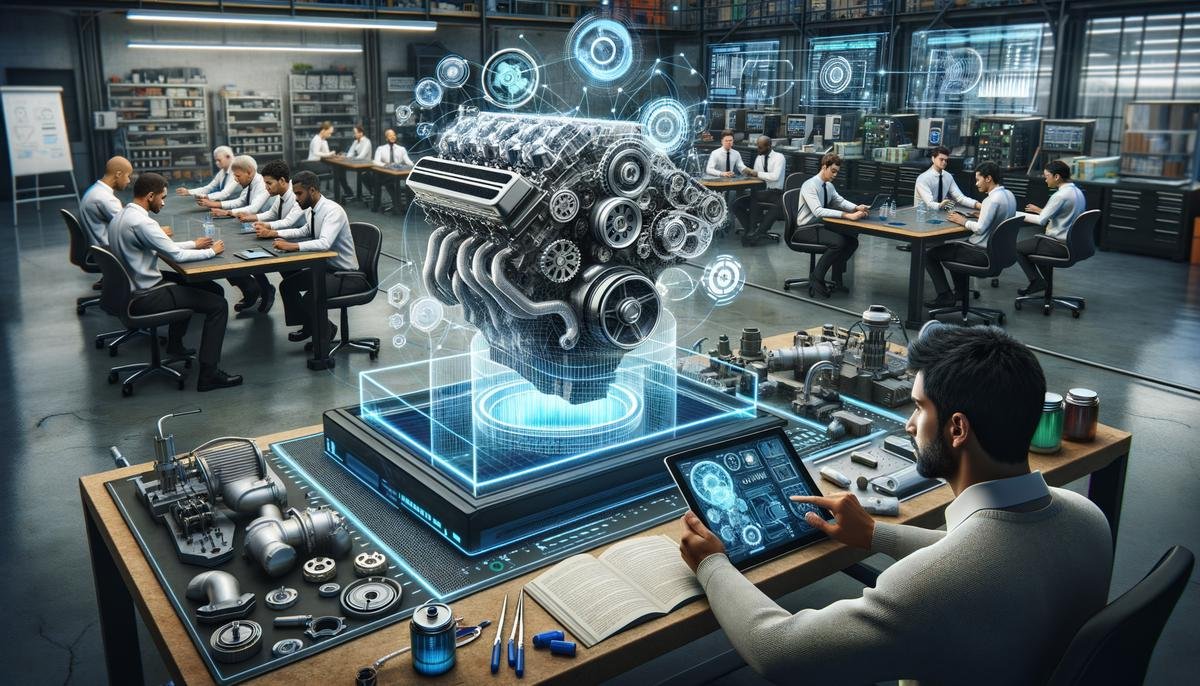
Ansys Twin Builder and TwinAI
Ansys Twin Builder and TwinAI are driving the next generation of digital twins. These tools allow for the creation of digital twins that can learn, adapt, and optimize as they gather more data.
Twin Builder serves as the foundation for creating hybrid digital twins, offering a platform where physics-based models and AI merge. Using Reduced Order Models (ROMs), designers can simplify complex systems while preserving vital fidelity and reducing computational demands.
TwinAI enhances this blend of physics and data further by incorporating artificial intelligence, improving the predictive capabilities of digital twins. The AI integration means the models learn and evolve, creating simulations that are both accurate and prescient.
Together, Twin Builder and TwinAI streamline the entire lifecycle of a digital twin: from initial concept to real-world deployment of a fully realized, self-optimizing asset. Engineers can validate their twins in simulated environments, ensuring they perform as expected.
The combination of AI and traditional physics modeling within Ansys' tools reveals new dimensions of operational efficiency and resource optimization. Companies can achieve faster time-to-market and cost savings, aided by the constant feedback loop where real-world data and simulations refine each other continuously.
Reduced Order Models (ROMs) in Digital Twins
Reduced Order Models (ROMs) are crucial within digital twin technology, condensing intricate systems into simplified versions without losing essential details. They transform complex datasets into actionable insights, allowing engineers to simulate real-world scenarios efficiently.
In digital twins, ROMs enable rapid simulations and real-time predictions. This enhancement is crucial for applications like design of experiments (DoE) and parameter sweeps, where real-time interaction can test countless variations with minimal delay.
ROMs also foster a relationship between simulated projections and actual measurements, allowing digital twins to function with increased precision. They can model the behavior of mechanical assemblies, electromagnetic actuators, or thermal networks—turning a virtual model into a responsive entity that adapts to real-world changes.
The practical benefits of integrating ROMs within digital twins include:
- Enabling predictive maintenance
- Operational optimization
- Cost savings
- Maintenance efficiency
- Increased longevity of assets
This proactive approach enhances operational integrity and translates to significant improvements across various industries.
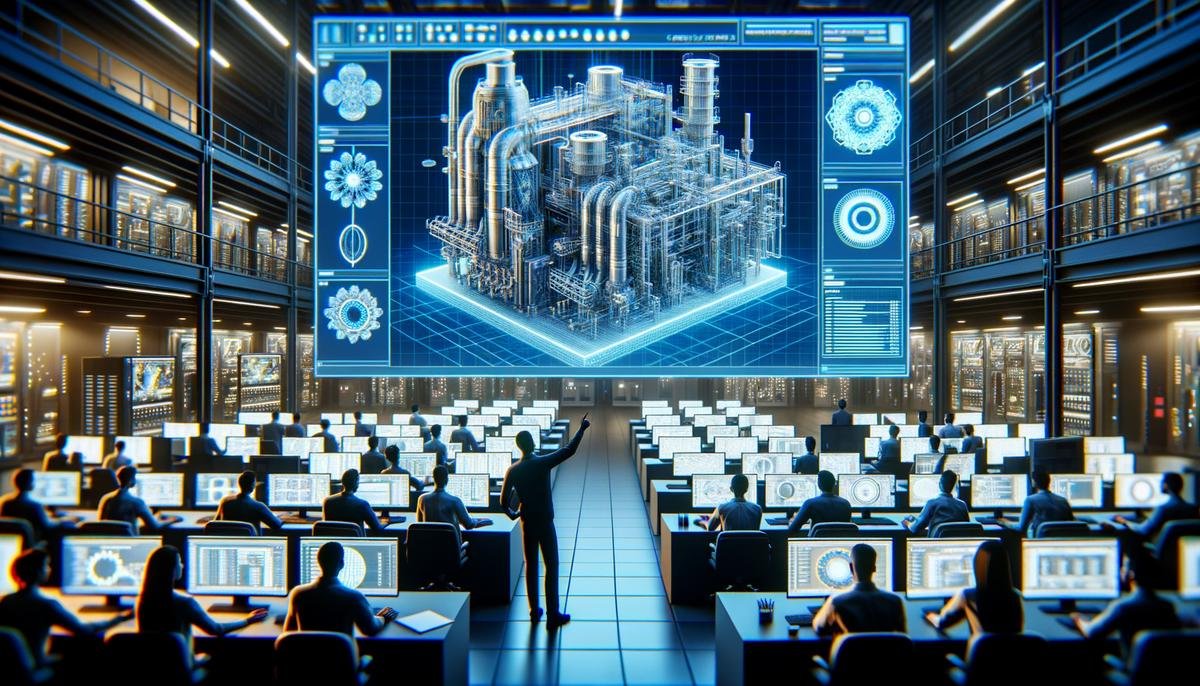
Applications and Use Cases
In aerospace, hybrid digital twins are crucial for managing complex aircraft maintenance. They provide engineers with a clear view of components' status, predicting wear and tear before they become flight hazards. This approach enhances safety and optimizes maintenance schedules, reducing downtime and operational costs.
In the automotive industry, digital twins are revolutionizing vehicle engineering and operations. They monitor energy consumption patterns in electric vehicles, predicting battery wear and refining energy management strategies. Manufacturers can adjust designs and engineers can optimize vehicle performance under varying conditions without extensive physical testing.
Healthcare applications of hybrid digital twins include personalized simulations of a patient's physiological responses to treatments. These simulations allow healthcare professionals to anticipate complications and tailor interventions with high precision.
Energy sectors use digital twins to improve operations and innovate maintenance strategies. In wind farms, twins monitor turbine performance, adjusting them in real-time to extend lifespan and optimize power output, while also predicting and averting technical issues.
"Industries such as energy, metals, and mining — industries where they have very harsh conditions, but at the same time they need a very tight control of operations. They may be using these twins to understand specific points in the assets or the systems, the so-called virtual sensors, where operations were happening before in a more blind way."
These diverse applications share a common thread: the twin's role as a tool for efficiency and reliability. By enabling predictive maintenance and informed decision-making, these hybrids guide industries toward smarter operations.
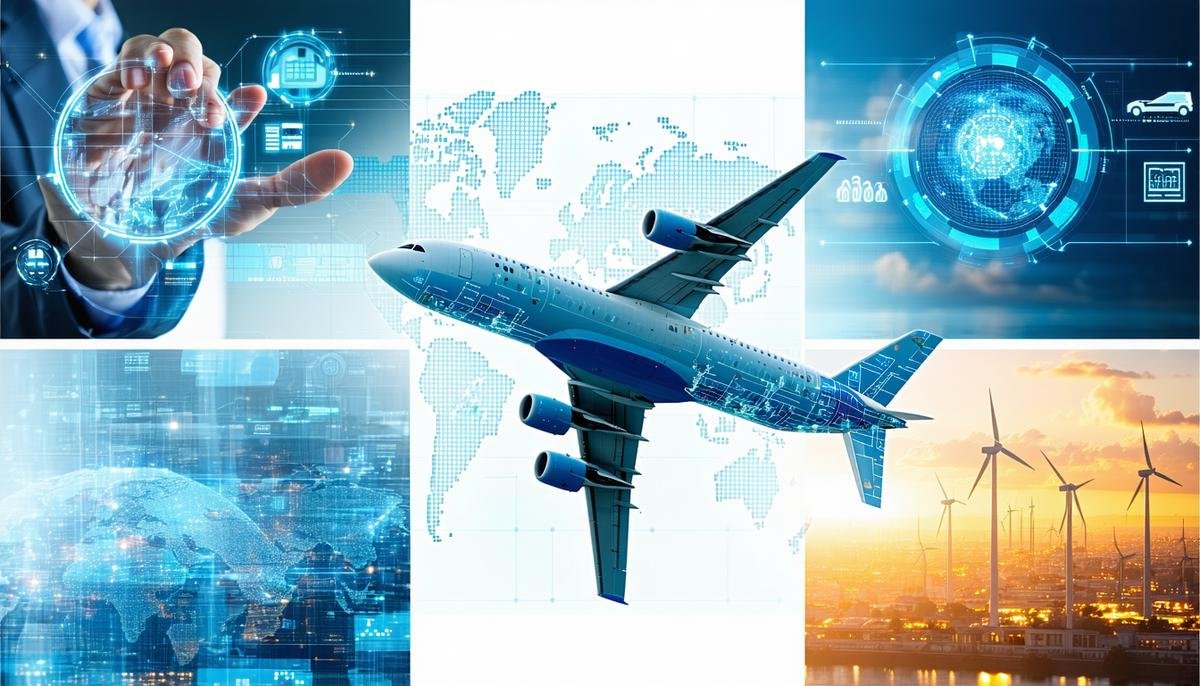
Integration with IIoT and Cloud Platforms
The integration of digital twins with Industrial Internet of Things (IIoT) and cloud platforms elevates their function from passive observers to active participants in operational ecosystems. Through IIoT integration, digital twins connect to a network of sensors and smart devices, providing a constant stream of live data.
This integration reduces unexpected downtimes and maintenance costs. By analyzing patterns and deviations in real-time data, digital twins can forecast potential failures and recommend preemptive actions.
Cloud platforms enhance digital twins' capabilities by offering scalability. With cloud integration, digital twins can expand alongside growing operations, making them suitable for both small-scale applications and large industrial deployments. The cloud acts as a central hub, allowing data from numerous twins to converge, enabling cross-system analyses and more comprehensive insights.
Cloud platforms also facilitate global accessibility and collaboration. Engineers and analysts can access updated twin models and their data remotely, solving problems and optimizing systems from anywhere.
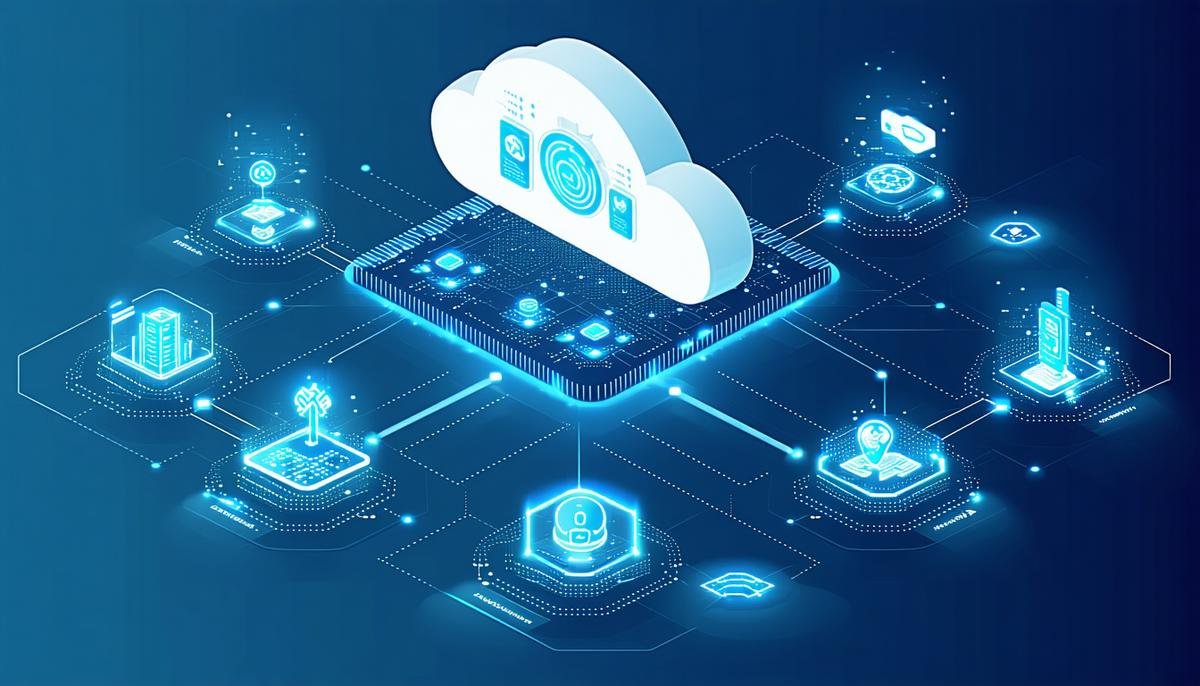
Hybrid digital twins represent a significant advancement in industrial operations management. By providing a detailed view of physical assets and integrating simulations, they offer new levels of insight and control, paving the way for smarter decision-making. As industries continue to embrace this technology, we can expect to see even more innovative applications and transformative impacts across various sectors.
- Digital Twin Consortium. Definition of Digital Twin. 2024.
- Ansys. Twin Builder: Simulation-Based Digital Twin Platform. 2024.
- Ansys. TwinAI: AI-Powered Digital Twin Software. 2024.
- IEEE. Case Study on Hybrid Digital Twin Accuracy. 2024.
- Honeywell. Battery Manufacturing Excellence Platform (Battery MXP). 2024.