Artificial Intelligence has steadily woven its threads into the fabric of wildlife conservation, presenting a blend of modern technological precision with traditional ecological practices. As we navigate this intersection, the integration of AI not only promises to enhance monitoring and protection efforts but also introduces a spectrum of challenges and ethical considerations that must be addressed.
AI Applications in Wildlife Monitoring
AI-driven technology advancements increasingly play crucial roles in the conservation of wildlife. Technologies, especially Artificial Intelligence, enhance the tracking and monitoring of species such as Siberian tigers and humpback whales. Utilized in various monitoring processes, these technologies sharpen data accuracy thus, amplifying conservation endeavors. Let’s examine some specifics of how AI contributes to wildlife conservation.
Species identification via AI technologies expedites analyzing vast amounts of camera trap images. Tools such as those developed by organizations like Microsoft and Cornell University refine the detection and recognition processes, allowing for swift identification of species from captured images. This automated identification process supports rangers and researchers in monitoring elusive or endangered species with less human error and intrusion.
These tools have seen real-world applications. For instance, Microsoft’s AI for Earth program aids in the pooling and analysis of camera trap images across continents, giving researchers dynamic tools to monitor wildlife migration patterns and population numbers globally. Automated species sorting by AI reduces human labor and sharpens the precision of population studies.
Habitat monitoring is benefiting from AI applications. Machine learning models interpret satellite and drone imagery to track habitat changes over time. This monitoring allows detection of illegal deforestation or changes in land use that might threaten biodiversity. Integrating AI for habitat monitoring aims to identify potential risks before they become unmanageable. Advanced systems can alert authorities about unusual levels of activity in protected areas that could indicate poaching or illegal logging.
For larger fauna such as whales, conservation efforts are eased by coupling acoustic monitoring with AI analysis. Specially designed algorithms can browse through thousands of hours of sound recordings collected from ocean floors, isolating whale calls and eliminating the manual labor of scanning waveforms. Tools like PatternRadio have enhanced how scientists isolate and study humpback whale songs, influencing conservation strategies in marine environments.
The impact extends to anti-poaching efforts where AI-driven tools predict poaching threats or illegal logging activities by analyzing satellite imagery and historical data. Systems like the Protection Assistant for Wildlife Security (PAWS), initially developed by UCLA, use game theory and machine learning to anticipate poacher paths and aid rangers in plotting efficient patrol routes.
Collaborations between World Wildlife Fund and tech giants help leverage expertise and resources. Advanced AI sustains continuous assessment and intervention plans that can be game-changers, especially in resource-limited natural parks.
The efficiency of these AI applications reveals its advantage: Powered by vast data inputs and developing AI models, current conservation work continuously realigns to be proactive and adaptive to new threats and challenges.
As the number of applications grows and technology deepens, the blend between traditional conservation techniques and modern robotic precision presents an intriguing marriage of eco-protection efforts solidified by AI influence, orchestrating a future where wildlife thrives under the careful watch of advanced technology platforms. Time-consuming traditional methods give way to this new guard of ecological stewardship, uplifting the quality and reach of environmental conservation worldwide.
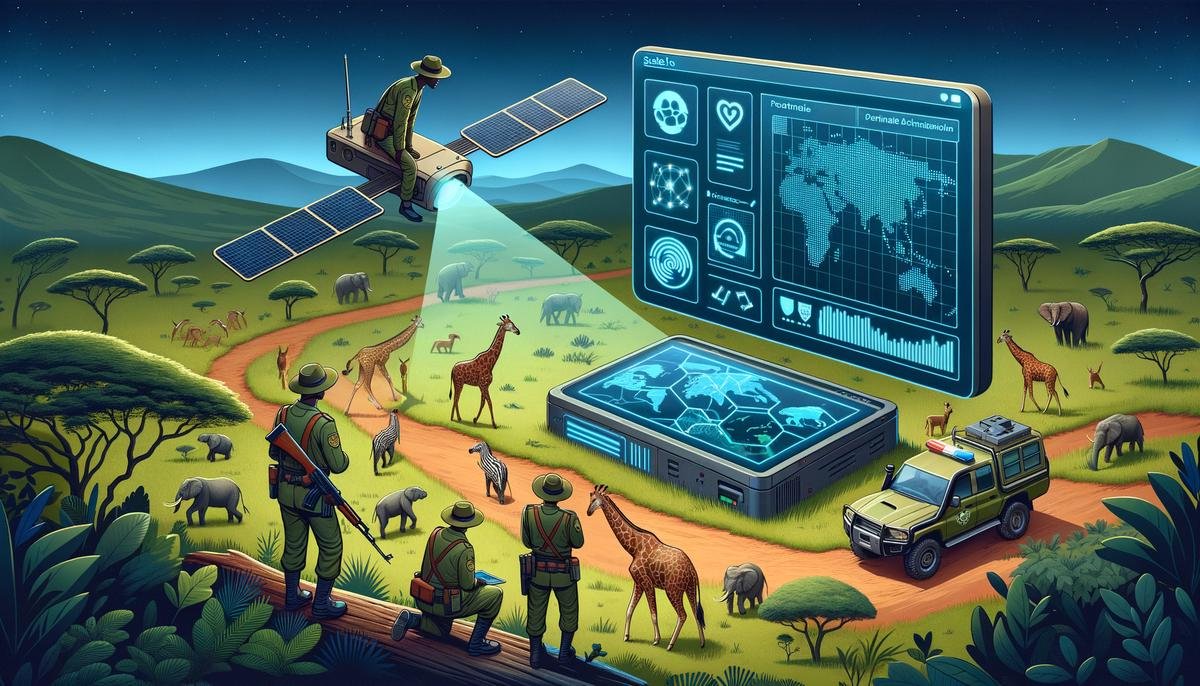
Challenges in AI Deployment
While AI technologies advance wildlife conservation, their deployment is met with challenges spanning technical, ethical, and logistical realms. Quality of data, integrity and biases of AI models, and the requirement for computational resources emerge as significant obstacles.
Accurate data collection poses a primary challenge; AI systems depend on high-quality, extensive datasets to train algorithms responsible for identifying species or habitats. Data collected through remote devices such as camera traps or acoustic sensors might suffer from incompleteness or noise-induced errors, which can influence the reliability of AI analysis. This is crucial where decisions impacting species conservation methods are concerned, as inaccuracy could lead to suboptimal or erroneous actions.
Biases inherent in AI models present obstacles often overlooked. These biases might originate from uneven sample distributions, historical survey data, or algorithmic preferences embedded within the code. For instance, an AI designed around data from a specific region may fail when applied to a different ecological zone. Another ethical concern surfaces around the potential de-emphasis of traditional ecological wisdom and practices which have sustained biodiversity for centuries.
Launching and maintaining AI-driven systems mandates substantial computational resources not uniformly affordable or accessible across all conservation areas. Robust servers, power stability, and internet bandwidth crucial for running advanced algorithms are luxuries in remote or underfunded protected areas. The ecological footprint of installing and operating technological solutions in these pristine environments also calls into question the sustainability of using such heavy-duty computational technologies.
The ethical debate around these deployments concerns how an over-reliance on technology could potentially detach human stakeholders from natural environs. This separation might weaken the connective imperative that conservationists have with the landscapes and communities they seek to protect. A technologically heavy-handed approach might alienate indigenous communities, whose involvement and traditional knowledge play vital roles in maintaining ecological balances.
Educational disparities in global conservation teams mean that technological training must precede deployment to ensure the on-site personnel, habitually skilled in biology or ecology rather than technology, can competently engage with the advanced systems.
While AI holds potential for transforming wildlife conservation practices by maximizing efficiency and breadth of monitoring capabilities, acknowledging and addressing these challenges is imperative to fully harness AI’s proficiency without compromising the natural essence and inclusive quests of global conservation objectives. Balancing tech-driven solutions with informed conversations about conservation ensures that while we step into a future of digital stewardship, we do not lose grasp of the nature those technologies aim to protect. Effective integration of AI in conservation must synergize with its broader goals—saving species in balance with nurturing human-nature interconnectedness.
Integrating AI with Traditional Conservation Methods
Integrating AI with traditional conservation methods offers a bridge between cutting-edge technology and age-old nature preservation practices. Successfully combining these approaches enhances the effectiveness and scope of conservation strategies, leveraging the best of both worlds. Case studies where such integrations have flourished provide insights into the synergy between AI and traditional methodologies.
One compelling example can be found in the Savannah regions of Africa, where conservationists use AI-powered drones alongside indigenous tracking techniques to monitor wildlife movements and deter poachers. Community rangers equipped with knowledge passed down through generations guide tech teams to hotspots where illegal activities are prevalent. The drones provide a bird’s-eye view, capturing real-time images that are analyzed by AI to swiftly detect human activity or distressed animals. This collaboration has increased the coverage area and brought down response times.
Another case study involves the forests of the Amazon where AI is integrated with the wisdom of local tribes to monitor ecological health. AI-facilitated camera traps and acoustic sensors collect extensive data on wildlife species, which is then blended with indigenous knowledge systems to draft policies that favor sustainable practices. The combination ensures that conservation measures respect and incorporate traditional values and ecological insights known to indigenous populations, fostering a sense of ownership and accountability among local communities.
By overlaying AI analytics over traditional tagging and tracking systems used for studying migratory patterns in birds, researchers have enhanced their understanding of evolving patterns in migration paths possibly altered due to climate change. AI algorithms process vast datasets on flight patterns that would take humans considerable time to analyze, allowing for timelier adjustments in conservation strategies.
Blending AI with human expertise grounds the technology in local contexts and fortifies traditional methods with data-driven support. Maintaining balances—ensuring technological interventions do not overreach, encroaching on privacy or diminishing human elements central to ecology—is crucial. It encourages adopting a ‘blended learning’ approach where AI tools become part of the ecological toolkit while traditional practices are respected and upgraded rather than replaced.
As AI technology progresses, its integration with established conservation methods presents an opportunity for a sustainable future where technology and tradition coalesce, leading to smarter, community-inclusive strategies. This synergy invites an optimistic paradigm in environmental stewardship, one that respects history while embracing the future, ensuring that conservation efforts are as dynamic and complex as the ecosystems they aim to protect.
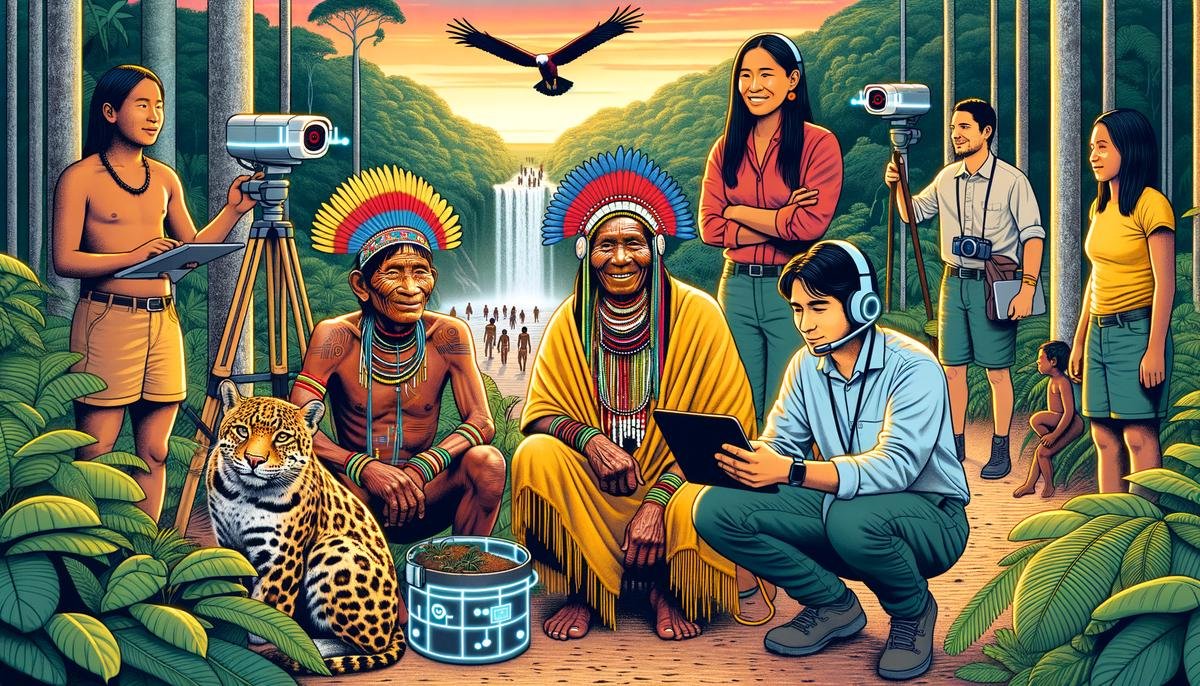
Future Prospects of AI in Conservation
The horizon for AI in wildlife conservation beams with potential and transformative prospects, particularly as we delve further into machine learning, predictive analytics, and biodiversity credit markets. Each of these elements promises to layer additional sophistication and efficiency onto existing conservation efforts, amplifying outcomes in new directions.
Significant strides are anticipated in machine learning algorithms that lean heavily on enhanced data acquisition methods and more complex analysis strategies. These evolving algorithms are poised to better predict environmental changes, offering more accurate forecasts and more frequent observational intervals. The implications for preemptive actions regarding endangered species and habitats sharpen the effectiveness of conservation measures.
Predictive analytics emerges as a striking stronghold in the arsenal of conservation tools. More than ever before, AI could help us discern not only the current status of species and ecosystems but also accurately predict future outcomes based on a plethora of environmental inputs. Predictive models can become a cornerstone in establishing preemptive conservation strategies that not merely pinpoint threats but diffuse them efficiently, before they morph into irreversible damages.
Shifting focus towards the economic instruments on the AI-enhanced conservation landscape, biodiversity credit markets are capturing interest as pivotal motivators for ecosystem restoration investments. AI’s role in these markets can be dynamic—from mapping and monitoring ecosystems reliably and efficiently, to generating verifiable data that supports the issuance of biodiversity credits. Such mechanisms encourage positive behavioral shifts towards conservation on a corporate scale by attaching tangible returns to ecological stewardship.
The incorporation of AI in the valuation, trading, and monitoring of these credits adds a layer of transparency and effectiveness typically challenging to achieve in standardized environmental policy frameworks. As we edge into data-rich methodologies, AI brings precision to how biodiversity enhancements are quantified and valued, potentially reinforcing a more organic synergy between economic pursuits and environmental preservation.
In grappling with these technological advances, it is paramount to ensure that AI tools do not solely morph into replacements for traditional conservation methods but rather function synergistically to amplify human efforts. As machine learning becomes more weighted towards extensive datasets and predictive analytics more insightful through advanced heuristics, the integration of these technologies must focus on extending the capabilities of human conservationists rather than eclipsing them.
The road maps to integrate AI within wildlife preservation promise exciting territories. What remains essential is a guided approach to adherence and harmony between these emerging tech tools and the hands-on, tactile fieldwork of conservation. By fostering a climate where technology participates as an ally—and not a replacement—of biological science and ecology, we can architect a future where AI conjures a richer, more diverse biosphere.
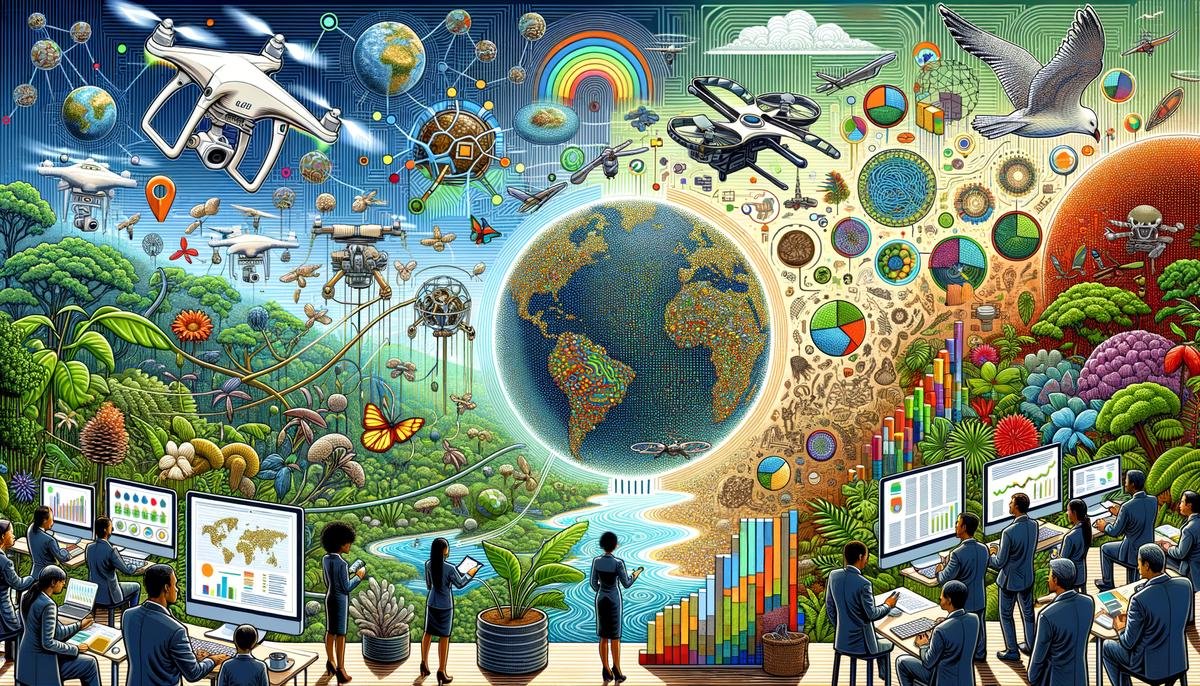
Ethical Considerations and Community Involvement
As the tapestry of wildlife conservation becomes adorned with scientific threads of artificial intelligence, difficult ethical considerations need to be managed. Turning the lens onto AI’s ascent in ecology raises ethical questions, especially related to the natural autonomy of wildlife and potential disruptions caused by dense technological infrastructures. The intervention of AI shapes the landscape of our observational methods and applies analytical lenses perhaps previously unforeseeable, balancing between aid and impairment to natural processes.
A core ethical concern relates to technologically induced disturbances in wildlife behavior and habitat. Deployment of noise-emitting devices, such as drones or automated monitors and sensor systems, can potentially disrupt animal lifestyles and routes, introducing artificial stressors into otherwise untouched ecosystems. Another concern lies in AI’s deep data gathering—ranging from genetic to geolocational information—that may veer into invasive territories, raising privacy threats to biodiversity.
Another delicate facet lies in decision-making—programming an algorithm embeds a set of biases derived from its curators’ perspectives, which inherently governs conservation decisions, sometimes overshadowing less quantifiable traditional ecological knowledge. Wherever AI threads its binary codes across natural canvases, a faithful reflection is necessary to ensure these lands remain unscathed by unintended digital footprints.
Given these ethical considerations, involving local communities in harnessing AI’s power turns critical in driving not just successful but rightly-conscious conservation strategies. Local communities offer a trove of indigenous knowledge which is crucial in outlining both the biological nuance and the cultural significance of natural repositories. Inviting participation from these stakeholders refines AI integration, lending ecological efforts precision—an integration thoughtfully steered with local insights and generational stewardship. This indigenous participatory approach cultivates responsibility; it roots technology into socio-cultural contexts, enriching biodiversity maintenance with harmonizing tunes.
Collaborations with locals go beyond tailoring ethical AI applications—it’s about endorsing AI enlightenment within these communities. Training and knowledge exchanges empower people directly affected by conservation laws to not only grasp but input into how their environment is monitored and treated, offering an equity in voice that had previously risked muffling.
Recognizing these ethical dimensions not only selects the fruits of technological progression but also realigns erratic vines grasping at unchecked developments. This conscientious re-calibration plots a cartography where technology comforts nature without heavy hands—a patient partnership written in codes both binary and culturally indelible. In this collaborative spirit, AI nurtured within local interactions and leashed by ethical clarity brings scalability to conservation, now heaved by voices that resonate through genealogies shaped by lived engagement with land.
Highlighting this inter-relation between ethical AI embodiment and community involvement elevates understanding about the complex coterie of nature-centric technology harvested for greater good. As the digital dawn escorts conservation into bright terrains, collective assurance of its pace—one respectful and receptive—fortifies a tomorrow lush with vibrant biodiversity secured by potent, yet gentle, cross-continental handshakes between tradition and innovation.
</