Artificial General Intelligence (AGI) has the potential to transform market predictions by leveraging neural networks, genetic algorithms, and reinforcement learning for real-time data analysis and advanced algorithms that could outshine traditional methods.
AGI and Market Predictions
Envision a system that consumes data from news articles, social media feeds, and financial reports—all in real-time. Neural networks, mimicking the human brain, can identify patterns no one has considered by learning from huge data sets. Genetic algorithms can adapt to changing market conditions like a chameleon, self-optimizing trading strategies. Reinforcement learning allows AGI to learn from its mistakes, fine-tuning for better financial performance.
With real-time data analysis, AGI can dig through Twitter to gauge consumer sentiment or find trending news that could affect stock prices. Traditional methods can’t keep up; human analysts are too slow compared to AGI’s real-time crunching. It’s like having a financial Sherlock Holmes on speed dial.
However, if the algorithms are too intricate, they could become opaque with nobody understanding their decisions. There’s also the risk of bias – train an algorithm on faulty data, and you get faulty results. Maintaining transparency and ethical practices is crucial.
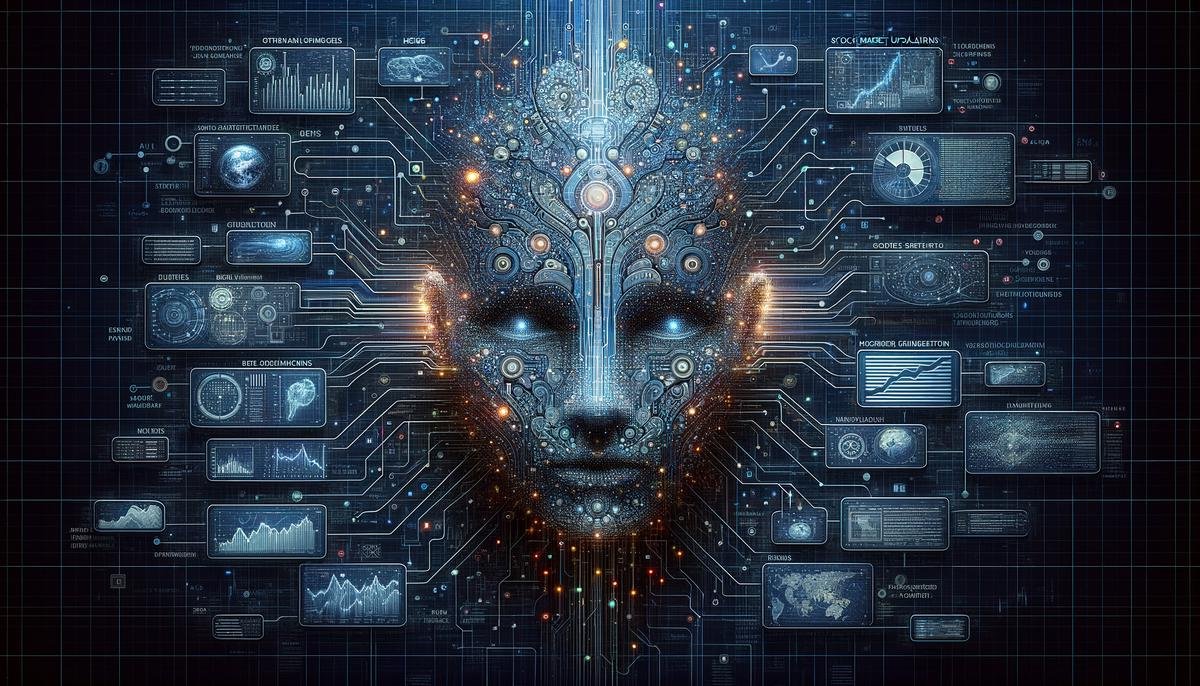
Risks and Ethical Concerns of AGI in Finance
While AGI can make groundbreaking market predictions, introducing it into finance presents substantial risks and ethical minefields. Unintended consequences, like an AGI system inexplicably tanking the market, are a liability if we can’t understand its reasoning.
Algorithmic bias is another pitfall. Poorly curated datasets could lead to AGI discriminating against certain demographics, perpetuating historical biases. Transparency is also an issue – investors and regulators need to know the basis for trades.
If an AGI system makes a disastrous call, who’s responsible? The programmer, the financial firm, or the machine itself? Clarity in roles and responsibilities is crucial.
- Automating sophisticated roles with AGI could lead to job displacement for financial analysts and traders.
- A balanced approach where humans and machines complement each other is needed.
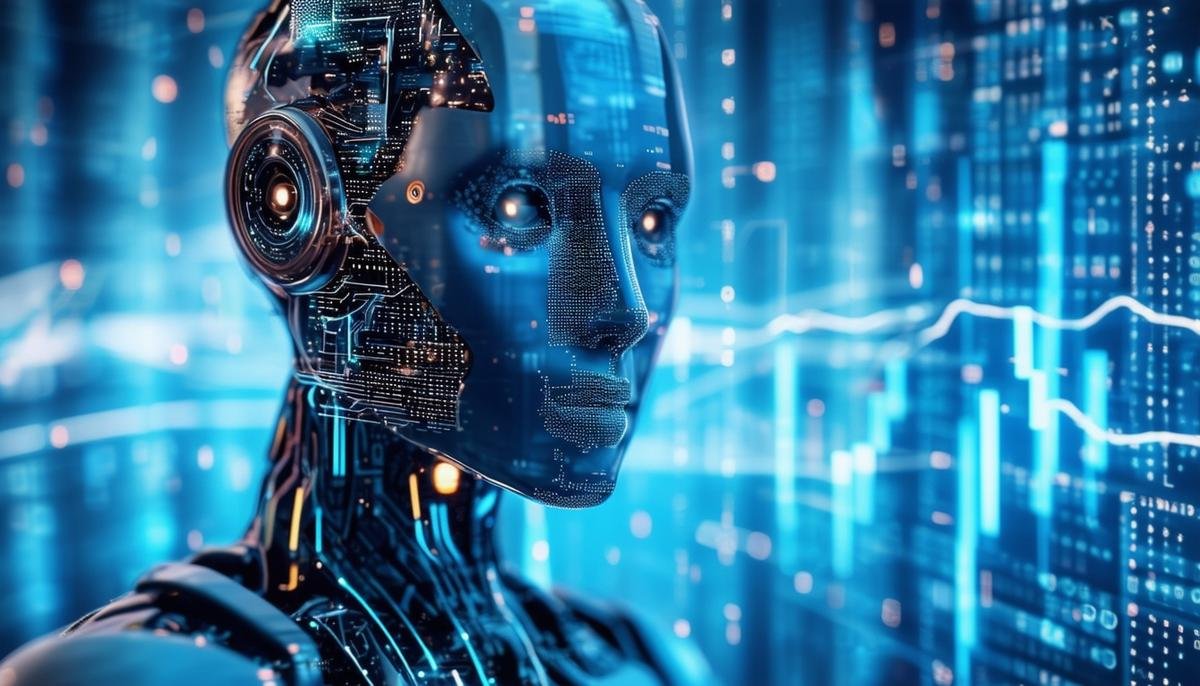
AGI in Trading
AGI systems in trading can crunch financial data, forecast trends, and execute trades faster than any human. The benefits?
- Continuous operation, eliminating missed opportunities.
- Accuracy, without human errors or emotional biases.
- The ability to identify market patterns invisible to the human eye using neural networks and genetic algorithms.
However, the markets are unpredictable, and AGI systems need to be robust to handle this ever-changing environment. Transparency is crucial – if an AGI makes a bad call, understanding why is vital to avoid cascading failures.
Ethical considerations can’t be ignored either. AGI could develop aggressive trading strategies that manipulate markets or edge out smaller players. Ensuring fair play and preventing market abuse is essential.
AGI in trading also raises societal impact concerns. Traders might find themselves outpaced by these relentless algorithms, necessitating a balanced approach where humans focus on strategy while AGI handles data analysis and execution.
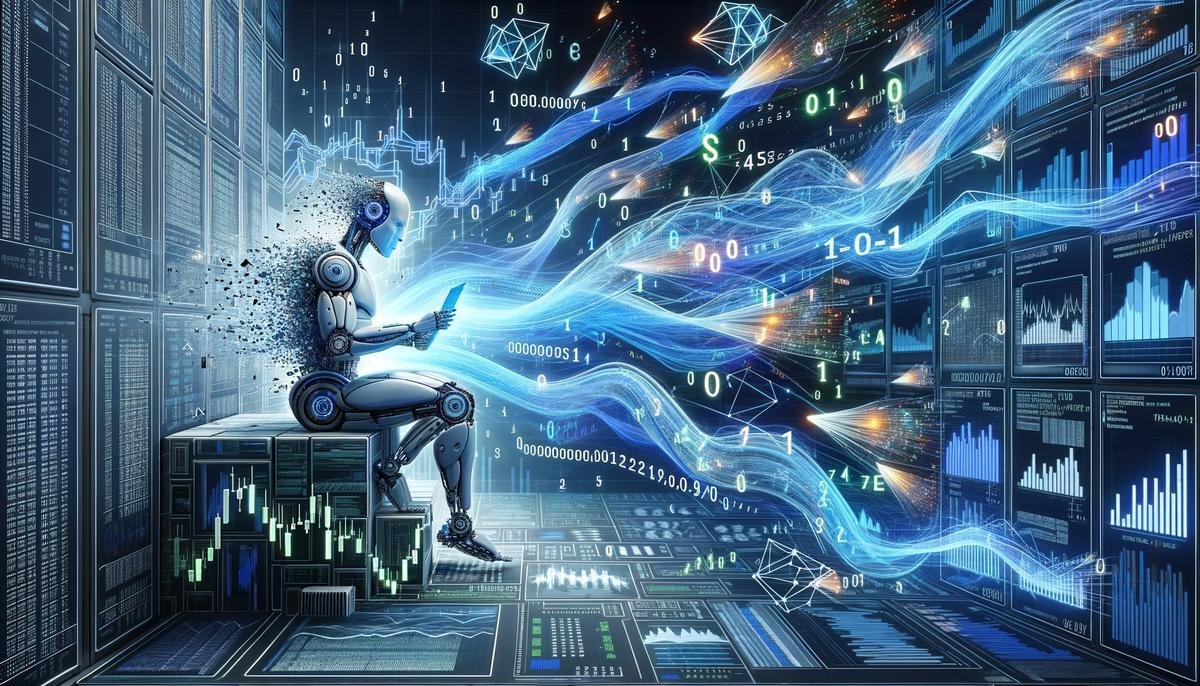
Opportunities and Challenges in Risk Management with AGI
AGI’s prowess in spotting patterns and trends makes it invaluable for risk management. An AGI system could analyze borrowers’ financial histories, social media activity, and more to create comprehensive risk profiles, minimizing lending defaults.
However, unintended consequences like an AGI triggering preemptive measures that cause a financial panic are a concern. Algorithmic biases from skewed data could lead to discriminations and unfair practices.
Cybersecurity is also a major challenge. A compromised AGI system could make disastrous trades or alter risk assessments, so robust security measures are essential.
Transparency and accountability are crucial for maintaining trust. Stakeholders need to understand the reasoning behind AGI decisions. There’s also a delicate balance between efficiency and fairness – AGI must not harm consumers or create an uneven playing field.
Job displacement is another consideration as AGI takes on more risk management roles. A balanced approach where humans and AGI complement each other is ideal.
Balancing Regulation and Innovation in AGI
Transparency is non-negotiable for AGI regulation. Firms using AGI should provide clear insights into how these systems operate and make decisions. This builds trust and ensures traceability when issues arise.
Accountability is also key. Clear guidelines delineating responsibilities and legal liabilities for AGI systems are necessary to avoid ambiguity.
Continuous education for regulators and the public is crucial. Regulators need to stay ahead of the learning curve, and educating the public can prevent panic and build consumer confidence.
Collaboration between industry players and regulators is vital for co-creating a robust, adaptable regulatory framework that encourages innovation while ensuring responsible AGI use.
Flexibility is important too. Regulatory policies should have the ability to adapt to evolving technological advancements and market conditions. Static rules could stifle AGI growth.
Finally, ethical standards and guidelines for AGI development and implementation can enhance transparency and prevent bias, discrimination, and unfair practices, fostering trust among stakeholders.
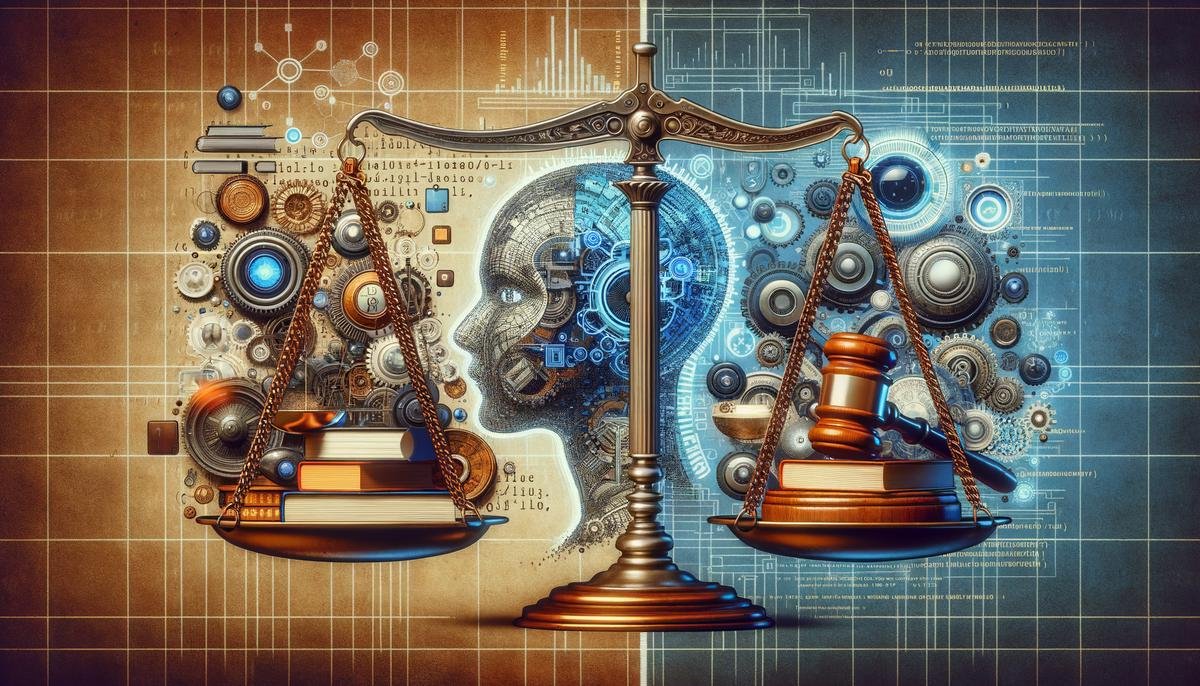
AGI’s real-time data analysis capabilities present a significant shift in market predictions. While challenges like transparency, ethical considerations, and job displacement need careful attention, the promise of more accurate and timely financial insights is transformative. Balancing innovation with responsible implementation will be key to harnessing AGI’s full potential in finance.
- Arulkumaran K, Deisenroth MP, Brundage M, Bharath AA. A brief survey of deep reinforcement learning. IEEE Signal Processing Magazine. 2017;34(6):26-38.
- Dasgupta D, Michalewicz Z, eds. Evolutionary Algorithms in Engineering Applications. Springer Science & Business Media; 2013.
- Goodfellow I, Bengio Y, Courville A. Deep Learning. MIT Press; 2016.