As we stand on the brink of a technological revolution, the digital universe burgeons with data, presenting both a challenge and an opportunity. The infusion of artificial intelligence (AI) into data analysis has emerged as a pivotal force, driving innovation and unlocking insights with unprecedented precision. At the heart of this transformation lies a synergy of machine learning algorithms, intricate neural networks, and robust statistical principles. Together, they form the foundation upon which AI can deftly navigate vast datasets, identifying trends and foreseeing outcomes that once eluded human cognition. In this exploration, we venture into the complex world of AI data analysis, unraveling how these components interlace to forecast a future shaped by data-driven wisdom.
Foundations of AI in Data Analysis
The Core Principles of AI in Data Analytics: Deciphering Patterns and Predictions
Artificial intelligence (AI) has become an intrinsic component of the modern data analytics landscape, enabling organizations and researchers to interpret vast quantities of data with unprecedented efficiency and sophistication. Thus, to appreciate the revolution unfolding in the realm of data science, it is crucial to understand the core principles that underpin AI in this context.
First and foremost, at the heart of AI in data analytics lies the principle of machine learning. This facet of AI allows algorithms to learn from and make predictions on data without being explicitly programmed for the task. Machine learning models adeptly identify patterns and correlations within the data they process, continually refining their predictions as more data becomes available.
The second fundamental cornerstone is the neural network, often inspired by the human brain’s architecture. Neural networks consist of layers of interconnected nodes that mimic the neurons in the brain, enabling the processing of complex data through a structure known as deep learning. The depth and breadth of these networks allow for the sophisticated identification of nuances that simpler models might overlook.
Predictive analytics, another key principle, demonstrates AI’s foresight capabilities. By analyzing historical data, AI can forecast future trends, behaviors, and events with a degree of precision invaluable to decision-makers in numerous fields, from finance to healthcare.
Meanwhile, natural language processing (NLP) enables AI to comprehend human language within data. It discerns meaning from text and spoken words, allowing for sentiment analysis, automated customer service, and the extraction of key information from vast digital landscapes.
Equally crucial is the principle of reinforcement learning, a type of machine learning where an AI system learns to make decisions by performing actions and observing the results. Continually refining its approach, the system effectively trains itself through trial and error, striving to maximize a notion of cumulative reward.
Data quality and ethics represent the bedrock of all AI data analytic systems. The integrity and bias of data, along with privacy concerns, are ever-present considerations, dictating the reliability and moral standing of AI analytics outcomes.
Lasty, explainability and transparency are increasingly recognized as vital. The ability to understand and trust AI’s decision-making process demands solutions that may be interpreted by humans, ensuring accountability and fostering confidence in the systems at work.
In essence, AI in data analytics is not merely a tool but a dynamic and evolving field that amalgamates complex algorithms, neural networks, predictive capabilities, language comprehension, adaptive decision-making, ethical responsibility, and intelligibility. It stands as a testament to the relentless pursuit of knowledge that defines humanity’s advancement through the information age.
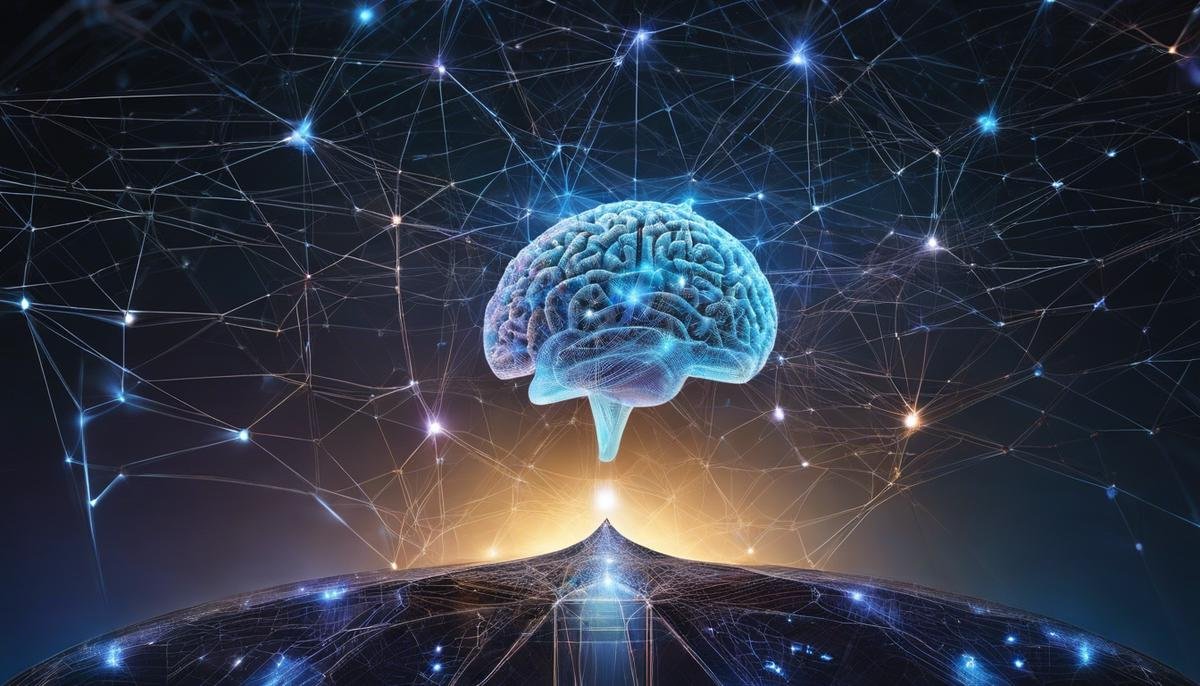
Innovative Methodologies in AI Data Analysis
Advancements in Artificial Intelligence (AI)
have invariably altered the landscape of data analysis, steering it toward greater efficiency and insightful discovery. Among these advancements are cutting-edge methodologies that significantly expand the potential of what can be quantified, understood, and predicted from vast pools of data.One such revolutionary methodology is the development of automated data preprocessing tools. These instruments utilize AI to cleanse, sort, and prepare data for analysis, mitigating the laborious and time-consuming tasks that would otherwise fall to human analysts. Automated preprocessing tools have a profound impact on data analysis by ensuring cleaner datasets which, in turn, bolster the performance of machine learning models and bolster predictive accuracy.
Furthermore, the integration of AI with the burgeoning field of big data
has catalyzed the emergence of advanced analytics techniques. The capacity to harness vast arrays of disparate data points has armed researchers with the potential to uncover correlations and patterns not discernible at smaller scales. AI-driven big data analytics permits a holistic view, where the confluence of diverse data types—from social media metrics to sensor data—can be synergistically analyzed, delivering nuanced and multi-layered insights.Another pivotal methodology is the implementation of ensemble learning. Here, multiple machine learning models, each with their unique strengths and weaknesses, are combined to improve overall predictive performance. Rather than relying on a singular approach, ensemble methods amalgamate the insights of various models, thereby reducing individual biases and variances, culminating in a more robust, collective intelligence.
Finally, the expansion of AI’s capability into the domain of simulation and scenario analysis is fundamentally reshaping data analysis. By generating synthetic data that mirrors real-world conditions, AI systems can model countless scenarios, providing organizations with the foresight to anticipate potential obstacles and opportunities. These simulations enable data analysts to test theories and hypotheses in silico, reducing risks associated with real-world experimentation.
Each of these methodologies stands as a testament to the relentless pursuit of more profound understanding and innovation in the field of data analysis. They are not the final frontier but stepping stones toward an ever-evolving horizon of possibilities. Through the meticulous application of these AI-driven techniques, the potential to decipher the complexities of data has reached unprecedented levels, heralding a new epoch of enlightenment in the digital age.
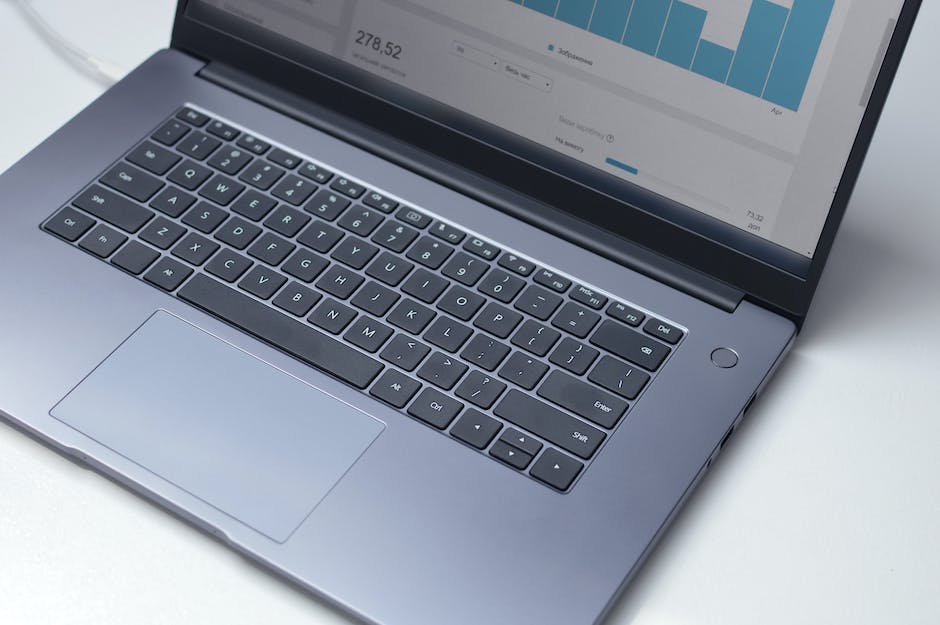
Ethical Considerations and Bias Mitigation
The Emergence of Ethical Dilemmas in AI-Driven Data Analysis: Addressing Bias for the Sake of Integrity
As the burgeoning field of artificial intelligence (AI) continues to meld with the vast domain of data analysis, ethical concerns become increasingly pressing. These dilemmas are not hypothetical—they are real challenges that confront practitioners and theorists alike, demanding attention for the trustworthiness and sustainability of AI applications.
Among the most pernicious issues is the potential for inherent bias within AI systems. Bias in AI can easily become a systemic issue, influencing outcomes in ways that may perpetuate inequalities or injustices. The sources of this bias are diverse, originating from prejudiced data sets, biased algorithm design, or discriminatory decision frameworks. The consequences of bias in AI-assisted data analysis are far-reaching and can affect everything from credit scoring and job recruitment to judicial sentencing and healthcare.
In order to grapple with these ethical concerns, steps must be taken to mitigate bias and ensure that AI systems function in a fair and equitable manner. Bias can be minimized through conscientious data acquisition strategies. For example, it is imperative to ensure that training data is as inclusive and representative as possible, capturing the multifaceted nature of real-world demographics.
Additionally, bias detection and correction algorithms have taken center stage as essential tools for scrutinizing AI systems. These algorithms can retrospectively analyze AI decisions, identify patterns of bias, and adjust the system’s function accordingly. The development of these algorithms is not merely a technical endeavor but a moral imperative as well.
Another approach is the establishment of diversely composed oversight committees. These committees, armed with expertise from various fields, can provide multidimensional evaluations of AI data analysis tools, ensuring that ethical considerations are accounted for during the development and deployment phases. Beyond this, such committees can foster public dialogue on the ethical implications of AI, promoting a society-wide ethos of responsibility and awareness.
In the quest to address these ethical dilemmas, we must not underestimate the role of international standards and regulations. Constructing a global ethical framework that can be universally adopted will harmonize efforts to curb bias and other ethical challenges, creating a cohesive strategy that transcends national boundaries.
To culminate, bias in AI is not immutable. Through vigilant efforts to recognize and correct it, we can work towards AI systems that are as devoid of prejudice as the principles of science and academia demand. Letting these systems run unchecked is untenable; therefore, with every stride in AI’s capabilities, an equal stride in ethical vigilance must follow. To do less would be to accept a digital future compromised by the very biases we strive to overcome in our broader society.
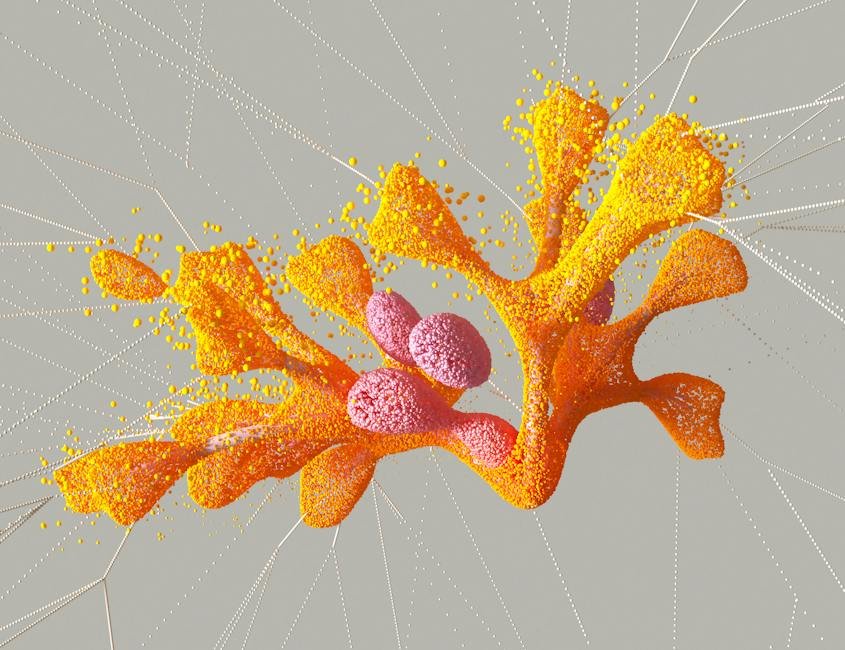
Challenges and Solutions in AI Data Analysis
In the burgeoning field of artificial intelligence (AI), data analysis stands as a critical foundation, yet it is fraught with intricate challenges that demand nuanced solutions. Central among these is the conundrum of handling the vast volumes of unstructured data. AI systems must efficiently distill meaningful patterns from this data deluge, a necessity that often surpasses the capabilities of traditional data management practices. In response, innovative machine learning algorithms are being developed to automate the categorization and analysis of such complex datasets.
Equally pressing is the issue of computational limitations. Despite rapid advancements, current hardware often struggles to keep pace with the computational demands of sophisticated AI models. This can slow the progress of data analysis considerably. Potential solutions include the advancement of quantum computing and harnessing the power of distributed computing networks, which collectively can provide the colossal computational resources required for such complex tasks.
Another predominant challenge is the dynamic nature of the data itself. Data drift occurs when the statistical properties of the target variable, which the model is predicting, change over time in unforeseen ways. This can lead to a decrease in model performance and requires constant vigilance to identify and adapt to these changes. Implementing adaptive learning systems that can detect and adjust to this drift autonomously is a crucial area of research.
Interoperability presents a further complication. As AI data analysis becomes more integral across diverse sectors, there is a pressing need for systems to communicate and exchange data seamlessly. The development of unified data standards and cross-platform technologies is vital for facilitating this. Such advancements would enable a more cohesive and functional AI ecosystem.
Furthermore, the scarcity of domain expertise poses a significant hindrance. The interpretability of AI-driven analyses often requires insight beyond mere computational prowess. Domain experts are essential in gleaning the true significance from AI-generated insights. Investment in education and interdisciplinary collaboration is essential to cultivate a workforce proficient in both AI techniques and domain-specific knowledge.
In conclusion, the field of AI data analysis is brimming with challenges, from handling unstructured data and computational inadequacies to dealing with data drift and ensuring interoperability. Simultaneously, the shortage of domain experts underscores the multifaceted nature of these issues. Addressing these challenges demands a concerted effort from the global research community, an effort that hinges on innovation, collaboration, and a relentless pursuit of efficiency and effectiveness in AI systems. The journey to overcome these hurdles is arduous, yet it harbors the profound capacity to revolutionize our data-driven world.
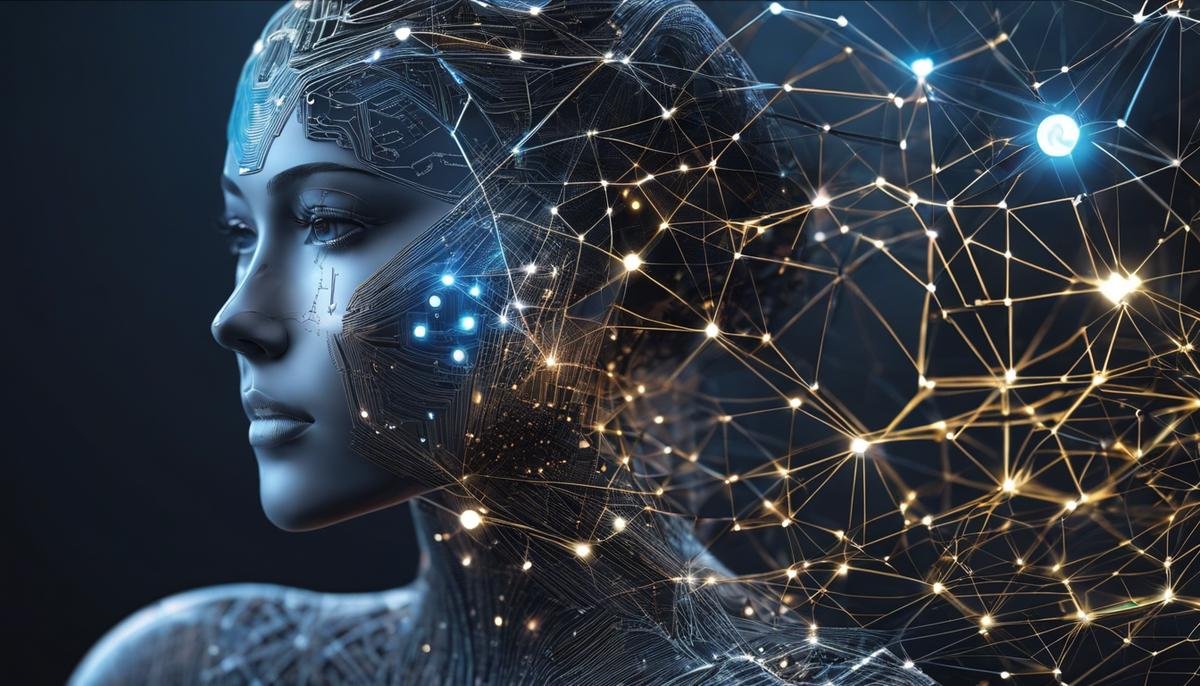
The Future and Evolution of AI in Data Analysis
As the world progresses into an era of unprecedented data proliferation, the vanguard of this evolution is Artificial Intelligence (AI). In the domain of data analysis, AI has begun to exert a transformative effect, a crescendo of innovation that promises to redefine the landscape of data examination and interpretation.
Building upon existing AI contributions to data analysis such as machine learning, natural language processing, and predictive analytics, several burgeoning technologies are poised to further catalyze the efficacy and efficiency of data analytics.
Foremost among these is the advent of automated data preprocessing tools. Such advancements are crucial as they promise to streamline one of the most time-consuming aspects of data analysis: prepping raw data into a suitable format for analysis. By doing so, they enable analysts to focus on more complex tasks that require human ingenuity.
Integration of AI with big data analytics is another horizon of potential, where AI algorithms are being tailored to handle vast datasets, discerning patterns and insights which would be inscrutable to human cognition alone. The synergy between AI and big data analytics is not merely additive but multiplicative, creating possibilities for revelations in trends and correlations on a macro scale.
Furthermore, ensemble learning—an approach where multiple models are strategically combined to solve the same problem—heralds a future where the robustness and accuracy of AI-driven data analysis are greatly enhanced. By leveraging the strengths of various models, ensemble learning encapsulates a holistic approach to data interpretation, resulting in more reliable and nuanced insights.
AI’s capabilities extend into the realm of simulation and scenario analysis, where it can construct intricate models to forecast and navigate through myriad possible futures. By doing so, organizations can anticipate and prepare for potential challenges and opportunities, thereby optimizing strategic planning and decision-making.
However, the march of technological progress is tightroped by the balancing act of ethics. A significant frontier is in handling unstructured data, which makes up a large portion of the digital universe. AI systems that can effectively comprehend and process unstructured data, such as images, video, and text, are under intensive development. These systems promise to automate and revolutionize analysis in fields such as medical diagnostics, market research, and security intelligence.
But AI, being an amplification of human capacities, also extends human limitations. Computational limitations, though continuously pushed back through advancements in hardware and algorithms, present a constantly evolving challenge to the field of AI-assisted data analysis. The innovative spirit has always found potential solutions, like the nascent quantum computing, which may hold keys to processing power previously unimaginable.
Equally significant is the phenomenon of data drift, where data evolves away from its original distribution due to changing underlying conditions over time. AI systems with adaptive learning capabilities that can recognize and adjust to such drifts are in formidable demand, ensuring that models remain relevant and accurate.
Interoperability stands out as a significant need; as data becomes more interconnected across various platforms and systems, the development of unified data standards will facilitate the seamless exchange and integration of information.
Given the breadth of knowledge and specificity required in modern data-heavy sectors, a scarcity of domain expertise has emerged as a constraint. As such, interdisciplinary collaboration is now more important than ever, where experts from diverse domains ally to inform and guide AI development and deployment for domain-specific applications.
The future of AI in data analysis is not a distant horizon—it’s an unfolding chapter. It heralds a renaissance of insight if harnessed responsibly; one where the light of data can illuminate the murkiest of uncertainties, a beacon guided by the spirit of human curiosity and amplified by the relentless march of artificial intelligence.
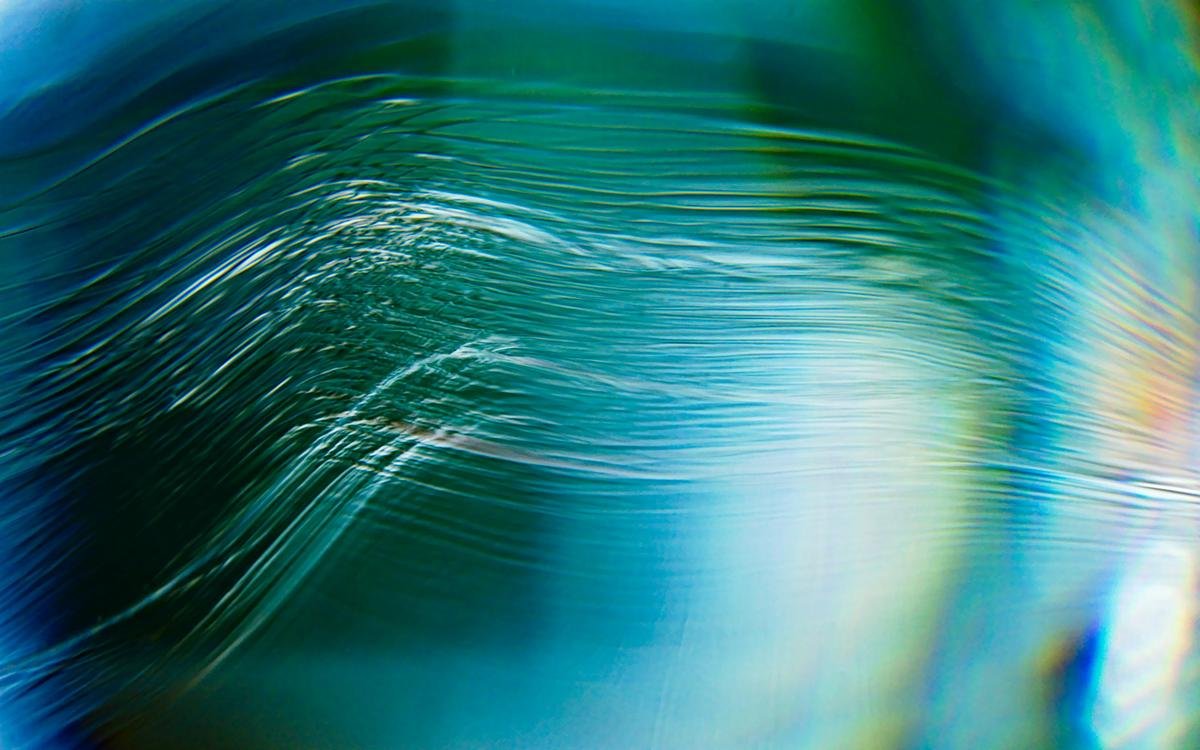
Photo by lazycreekimages on Unsplash
As we embrace the ceaseless march of progress, AI’s role in data analysis continues to evolve, transcending today’s limitations and charting a course toward an era of enlightened analytics. This journey encapsulates not only a technical expedition but also a moral quest, as we strive to infuse our digital endeavors with the highest ethical standards. By confronting challenges with innovation and addressing biases with integrity, we pave the way for a future where AI not only illuminates patterns hidden within colossal data mazes but also galvanizes industries across the spectrum. It’s a future where AI, harnessed responsibly and creatively, elevates the human experience toward greater heights of knowledge and understanding.