The quest to breach language barriers has long been a formidable endeavor, one that has shaped the course of human interaction and understanding. The advent of Machine Translation (MT) stands as a testament to this pursuit, weaving together the threads of computer science, linguistics, and artificial intelligence to forge a tapestry of global communication. From its rudimentary rule-based origins to the sophistication of Neural Machine Translation (NMT), the historical development of MT is a journey marked by relentless innovation and profound breakthroughs. As we peel back the layers of this evolutionary narrative, we gain insight into not only the technological wizardry that propels today’s AI-driven translators but also the rich tapestry of human intellect that has brought us to this point.
Historical Development of Machine Translation (MT)
The Evolutionary Journey of Machine Translation
Abstract:
Machine translation (MT), a segment of computational linguistics, has undergone significant growth over time. This article delineates the transformative pathway that machine translation has navigated since its conception, offering insights into how these changes have revolutionized communication across linguistic barriers.
Introduction to Machine Translation:
In the technologically infused era of global communication, machine translation stands as a pivotal tool in breaking down language barriers. The conception of machine translation dates back to the mid-20th century, with an objective to automatically convert texts or spoken words from one language to another, catering to an ever-growing need for cross-cultural communication and information accessibility.
Early Attempts:
The nascent stages of machine translation were marked by rule-based systems, which operated on sets of handcrafted linguistic rules. These initial attempts, while ambitious, were limited by the complexity of human language and often resulted in stilted and literal translations.
Advent of Statistical Models:
The advent of statistical machine translation (SMT) marked a radical shift from rule-based systems in the late 20th century. By utilizing large volumes of bilingual text data, SMT employed statistical models to predict the probability of certain words and phrases being accurate translations. This data-driven approach improved fluency and context-awareness in translations, albeit with some room for inaccuracies.
The Neural Revolution:
The most remarkable leap in machine translation is attributed to neural machine translation (NMT), which emerged around 2015. NMT uses artificial neural networks, mimicking human brain processing, to generate translations that are significantly smoother and more contextually accurate. This approach has led to the creation of systems that can learn from vast datasets and even self-improve over time through deep learning algorithms.
Current Landscape and Future Directions:
Today, machine translation is powered by increasingly sophisticated AI models, capable of handling complex language nuances and idiomatic expressions with far greater accuracy. The integration of NMT into translation services like Google Translate has democratized access to foreign languages, enabling instant communication across global communities.
While significant progress has been achieved, machine translation continues to evolve, with research efforts directed toward addressing the subtleties of human language, such as sarcasm, humor, and cultural references. The ongoing improvements aim to achieve near-human levels of linguistic understanding and translation quality.
Conclusion:
The trajectory of machine translation illustrates a landmark shift from rule-based limitations to AI-driven possibilities. Ongoing research and development in this area continue to enhance the reliability and ubiquity of machine translation, underscoring its importance as a tool for international discourse and the spread of knowledge.
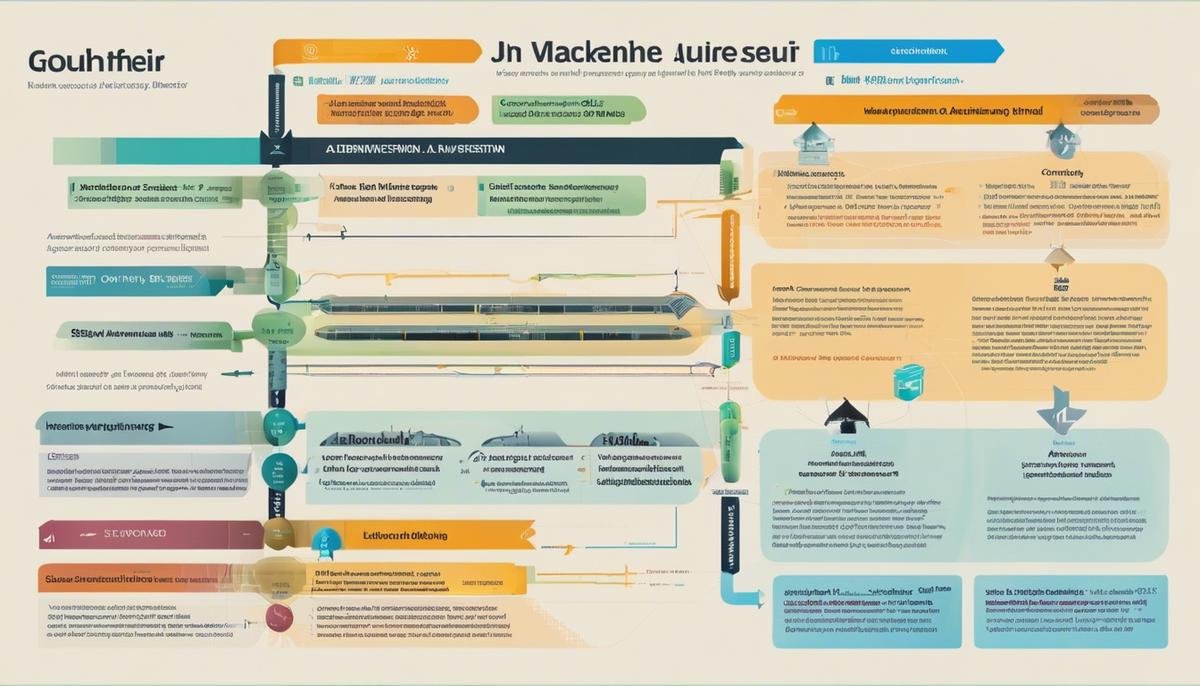
Neural Machine Translation (NMT)
Understanding the Core Mechanisms of Neural Machine Translation
Machine translation has undergone significant transformations with the rise of neural network models, which are designed to tackle the complexities of human language. Neural machine translation (NMT) is powered by a particular type of artificial intelligence known as deep learning, where large neural networks, with layers of interconnected nodes, are trained to convert text from one language to another.
The central architecture enabling NMT is the sequence-to-sequence model, which consists of two main components: an encoder and a decoder. The encoder’s function is to process the input text, typically a sentence in the source language, and to transform it into a numerical representation called a “context vector.” This context vector aims to encapsulate the essence of the input sentence, capturing not just the words but also their meaning and grammatical relations.
The decoder then takes this context vector and generates the translated sentence in the target language, one word at a time. It doesn’t merely string words together; it predicts the probability of each word based on the context vector and the sequence of words it has already produced. The decoder’s task is challenging because it must maintain grammatical integrity and convey the nuances of the source language’s meaning.
Key to NMT’s effectiveness is attention mechanisms. These mechanisms allow the translation model to focus on different parts of the source sentence at different times in the translation process, much like how a human interpreter might concentrate on particular words or phrases when translating. Attention mechanisms help overcome the limitations of earlier models that struggled with long sentences and complex expressions.
Another critical aspect of NMT is the continuous learning process. Neural machine translation models are not static. They are often trained on enormous datasets of bilingual text and continue to adapt and refine their translations based on new data. This ability to learn from a vast array of examples allows NMT systems to manage a wide range of linguistic phenomena, from idioms and colloquialisms to intricate syntactic constructs.
In practical terms, neural machine translation holds immense promise. It has revolutionized the field by improving the quality and fluency of translated texts, reducing errors that plagued earlier machine translation efforts, and bringing us closer to the goal of seamless, real-time cross-lingual communication. Although NMT is not without its challenges, such as managing linguistic diversity and the subtleties of cultural context, it represents a significant leap forward in the ongoing quest to break down language barriers. As computational power and algorithmic innovations continue to advance, the capabilities of neural machine translation will likewise expand, promising to redefine our interaction with the global tapestry of languages.
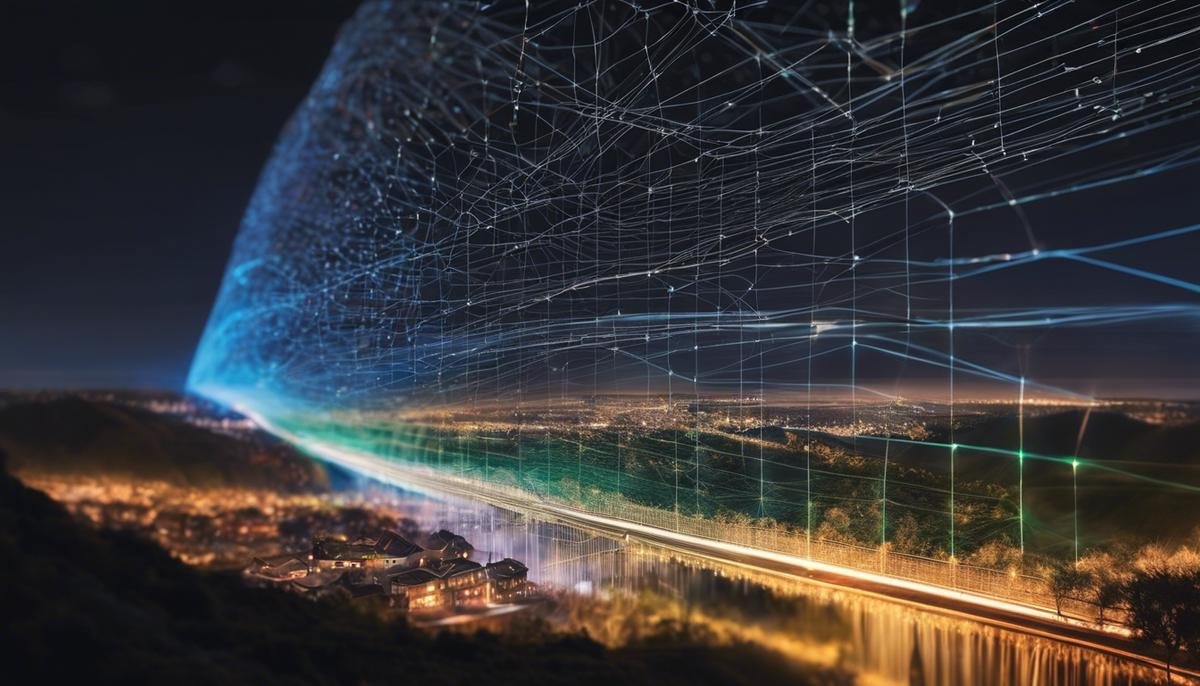
Challenges and Limitations of AI in Translation
Navigating the Nuances: The Complexities of Language in AI Translation
While the architecture of neural machine translation (NMT) has made substantial progress toward seamless translation, several hurdles still impede the path to a perfect rendition of human language. These challenges, deeply rooted in the intrinsic complexities of linguistics and culture, significantly shape the quest for improved AI translation systems.
Contextual Ambiguity and Idiomatic Expressions
One significant challenge for AI translation systems is deciphering context and idioms which are an integral part of human language. Unlike straightforward vocabulary, idiomatic expressions do not mean what the individual words suggest; “kick the bucket,” for instance, has nothing to do with physical buckets. Computing systems must therefore not only translate words but also discern and translate the intended meaning, which often requires a deep cultural understanding that AI currently lacks.
Subtleties of Syntax and Language Structures
The syntactical differences between languages pose another hurdle. The word order and grammatical rules vary enormously across languages, and an AI system must be able to restructure rather than simply replace words. The difficulty lies in maintaining the sentence’s original meaning while adhering to the grammatical rules of the target language. Variations in syntax demand an agile system that can recognize and adapt to the framework of both the source and target languages.
Handling Homographs and Homophones
Homographs are words that are spelled the same but have different meanings, and homophones sound the same when spoken but have different meanings and often spellings. AI systems can struggle to select the appropriate translation for these words without understanding the context fully. This requires not just advanced algorithms but also a rich database of language usage patterns to ensure accuracy.
Cultural Sensitivity and Localization
When dealing with translation, one must be aware of the cultural connotations and the specificities of local dialects—the process known as localization. For AI, grasping the intricacies of localization is challenging because it must account for differences that even humans may overlook, such as local customs, units of measure, date formats, and non-verbal communication cues.
Real-time Adaptation and Learning
Real-world use of AI translation often necessitates real-time adaptation and learning. Translation systems must be able to learn from new data and correct errors swiftly. This not only involves advanced algorithms but also the ability to parse and understand a wide array of topics and language styles, ranging from slang and colloquial speech to technical jargon and classical literature.
Evaluating Multiple Meanings and Ambiguities
Words or phrases in one language can sometimes translate into multiple equally plausible words in another, leading to uncertainty. AI systems must be equipped with decision-making capabilities to choose the most appropriate translation, often a Herculean task when dealing with languages that have a high level of ambiguity or where context does not clearly indicate the correct meaning.
Given these persistent challenges, the mission to refine AI translation systems continues. Progress in this arena is measured not just by the speed and accuracy of translations but by the nuanced understanding of language that embraces the full spectrum of human communication. The solutions to these challenges are poised to not only advance AI but also to deepen our own understanding of language and communication.
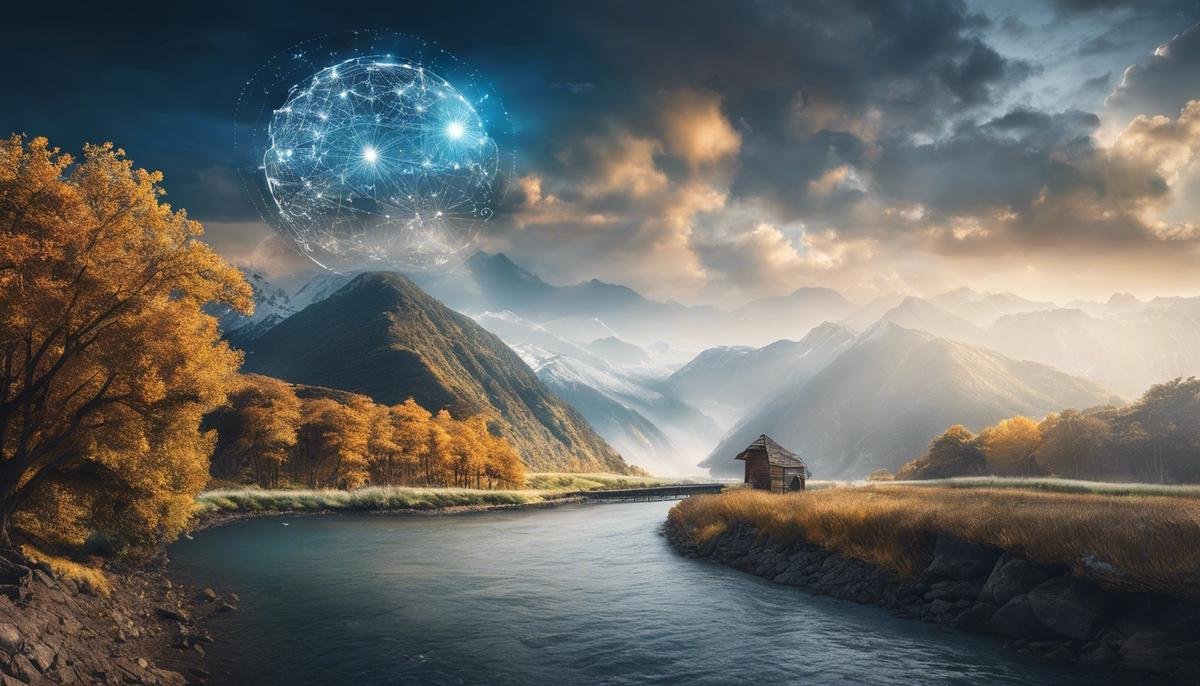
Evaluation Metrics and Methods
Assessing the Efficacy of Artificial Intelligence in Translation
Given the complexity of human languages, evaluating the efficacy of Artificial Intelligence (AI) translation is a multifaceted endeavor. AI translation, or Machine Translation (MT), is no longer an emerging field; it has become a staple in the arsenal of linguistic tools. To gauge its effectiveness, precise methods and metrics have been developed. Understanding these is crucial not only for improving machine translation systems but also for users who rely on these tools for cross-linguistic communication.
Criteria for Assessment
Primarily, machine translation is assessed on fluency and fidelity. Fluency pertains to how naturally the translated output resembles human-like text in the target language. Fidelity, on the other hand, examines the accuracy with which the original message is conveyed. Together, these aspects provide a foundation for evaluating machine translation systems.
BLEU Score: A Standard Metric
BLEU (Bilingual Evaluation Understudy) is one of the most cited metrics in the field. This algorithm computes the matching strings of words or phrases, known as n-grams, between the AI’s output and a set of high-quality human translations. Greater congruence translates to higher BLEU scores, signaling a more effective translation.
Emergent Evaluation Techniques
While BLEU and other automated metrics like METEOR and TER are informative, they have limitations. They are generally unable to capture semantic nuances and may not account for the variety of acceptable translations. Hence, human evaluation remains a benchmark for the subtleties that AI has yet to master fully.
Human Evaluation
Expert human evaluators review translations for grammaticality, adequacy, and comprehension. Does the translation maintain the meaning of the source text? Is it readable and well-phrased? These questions are indispensable in human evaluation and immensely valuable in calibrating machine translation models.
Post-editing Effort
The effort required to refine machine-translated content to a publishable quality is a pragmatic measure of its effectiveness. The lesser the editing required, the more advanced the translation model is considered.
User Feedback and Field Tests
User feedback is a treasure trove of insights. Field tests, where translated content is utilized in real-world scenarios, reveal the practical efficiency and acceptance of translation systems. They expose the AI to diverse language variations, slangs, and idioms, challenging its versatility and adaptability.
Word Error Rate (WER) and Character Error Rate (CER)
These metrics are simplistic yet informative for specific types of translation where word-order or character accuracy is vital. WER and CER identify the number of insertions, deletions, or substitutions needed to match a human translation.
Significance of Context
AI translations are scrutinized for their handling of context. Effective systems use surrounding text to interpret words with multiple meanings. This cohesion within the translated document is paramount.
Custom Evaluation for Diverse Languages
Every language introduces unique challenges. Evaluation methods are sometimes customized for language pairs to encapsulate specific linguistic features and societal norms.
Decoding Language Peculiarities
Finally, AI translation systems are tested for their adeptness at decoding language peculiarities—slang, idioms, rhetorical flair, and cultural references. Translations that manage to capture the essence of these subtleties garner acclaim for their nuanced approach.
In Conclusion
The pursuit to perfect AI translation is unending. As the world embraces increasingly advanced AI, researchers remain steadfast in their quest to refine the evaluation methods—ensuring that AI translation not only bridges languages but also encapsulates the depth and poetry innate to human communication. No summary can encapsulate ALL the nuances of this dedicated field, as it is forever evolving with the linguistic landscape and the relentless march of technology.
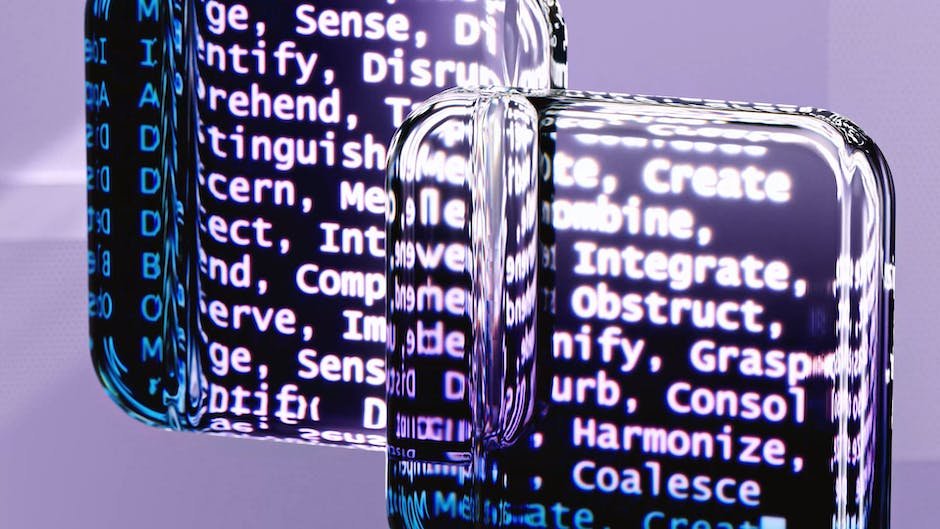
Ethical Considerations and Cultural Sensitivity
Ethical Considerations in AI Translation: Navigating the Complex Terrain
Artificial Intelligence (AI) translation systems, specifically Neural Machine Translation (NMT), have dramatically changed the landscape of language translation with their ability to learn and adapt. These systems can render text from one language to another with increasing proficiency, yet this advancement brings to the forefront several ethical concerns that must be considered.
The first of these concerns is the representation and fairness to all languages. AI translation systems are often trained on vast datasets composed predominantly of high-resource languages, such as English, Mandarin, or Spanish. This practice leads to a technological divide, enhancing the ability to accurately translate popular languages while leaving less common languages, typically associated with developing regions, less accurately represented and serviced.
Another critical ethical consideration is the preservation of cultural nuances in translation. Language is a vehicle of culture, and improper translation can result in the erosion or misinterpretation of cultural contexts. While AI systems might adeptly translate literal meanings, the subtleties of cultural expressions, humor, or local idioms present a significant challenge. The act of translating cultural context accurately is paramount, not only for the transmission of information but also to uphold the respect and integrity of the source material and its origin.
Consent and privacy issues also arise with AI translation. When individuals use online translation services, their input data can be retained and used to train and refine AI models. Users may unwittingly contribute sensitive or proprietary information that becomes part of a dataset, raising concerns about data ownership and the unauthorized use of personal or confidential information.
Employment implications must be pondered too. The progression of AI translation could potentially displace human translators, particularly in standard and repetitive text translations. While AI can assist and enhance the productivity of human translators, the ethical response to the potential loss of jobs in this profession warrants a serious discussion about re-skilling and the evolving role of human expertise in the age of AI.
Bias in AI is a universally acknowledged challenge, and in the realm of translation, it magnifies the potential for misrepresentation and stereotyping. Bias can enter AI systems through the datasets they are trained on, reflecting historical inequalities or societal prejudices embedded within the language used in these datasets. Efforts to de-bias these systems are imperative to prevent the perpetuation and amplification of social prejudices across languages and cultures.
Lastly, the reliability and accountability of AI translations for critical information must be taken into account. In legal, healthcare, and diplomatic settings, translation errors can have far-reaching consequences. Ensuring the trustworthiness of AI translations in contexts where miscommunication could result in life-altering outcomes is an ethical priority. Who is to be held accountable for these errors—the developers, the users, or the AI itself?
In conclusion, while the stakes are high, it is the collective responsibility of researchers, developers, policymakers, and society at large to navigate these ethical considerations with care. It is not merely about creating technology that can translate, but rather about ensuring that these AI systems do so in a manner that is equitable, culturally sensitive, and ethically responsible to the global community they serve.
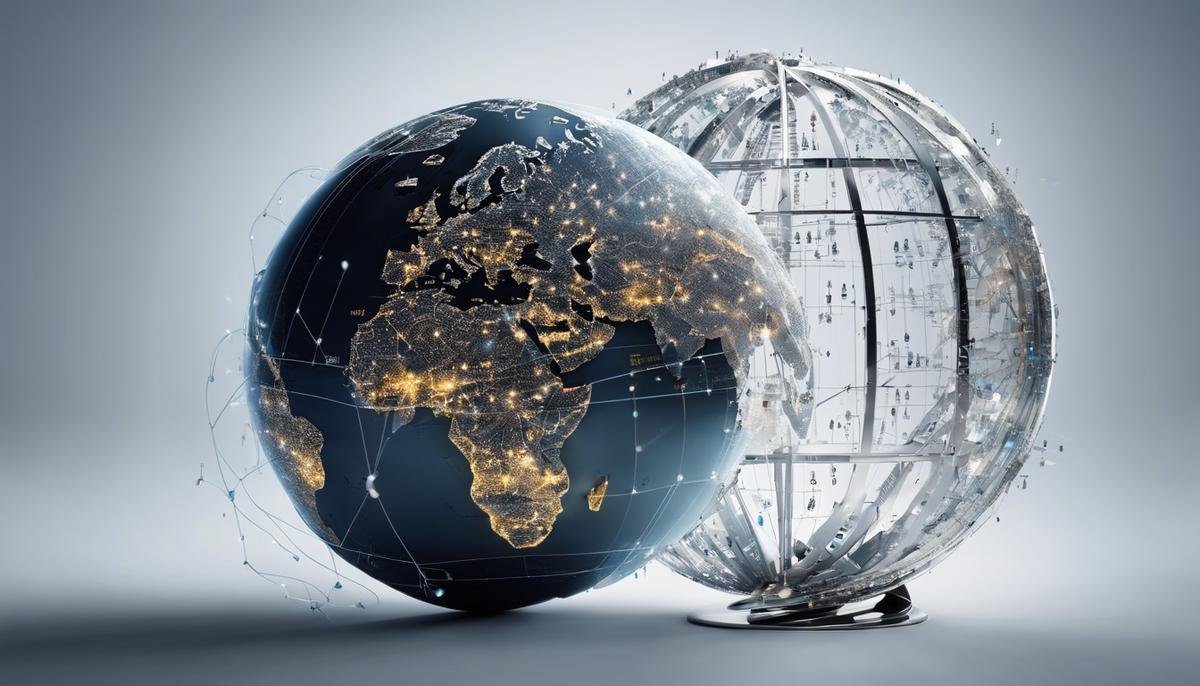
Future Directions and Research in AI Translation
Advancements in Interlingual Representation for Precise Translation
The field of machine translation, having progressed from rule-based systems to advanced neural models, now stands on the cusp of a new frontier: the cultivation of interlingual representation that surpasses mere word-to-word conversion. In this realm of translation, the anticipation of future developments promises transformations in how artificial intelligence comprehends and communicates the essence of language.
Refinement of Contextual Understanding
A core focus lies in the enhancement of AI’s ability to interpret context with sophistication equal to human intuition. Upcoming models are expected to delve deeper into the pragmatic and semantic elements of language, utilizing larger contextual windows and advanced algorithms. This evolution will reduce errors in situations where meaning is reliant on context rather than direct lexical cues, improving the overall translation of nuanced passages and complex dialogue.
Semantic Preservation Across Cultural Boundaries
Future AI translation aims to honor and preserve the original intent and emotional tone of source material. Advancements will likely incorporate ethnolinguistic data, enabling AI to bridge cultural gaps and adapt language in a way that stays true to the original cultural context while being meaningful in the target culture. This preservation will uphold the richness and diversity of human expression in AI-mediated communication.
Advancements in Real-Time Translation
Expect significant progress in the capacity for real-time, on-the-fly translation. Forthcoming innovations will potentially allow instantaneous translation of spoken language with high accuracy in diverse settings, from international conferences to remote education. This feature will pivot on the ability of AI systems to process and integrate new data rapidly, learn from interactions, and adapt to dynamic linguistic environments.
Ethical Considerations in AI Translation
As AI translation tools become pervasive, ethical considerations will take center stage. Developers will need to address consent and privacy, ensuring that the use of personal and cultural information for language modeling complies with ethical standards. Additionally, the fair treatment of all languages in machine translation models is vital, particularly for underrepresented or low-resource languages, fostering inclusivity in global communication.
Reduction of Biases in Translation Algorithms
Future advancements will also combat biases inherent in machine translation. AI models will be refined to mitigate gender, racial, or cultural prejudices that may arise from biased training data. Efforts will include diversifying datasets and incorporating anti-bias measures into algorithm design, aiming for translations that are fair and objective.
Enhancing Reliability in Critical Scenarios
The reliability of AI translation in scenarios where accuracy is critical, such as legal texts, medical instructions, or emergency alerts, will see marked improvements. Mechanisms ensuring accountability of AI translations will become indispensable, with more stringent validation processes and fallback protocols to human translators in scenarios where precision is non-negotiable.
In conclusion, the trajectory of advancements in AI translation is geared toward a more empathetic, context-aware, and ethically responsible application of technology. While challenges remain, the path forward is illuminated by a commitment to preserving human connection across the vast tapestry of languages that characterize our world.
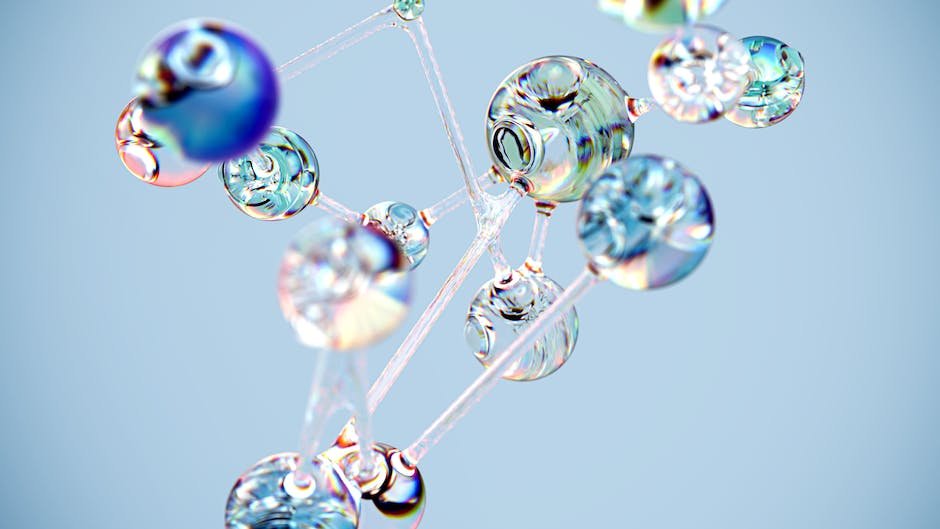
As the horizons of our global village expand, the dynamics of communication evolve in tandem, with AI translation playing an increasingly pivotal role. The journey of MT, fraught with challenges and adorned with victories, continues to spiral upwards, shaping a future wherein language diversity enriches rather than hinders. Delving into the junctures of ethics, culture, and advanced research serves as a beacon, guiding the development of more empathetic and inclusive translation tools. As this quest unearths novel solutions and navigates uncharted linguistic landscapes, the anticipation of a world ever more connected by the lifelines of understanding and shared language is not just a distant dream but an inevitable destination.