Predictive analytics is transforming how businesses make decisions by leveraging historical data to forecast future events. This article examines the methodologies, applications, and benefits of predictive analytics, as well as the challenges that come with it.
Understanding Predictive Analytics
Predictive analytics is a branch of data analytics that forecasts future events using historical data. It relies on statistical modeling and machine learning techniques to identify patterns and predict outcomes.
Statistical modeling is a core technique in predictive analytics. It involves creating mathematical models that represent relationships between variables in the data. For instance, regression analysis can predict continuous outcomes based on input features.
Machine learning enables models to learn from data over time, improving accuracy as more data is fed into the system. Common techniques include:
- Decision trees: Classify data into branches to make predictions
- Neural networks: Recognize patterns and solve complex problems
- Clustering: Group similar data points together
The global predictive analytics market was valued at $12.49 billion in 2022 and is projected to reach $38 billion by 2028, with a compound annual growth rate of about 20.4%.1
Predictive analytics is essential for informed decision-making and strategic planning across various industries. In finance, it helps forecast market trends and assess credit risk. In healthcare, it can predict patient outcomes and personalize treatment plans.
The process involves:
- Data collection
- Cleaning and preprocessing
- Statistical modeling and machine learning algorithm application
- Generating predictions
As the field evolves, the integration of AI continues to improve the accuracy and applicability of predictive analytics, making it indispensable for future-oriented businesses.
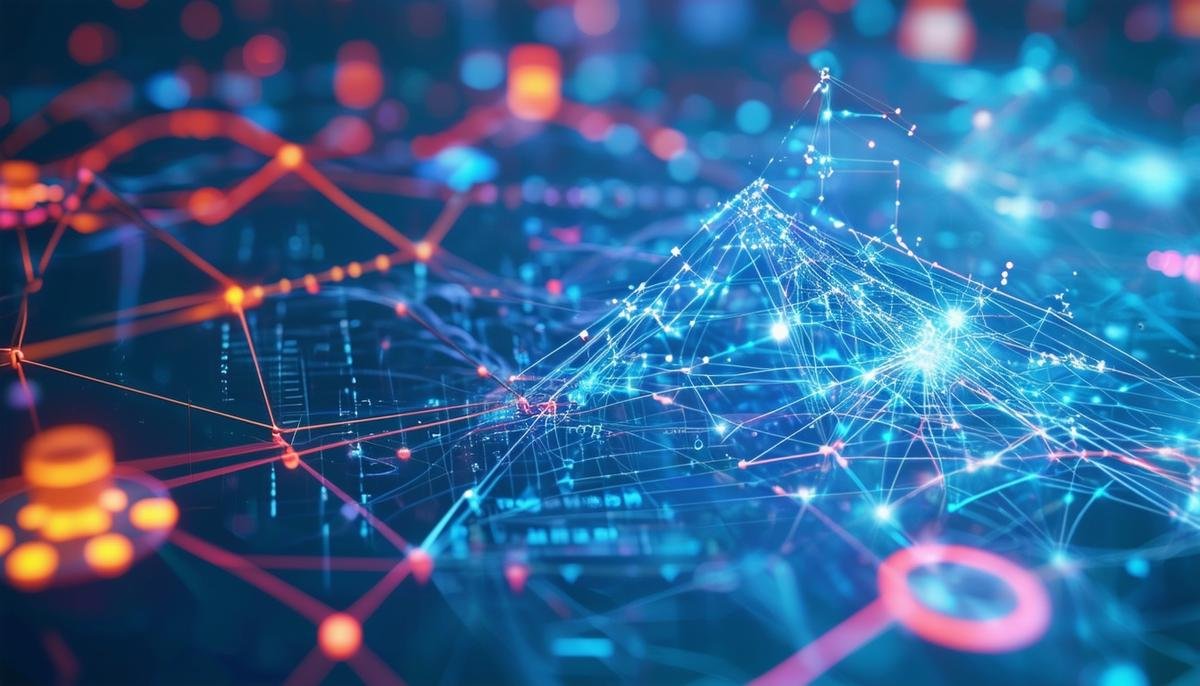
Applications of Predictive Analytics in Business
Predictive analytics is making an impact in various industries:
Industry | Applications |
---|---|
Finance | Risk management, fraud detection, credit risk assessment |
Healthcare | Patient risk identification, resource allocation, personalized medicine |
Retail | Inventory management, customer engagement, demand prediction |
Manufacturing | Predictive maintenance, supply chain optimization |
Telecommunications | Network management, customer retention |
Transportation and logistics | Route optimization, asset utilization, demand forecasting |
As companies continue to harness predictive analytics, we can expect more innovative applications across various sectors.
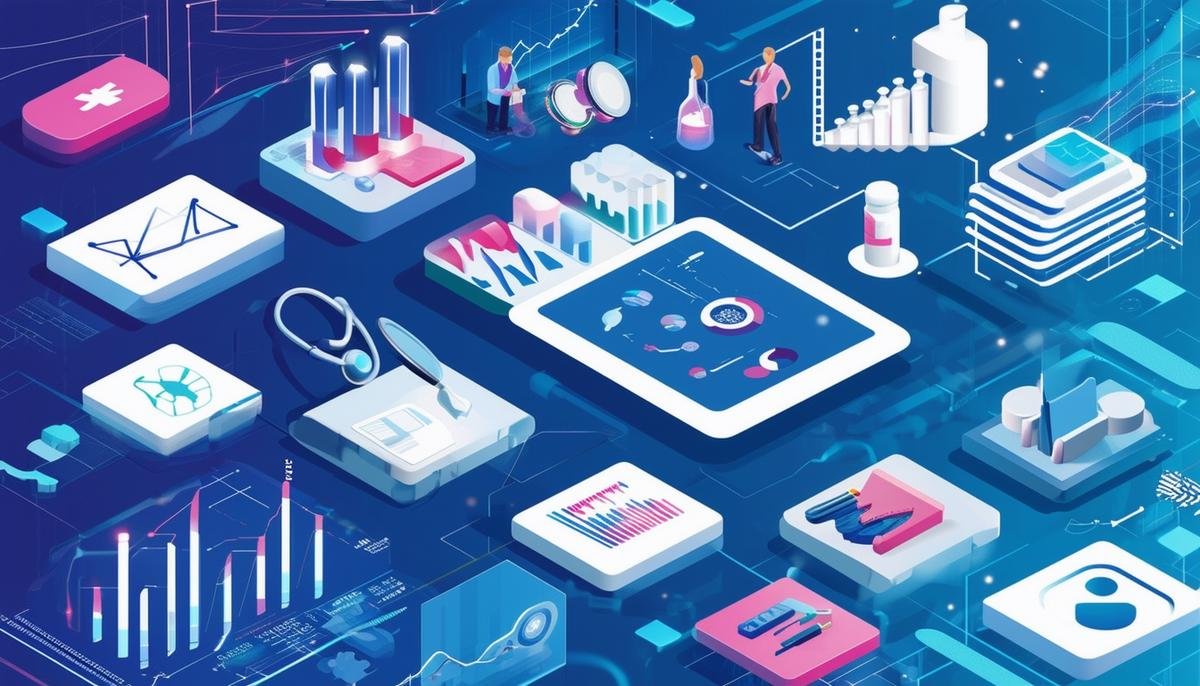
Benefits and Challenges of Predictive Analytics
Benefits of predictive analytics include:
- Improved decision-making
- Enhanced risk management
- Increased operational efficiency
- Better customer experiences
Challenges include:
- Data quality issues
- Integration difficulties with legacy systems
- Scarcity of skilled professionals
- Ethical and privacy concerns
Organizations must address these challenges through strategic investments in technology, talent, and ethical practices to fully harness the power of predictive analytics.
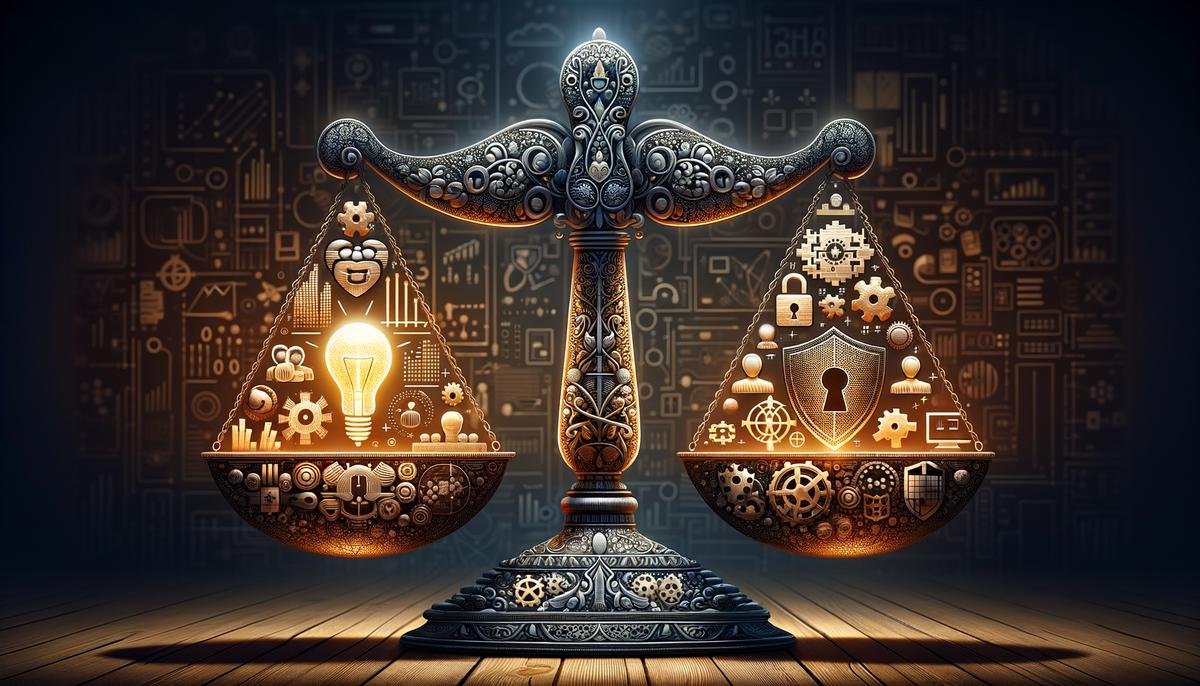
AI Enhancements in Predictive Analytics
AI has significantly enhanced predictive analytics through:
- Machine learning algorithms: These learn and adapt from data, improving predictive accuracy over time.
- Neural networks: Capable of recognizing complex patterns in large datasets, especially useful for tasks like image recognition and time-series forecasting.
- Synthetic data generation: Creates artificial data to supplement real data, addressing scarcity and privacy concerns.
- Real-time processing: Enables timely decision-making by analyzing streaming data.
Challenges in AI-enhanced predictive analytics include ensuring data quality, managing complex models, and addressing ethical considerations. Organizations need to implement sound frameworks and governance policies to address these challenges effectively.
“As AI continues to evolve, its integration with predictive analytics will unlock new possibilities across various industries.”
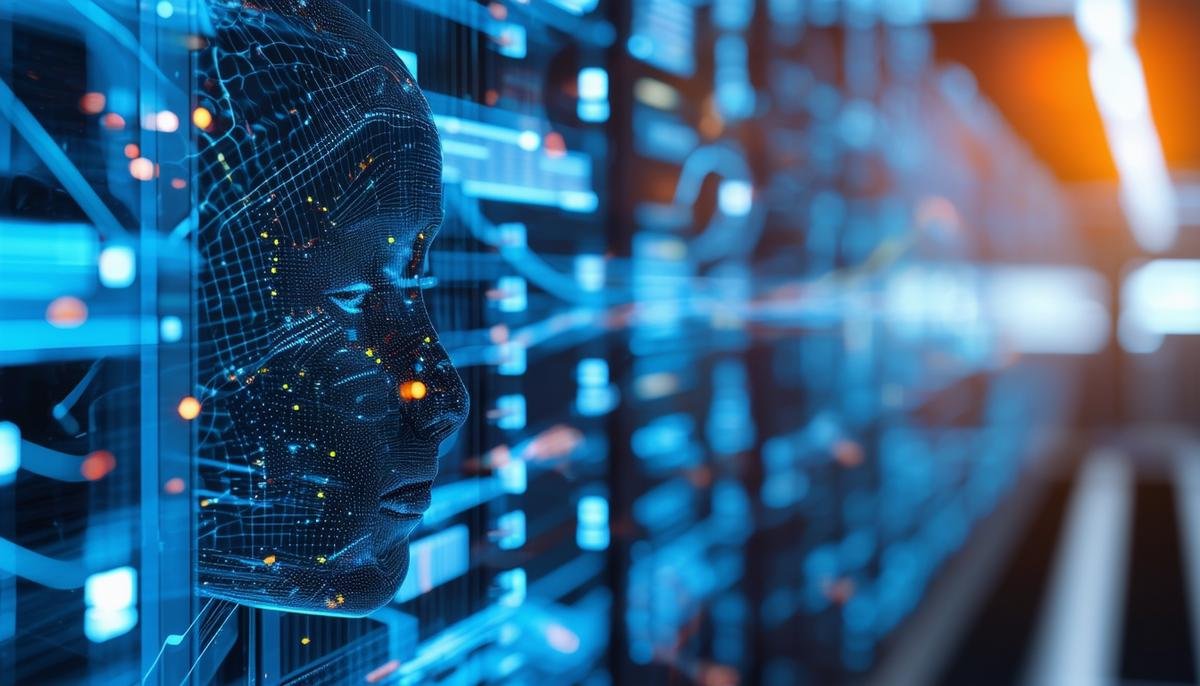
Ethical and Practical Considerations
When using AI-driven predictive analytics, ethical and practical considerations are crucial. Addressing these concerns requires regulations, best practices, and continuous oversight.
Data bias is a primary ethical challenge. AI models are only as good as their training data; if historical data contains biases, these can be perpetuated or amplified. Organizations must implement data auditing procedures to identify and rectify biases. Techniques such as:
- Re-sampling
- Re-weighting
- Fairness constraints
can help ensure equitable outcomes.
Privacy concerns are significant in AI-driven predictive analytics. Compliance with regulations such as GDPR and CCPA is essential. Adopting privacy-by-design principles can embed privacy considerations into the development process. Techniques like anonymization, data encryption, and differential privacy can further safeguard sensitive information.
Responsible AI use involves addressing bias and privacy and ensuring transparency and accountability. Organizations should adopt techniques for explainable AI (XAI) to make AI’s decision-making processes more transparent.
Implementing AI-driven Predictive Analytics
Implementing AI-driven predictive analytics requires careful planning and execution. Ensuring high-quality data availability is crucial. This involves investing in data collection and management infrastructure. Data cleaning and preprocessing are important stages that impact the accuracy of predictive models.
Integrating predictive analytics into existing systems and workflows can pose challenges. Legacy systems may require upgrades or middleware solutions. AI model deployment should minimize disruptions to ongoing operations.
Skill gaps within the workforce can pose challenges. Organizations must invest in upskilling their existing workforce or hiring specialized talent. Partnerships with academic institutions, industry consortia, and external consultants can provide additional expertise.
Continuous monitoring and evaluation are essential for maintaining the effectiveness of predictive analytics. AI models should be regularly retrained and fine-tuned using new data to ensure their accuracy and relevance.
Fostering a culture of ethics and responsibility within the organization is important. This involves:
- Setting clear guidelines and policies for ethical AI use
- Providing training on ethical considerations
- Encouraging accountability
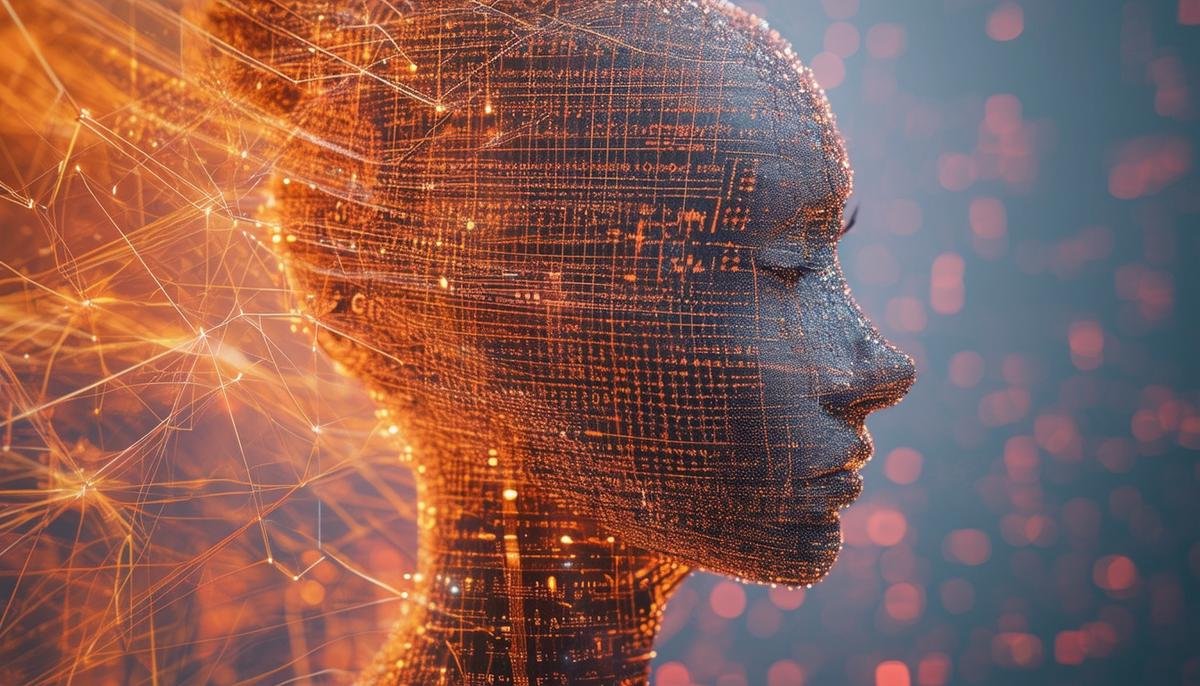
Future Trends in Predictive Analytics and AI
The future of predictive analytics and AI promises to be dynamic. Advancements in generative AI, real-time analytics, and integration with other technologies will expand their applications across various sectors.
Generative AI can create synthetic data that mirrors real-world data, enabling the simulation of a wide range of potential scenarios. This is valuable in fields where historical data is limited or outdated, such as climate science1.
Real-time analytics is gaining momentum, allowing organizations to make instant, data-driven decisions. In retail, for example, this can help businesses adjust pricing strategies dynamically based on current market conditions.
The integration of AI with other technologies is shaping the future of predictive analytics:
- IoT: In agriculture, IoT devices can monitor soil conditions, weather patterns, and crop health, while AI-driven models analyze this data to optimize farming practices.
- Blockchain: Can enhance the reliability of predictive models by ensuring data integrity and traceability.
- Edge computing: Enhances the efficiency of real-time predictive analytics by processing data closer to its source.
The democratization of predictive analytics and AI is another emerging trend. Low-code and no-code solutions enable business users to create and deploy predictive models without extensive programming knowledge.
Explainable AI (XAI) is gaining importance as predictive models become more complex. XAI techniques aim to make AI decision-making processes understandable to humans, crucial for building trust and ensuring ethical AI applications.
Advancements in natural language processing (NLP) are improving the usability of predictive analytics. NLP enables the extraction of insights from unstructured data, such as text and speech.
“The ethical and responsible use of predictive analytics and AI is becoming a focal point for organizations. Establishing comprehensive governance frameworks that address issues such as bias, privacy, and accountability is imperative.”
As these technologies continue to evolve, organizations must address associated ethical and practical challenges to fully harness their potential and drive meaningful outcomes.
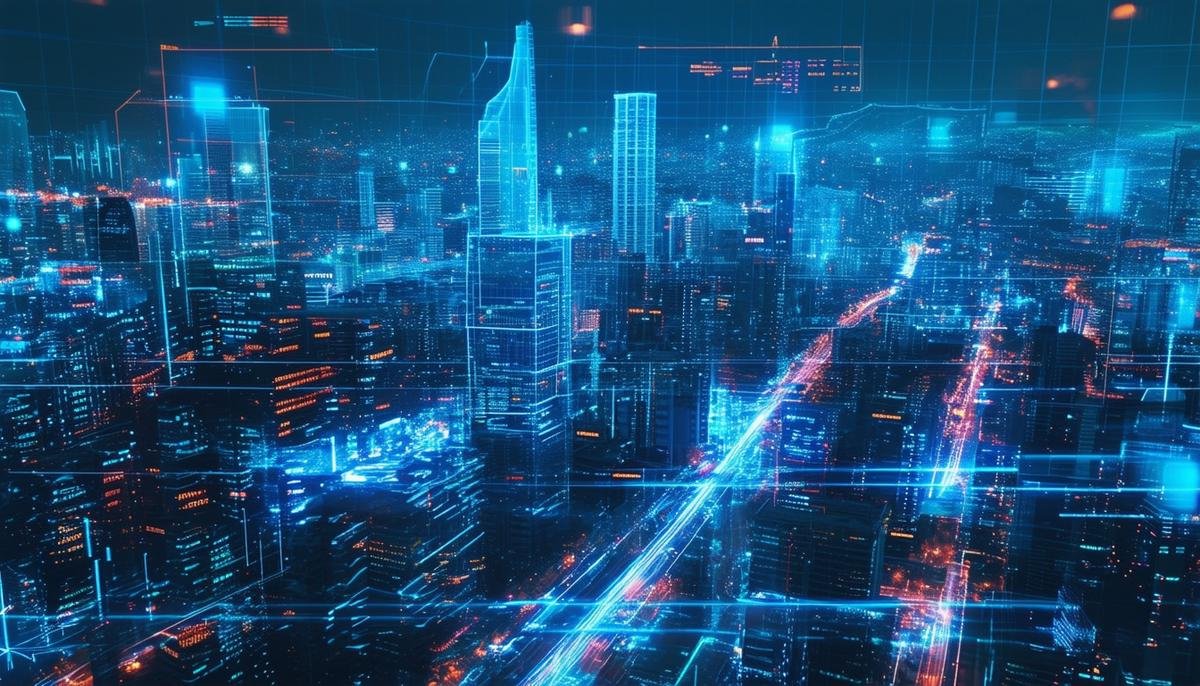
In summary, predictive analytics offers significant advantages in improving decision-making, risk management, and operational efficiency. While challenges exist, addressing them through strategic investments can maximize the potential of predictive analytics. As AI continues to evolve, its integration with predictive analytics will drive innovation and efficiency across various industries.