Challenges in Climate Prediction
Predicting climate change effects is complex and full of unexpected challenges. Climate models face several issues, with data scarcity being a major problem. There's a lack of long-term, high-quality observations from around the world, making it difficult to create accurate predictions.
The interactions between the ocean, atmosphere, and land are intricate and hard to model. Predicting the timing and intensity of extreme weather events like hurricanes, heatwaves, and droughts is particularly challenging.
The computational cost of running climate models is significant. These models must incorporate numerous variables and data points while using algorithms that require substantial processing power. As research progresses, new climate behavior patterns are discovered, necessitating even more advanced computations.
Despite these hurdles, scientists continue to work on improving climate predictions, recognizing the importance of this work.
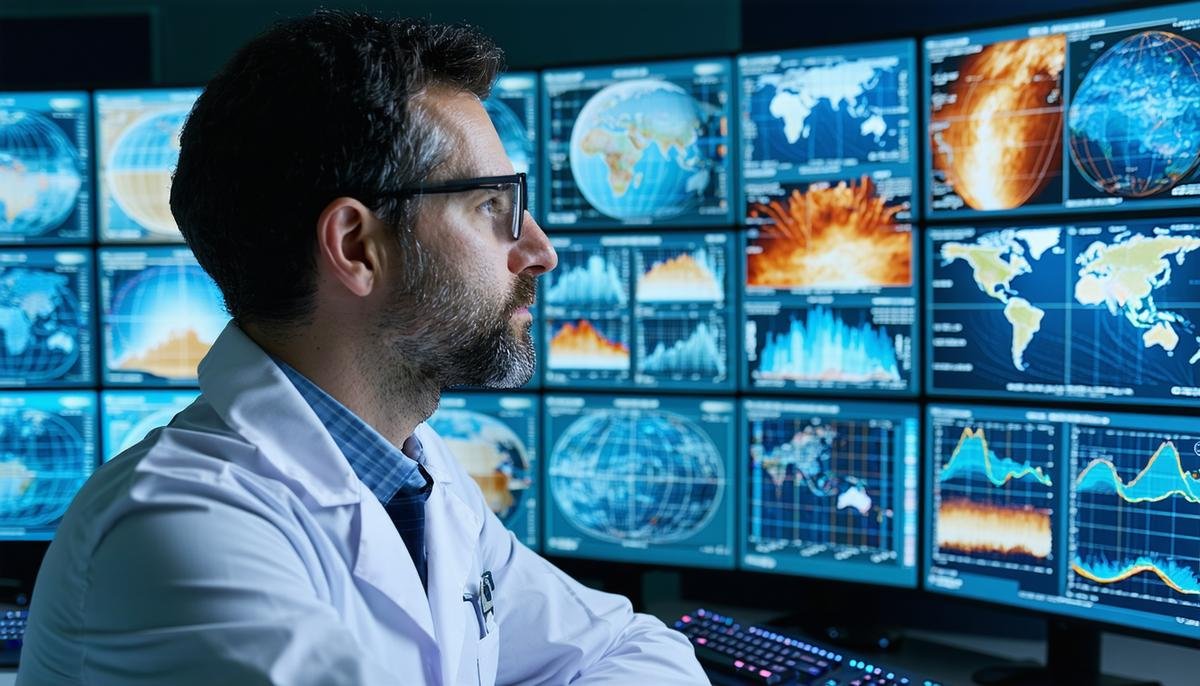
AI and Machine Learning Applications
AI and machine learning are emerging as important tools in climate prediction. These technologies can analyze vast amounts of data quickly, uncovering patterns and relationships that might take human researchers much longer to discover.
AI is particularly useful in predicting temperature extremes, such as heatwaves and cold snaps. Machine learning algorithms can sift through historical climate data to enhance prediction accuracy.
For droughts, machine learning models can simulate different scenarios and observe patterns in weather data to provide early warnings. This foresight can help communities better prepare for dry spells.
AI is also improving cyclone prediction by processing real-time data and historical patterns, leading to more accurate forecasts about storm paths and intensities.
The advantage of AI lies in its ability to detect and analyze complex meteorological interactions. It thrives on data diversity and quantity, adapting and refining predictions with incoming information.
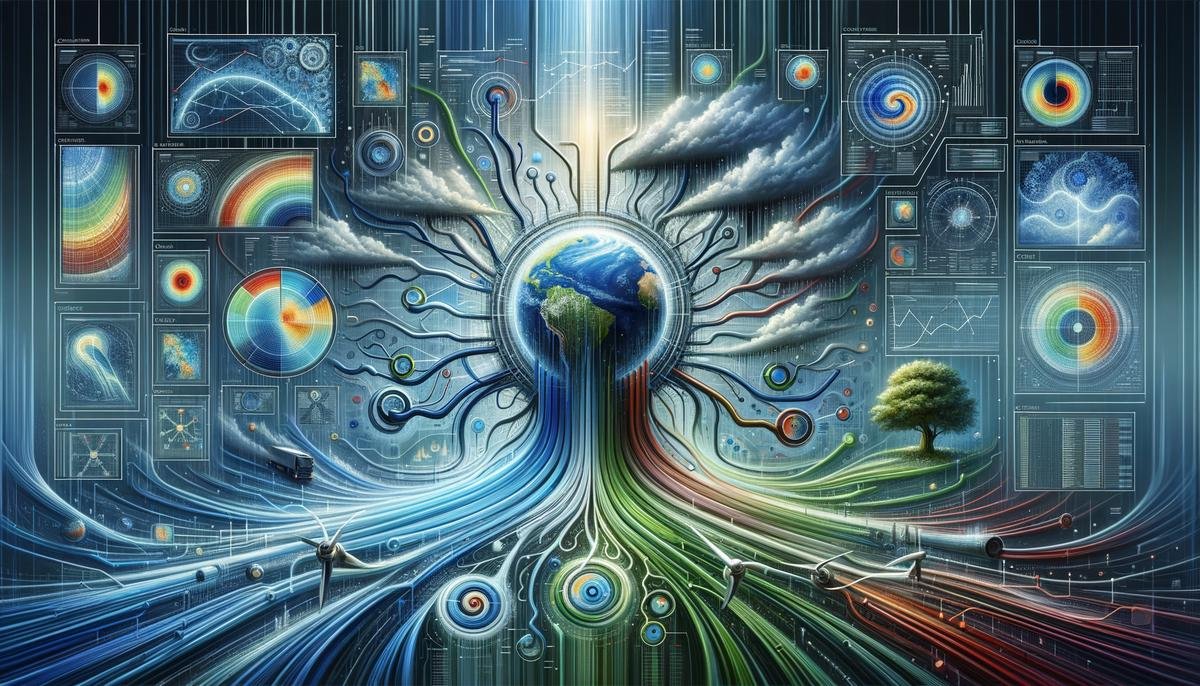
AI-Based Hybrid Models
AI-based hybrid models combine AI with traditional numerical forecasting methods to improve prediction accuracy. These models layer AI's pattern recognition capabilities over established frameworks of numerical forecasts.
Traditional models excel at capturing macro-level atmospheric and oceanic movements but can struggle with small-scale processes like cloud formation or localized precipitation patterns. AI helps by focusing on these intricate details.
Hybrid models can modify forecasts in real-time based on live data inputs, uncovering subtle meteorological signatures that might lead to extreme weather events.
These models are particularly good at improving forecast resolution, offering more localized predictions. This level of precision is crucial for timely alerts and urban planning.
Incorporating AI reduces the computational load typically required for exhaustive simulations, enabling quicker turnaround times and more responsive predictions.
Data and Methodological Challenges
Data scarcity remains a significant challenge in climate prediction. Despite numerous sensors and weather stations, there are still regions and aspects of our climate system with insufficient data, particularly in remote areas and over oceans.
The interpretability of AI models, especially complex neural networks, is another issue. Understanding how these models reach their predictions is crucial for building trust among scientists, policymakers, and stakeholders.
Generalizability is also a challenge, as AI models trained for one region may not perform well when applied to different geographical and atmospheric conditions.
To address these issues, standardized datasets and open-source tools are being developed. These resources aim to provide a consistent foundation for model training and foster collaboration within the research community.
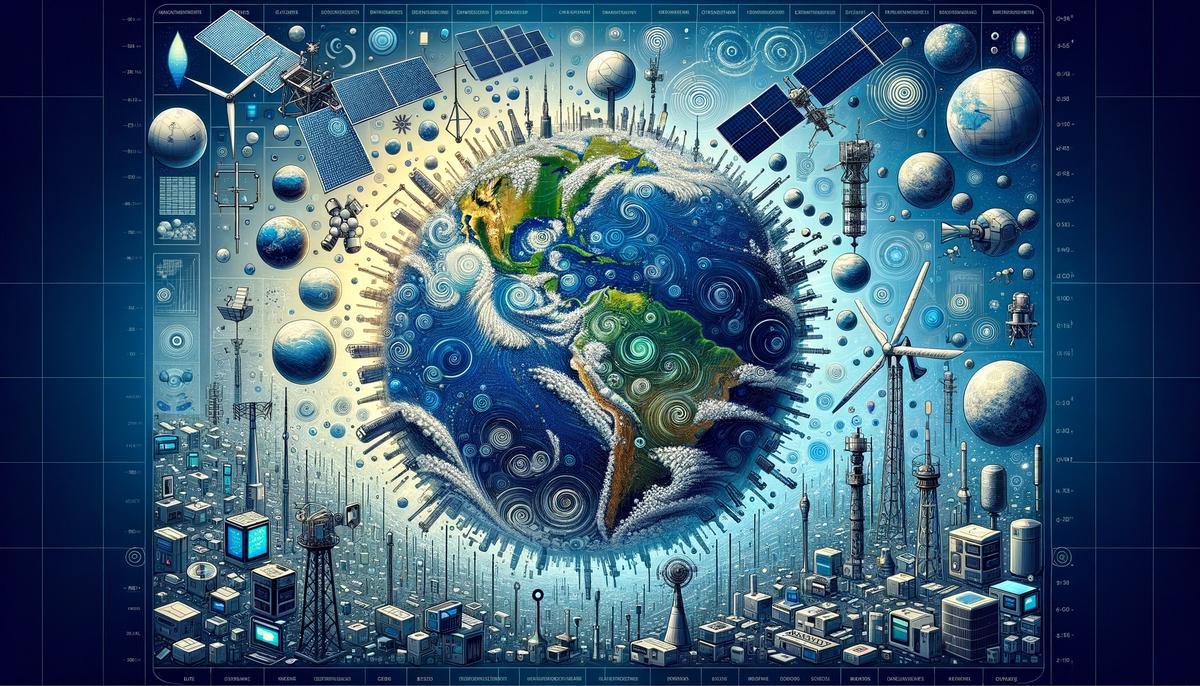
Future Perspectives and Innovations
The future of AI in climate science holds significant potential. Emerging innovations may lead to AI systems capable of offering comprehensive climate resilience solutions while running on eco-friendly architectures.
Advances in quantum computing could enhance AI's computational capabilities, allowing for more intricate climate models. Edge computing may enable faster, more localized climate predictions.
Collaboration across disciplines will be crucial in developing effective solutions. Integrating insights from meteorology, data science, policy-making, and social sciences could lead to more comprehensive and actionable predictions.
Developing environmentally sustainable AI technologies is important to minimize the environmental impact of these advancements. This includes using renewable energy sources and optimizing algorithms for efficiency.
The focus is on ensuring that AI-driven solutions contribute not only to climate predictions but also to global sustainability, involving ethical development, transparent information sharing, and collaborative efforts.
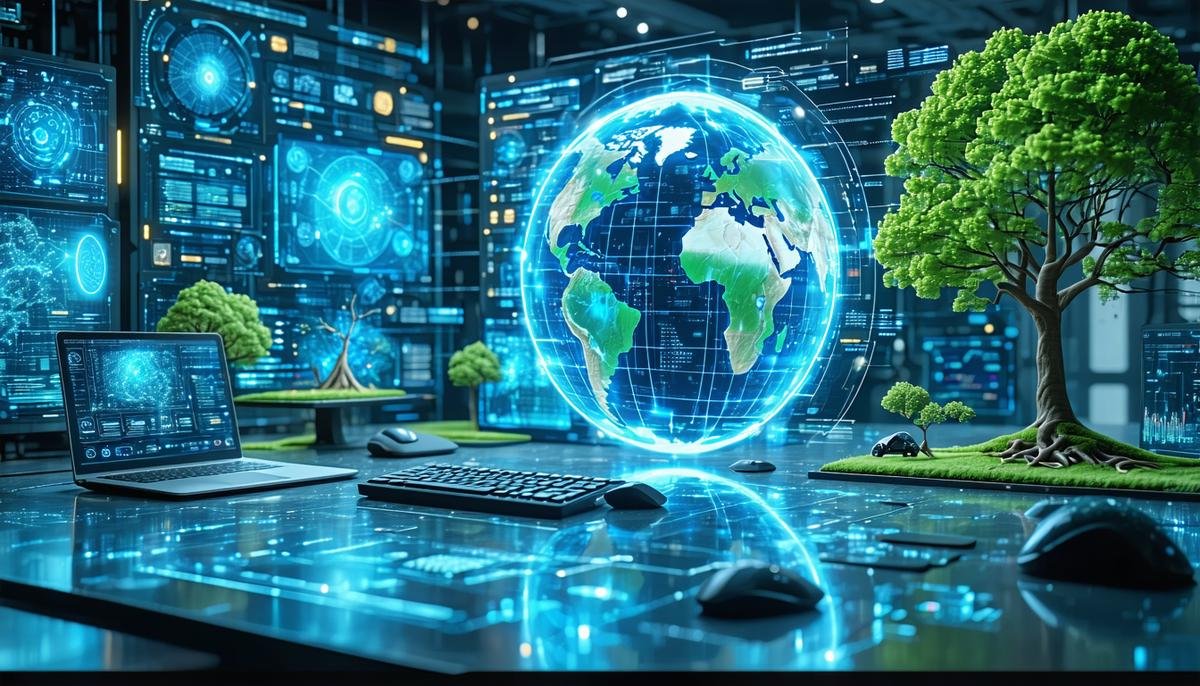
The integration of AI in climate science offers promising solutions for addressing climate challenges effectively and sustainably. This advancement aims to shape a resilient future for our planet through improved prediction and innovative approaches.
- Pachauri RK, Allen MR, Barros VR, et al. Climate Change 2014: Synthesis Report. Contribution of Working Groups I, II and III to the Fifth Assessment Report of the Intergovernmental Panel on Climate Change. IPCC, Geneva, Switzerland; 2014.
- Gale S. Climate change is happening now. Nature. 2022;608:S31-S31.
- Lerner K. Climate change, explained. Vox. 2023.
- Stern PC, Easterling WE. Making Climate Forecasts Matter. National Academy Press, Washington, D.C.; 1999.
- Hurrell J. The climate system: an overview. Climate Change 2001: The Scientific Basis. Cambridge University Press, Cambridge; 2003.
- Edwards PN. A Vast Machine: Computer Models, Climate Data, and the Politics of Global Warming. MIT Press, Cambridge, MA; 2010.
- Düben PD, Bauer P. Challenges and design choices for global weather and climate models based on machine learning. Geosci Model Dev. 2018;11:3999-4009.
- Kumar A, Hoerling MP. Prospects and limitations of seasonal atmospheric GCM predictions. Bull Am Meteorol Soc. 1995;76:335-345.
- Collins M, Chandler RE, Cox PM, et al. Quantifying future climate change. Nat Clim Chang. 2012;2:403-409.