Role of Data Quality in AI
High-quality data is essential for AI and machine learning systems. Accurate, complete, and relevant datasets are crucial for optimal performance. Without reliable data, AI models can produce inaccurate predictions and decisions, leading to missed opportunities and misguided strategies.
The integrity of AI systems depends on data quality. Biased or incomplete data can distort predictions and impact fairness. AI thrives on patterns and trends, making comprehensive datasets vital for tasks like anticipating customer needs or diagnosing healthcare conditions.
For businesses to remain competitive, they must prioritize data quality in their AI applications. Companies leveraging precise datasets can achieve more accurate forecasts and informed decision-making, harnessing AI's full potential.
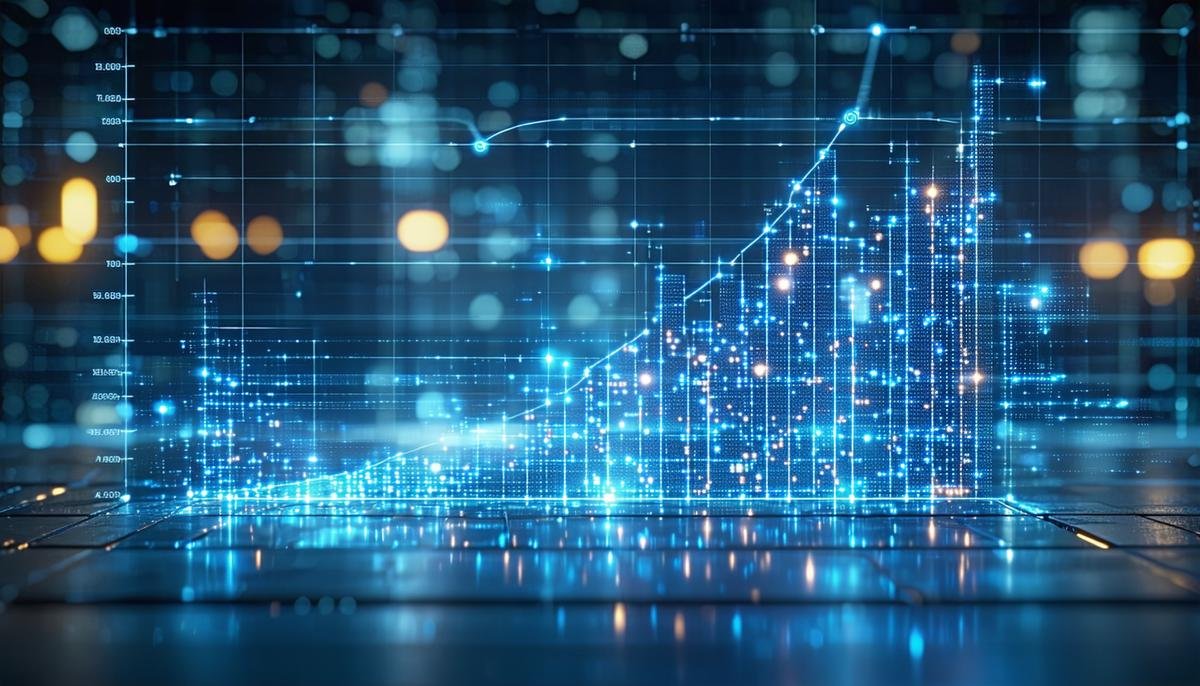
Challenges in Ensuring Data Quality
Securing data quality in AI and machine learning presents several challenges:
- Incomplete data: Missing information can skew the bigger picture, leading to unreliable conclusions.
- Inaccurate data: Human errors, faulty sensors, or hasty data entry can introduce inaccuracies that ripple through AI models.
- Outdated data: Relying on stale information can lead to unpredictable outcomes, especially in rapidly evolving fields.
- Biased data: Predictive models built on biased datasets can perpetuate prejudice and inequality.
These challenges underscore the importance of vigilant data curation and oversight. Organizations must continuously refine their datasets to ensure AI doesn't just function, but excels.
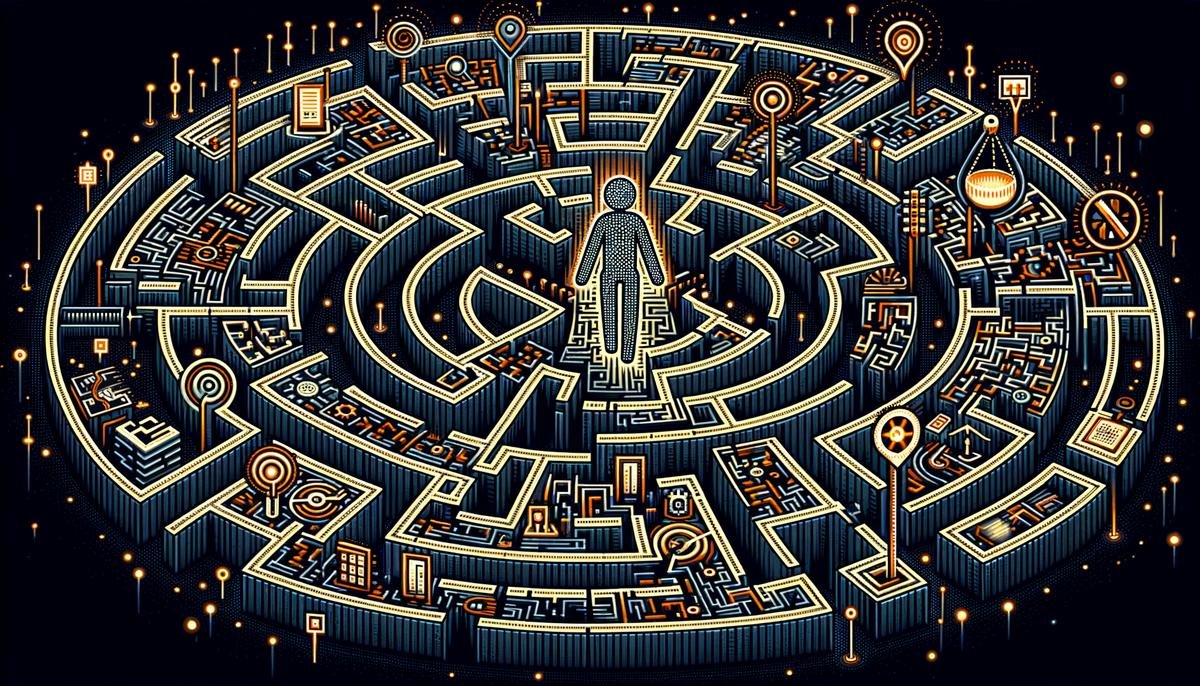
Technological Solutions for Data Quality
AI and machine learning offer solutions to improve data quality:
- Automated data cleaning: AI-powered tools efficiently identify and rectify corrupt or irrelevant data, saving time and reducing human errors.
- Anomaly detection: Machine learning algorithms recognize patterns and flag deviations, alerting operators to potential issues in real-time.
- Real-time data monitoring: Continuous oversight ensures data integrity remains uncompromised, especially in environments with constant data flows.
These technologies provide comprehensive frameworks for data quality management, aligning with evolving business needs. By automating repetitive tasks and offering continuous monitoring, they empower organizations to make reliable decisions based on high-quality data.
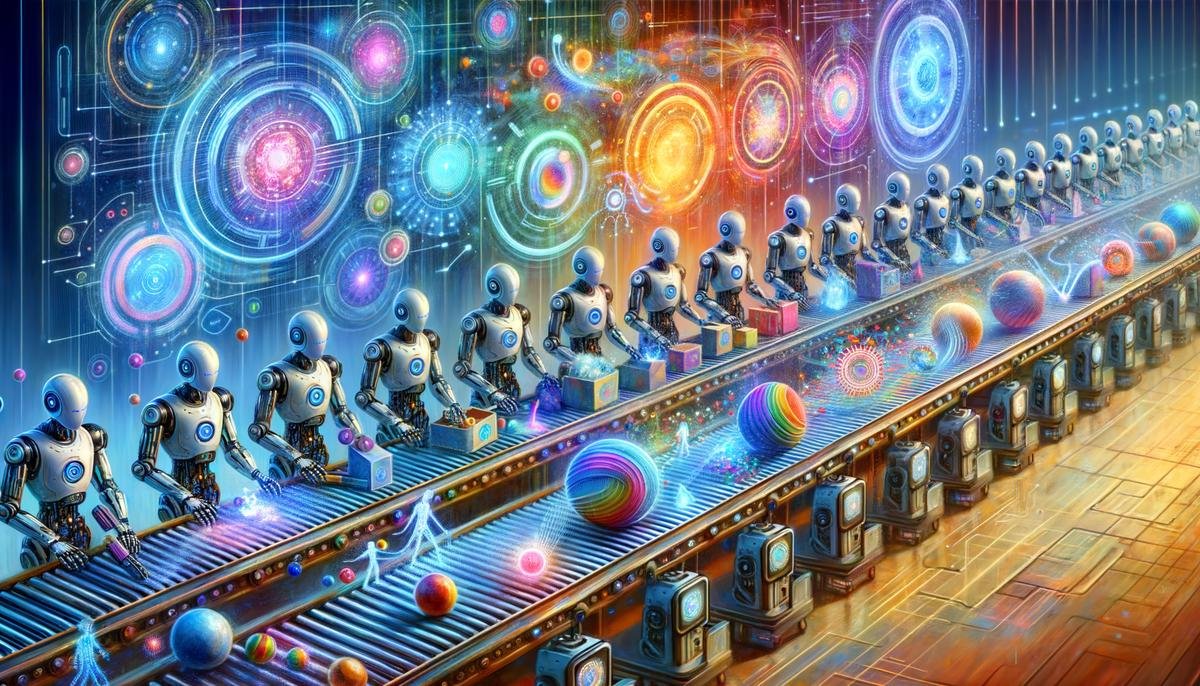
Data Management Strategies
Effective data management strategies are crucial for maintaining high-quality datasets:
- Data governance: Establishes clear policies on data access, security, and usage, promoting consistency and accountability across the organization.
- Validation rules: Act as gatekeepers, scrutinizing incoming data against predefined criteria to filter out anomalies before they enter the system.
- Regular audits: Systematic examinations of datasets and processes help identify discrepancies and potential issues, enabling timely interventions.
These strategies form a comprehensive approach to data management, ensuring that AI systems function optimally and provide actionable insights for successful business outcomes.
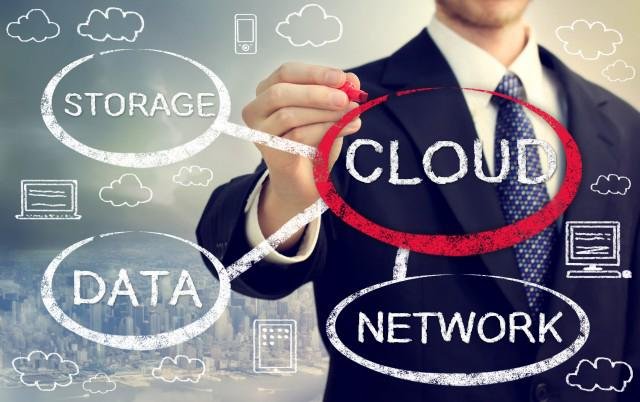
Impact of Poor Data Quality
Poor data quality can have significant consequences for AI systems:
- Inaccurate predictions: Compromised data can lead to misguided strategies and decisions.
- Bias amplification: AI systems can inherit and perpetuate biases present in training data, potentially exacerbating societal inequalities.
- Misdiagnoses in healthcare: Incomplete or biased data can result in incorrect medical decisions, putting patients at risk.
- Financial missteps: Outdated or irrelevant data in financial models can lead to poor investment decisions and economic ramifications.
These examples highlight the importance of maintaining high data quality to ensure responsible and effective AI implementation.
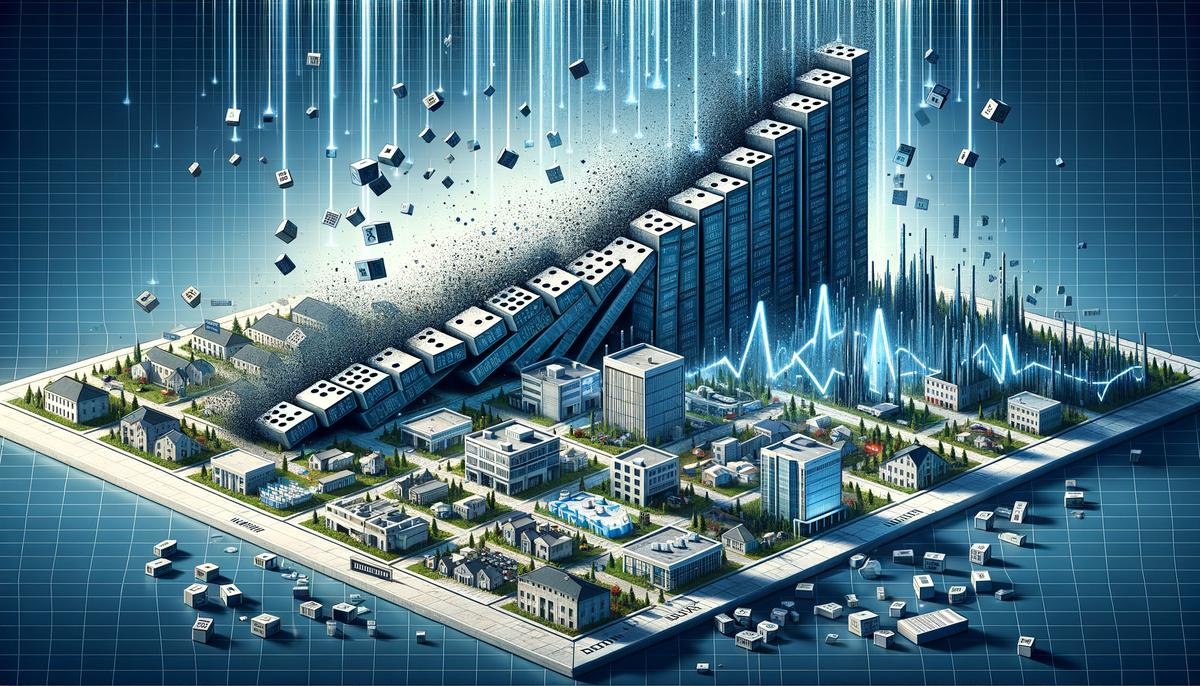
In AI, the quality of data is crucial. Without reliable data, AI systems can produce flawed outcomes, eroding trust. Organizations aiming to leverage AI effectively must prioritize high-quality data.
"The key to making generative AI great is by introducing proprietary enterprise data into generative AI pipelines. If that is not accurate or not reliable, then all of [an organization's] generative AI efforts will be moot."
This emphasizes the critical role of data quality in the success of AI initiatives. As AI and machine learning continue to evolve, the demand for accurate, comprehensive, and unbiased data will only increase, making data quality a cornerstone of modern business strategy.
- Moses B. The importance of data quality in generative AI. Data Quality Day. 2023.
- Sanderson C. The role of communication in data quality management. Data Quality Day. 2023.
- Neufeld D. Testing tools for improved data quality. Data Quality Day. 2023.