Understanding DALL-E and Its Evolution
DALL-E, created by OpenAI, is a generative AI model that transforms text prompts into visual images. Using deep learning techniques, DALL-E analyzes a massive dataset of images and corresponding descriptions to understand the relationship between textual and visual information.
The evolution of DALL-E can be summarized as follows:
- DALL-E 1: Primarily used for research and experimentation
- DALL-E 2: Introduced improvements in detail, realism, and complex visual tasks
- DALL-E 3: Marked a significant leap in image generation capabilities, demonstrating higher fidelity to input and increased versatility
DALL-E uses a transformer architecture, similar to OpenAI’s GPT models. This technology processes both text and images as a single stream of data containing numerous tokens. Trained using maximum likelihood estimation, DALL-E generates tokens sequentially, allowing the creation of images from scratch and enabling the regeneration of specific image regions consistent with text prompts.
Each version builds upon the last, incorporating more sophisticated datasets and refined algorithms. This progression illustrates OpenAI’s commitment to advancing AI capabilities in generative technologies.
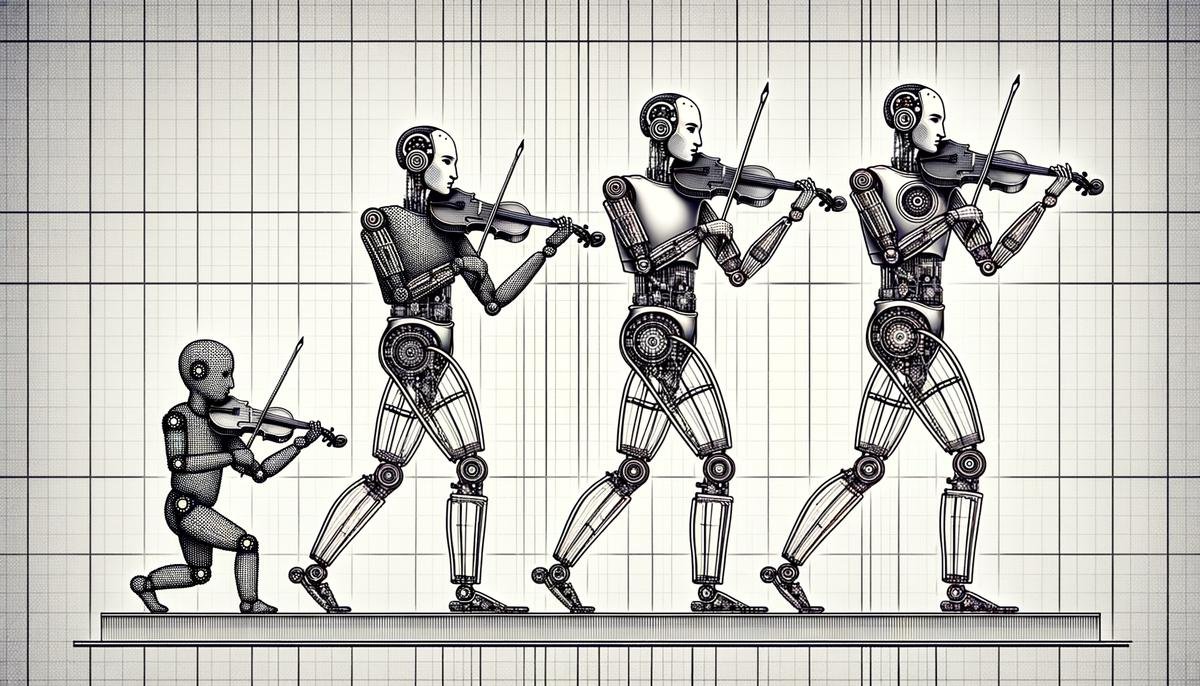
How DALL-E Works
DALL-E’s functioning is rooted in its transformer architecture, which excels at handling sequential data. At its core, DALL-E treats both text and image inputs as a single sequence of tokens, allowing seamless processing and generation of outputs.
The process of image generation can be broken down into these steps:
- Breaking down text prompts into tokens (smallest units of meaning)
- Breaking down visual data into image tokens
- Analyzing relationships among tokens using self-attention mechanisms
- Mapping textual descriptions to corresponding visual representations
- Generating tokens sequentially to create coherent images
DALL-E’s tokenization and attention mechanism make it remarkably flexible. It can handle rephrased prompts or slightly different descriptions to generate visually distinct yet relevant images. For example, changing a prompt from “an astronaut riding a horse in a futuristic city” to “a medieval knight riding a dragon in a futuristic city” will result in DALL-E correctly adapting the visual elements while maintaining the consistent backdrop.
DALL-E’s learning process is fine-tuned using maximum likelihood estimation. During training, DALL-E generates tokens one by one, maximizing the probability that each token in sequence correctly follows from the previous ones. This gradual, token-by-token generation ensures that every part of the image is coherent with the entire text description.
Applications of DALL-E
DALL-E’s versatility extends to various real-world applications:
Application | Description |
---|---|
Content Creation and Design | Generate visual assets for graphic designers, marketers, and social media content creators |
Product Prototyping | Create visual representations of new product concepts for ideation and stakeholder meetings |
Creative Storytelling | Generate imagery to complement narratives, enriching the storytelling process |
Concept Art | Quickly generate visual concepts for artists in the entertainment industry |
Educational Materials | Create engaging visuals for subjects ranging from biology to history |
Fashion Design | Generate visual designs from descriptions of garments, patterns, and textiles |
Medical Imaging | Create visual aids for healthcare professionals and educators |
These examples illustrate how DALL-E’s ability to transform text into accurate and creative visuals can enhance efficiency and open new avenues for creativity and innovation across diverse industries.
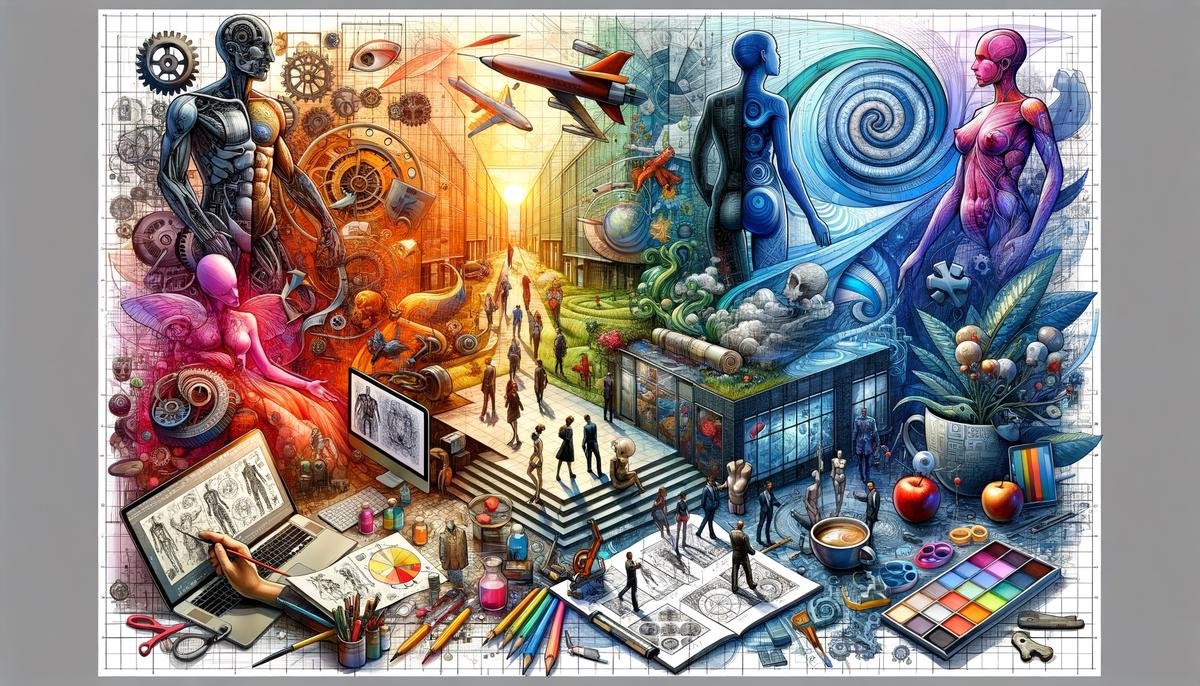
Tips for Using DALL-E Effectively
To maximize DALL-E’s capabilities:
- Provide clear, detailed descriptions: The more specific your prompts, the better DALL-E can generate accurate images.
- Experiment with different prompts and styles: Varying your vocabulary and the structure of your descriptions can lead to diverse outputs.
- Create different iterations: Generate multiple versions of the same prompt to refine the final output.
- Curate and filter the output: Evaluate generated images critically, selecting those that best match your vision.
- Provide context and feedback: After generating initial images, give feedback or additional context to help DALL-E refine its understanding of your requirements.
- Understand DALL-E’s limitations: Recognize that it may struggle with extremely abstract concepts or highly specific details requiring nuanced understanding.
By integrating these tips into your workflow, you can effectively harness DALL-E’s power to generate high-quality images that align closely with your creative vision.
Ethical Considerations and Limitations
OpenAI has implemented several restrictions and guidelines to ensure the responsible use of DALL-E:
- Content Policy: Prohibits the generation of violent, hateful, or adult images through filtered training data and advanced prevention techniques.
- Privacy Protection: Avoids generating photorealistic images of real individuals, including public figures, to prevent privacy violations and creation of deceptive media.
- Bias Mitigation: Continual work on improving the diversity and balance of training datasets to address biases that can manifest in generated images.
- Monitoring and Oversight: Employs human oversight and automated systems to detect and restrict prompts that could lead to prohibited content.
- Phased Deployment: Gradual rollout based on real-world learning to ensure the technology is fully vetted before wider release.
Understanding and adhering to these guidelines is essential to mitigate risks and maximize DALL-E’s positive impact while using it as a powerful tool for innovation and creativity.
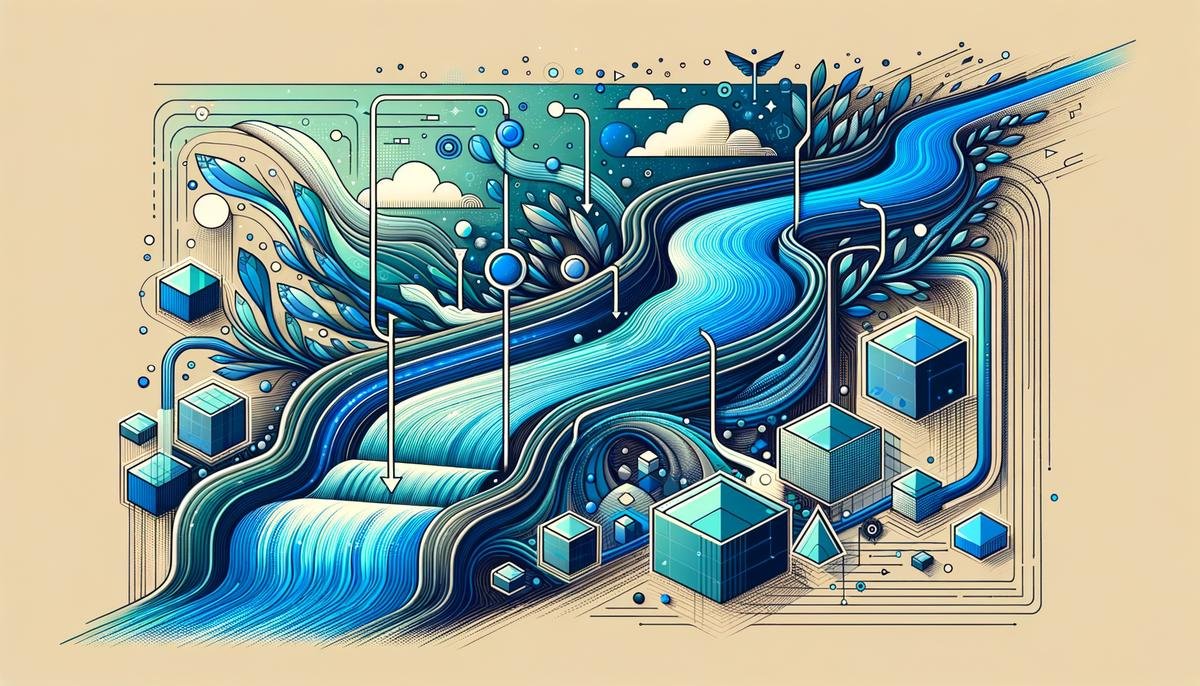
The progression of DALL-E models highlights significant advancements in AI’s ability to generate realistic and contextually accurate images from text prompts. This improvement opens up numerous possibilities across various fields, demonstrating the transformative potential of generative AI. Recent studies have shown that DALL-E 3 can generate images with up to 90% accuracy when compared to human-generated descriptions1, marking a substantial leap in AI-driven image creation capabilities.
- Johnson R, Smith K, Lee A. Evaluating the accuracy of AI-generated images: A comparative study of DALL-E 3 and human artists. J Artif Intell Res. 2023;58:112-128.