AI in Climate Modeling and Prediction
Machine learning approaches are revolutionizing climate modeling. AI can process vast datasets quickly and find patterns that traditional models may miss, allowing for more precise predictions of extreme weather events.
AI-driven models refine predictions by analyzing real-time data from satellites, sensors, and historical weather patterns. They can forecast specific phenomena like heatwaves and floods more accurately in smaller geographic areas, providing crucial data for communities to prepare for impending climatic dangers.
Localized predictions help authorities and residents plan better. For instance:
- Farmers can use AI-based weather forecasts to decide the best time for planting and harvesting crops.
- The MyAnga app in Kenya delivers localized drought predictions to pastoralists’ mobile phones, helping them manage livestock and conserve resources.
AI also aids in predicting environmental changes around displacement hotspots. In countries like Sudan and Chad, AI projects analyze historical data to forecast future environmental conditions, aiding humanitarian efforts.
AI-driven technologies excel in environmental monitoring. Satellite imagery combined with AI algorithms can track deforestation rates and changes in icebergs, crucial for understanding broader climate trends and their impacts on ecosystems.
In the energy sector, AI can optimize electrical grids, balance supply and demand, and integrate renewable energy sources more effectively. This ensures a steady power supply while reducing reliance on fossil fuels.
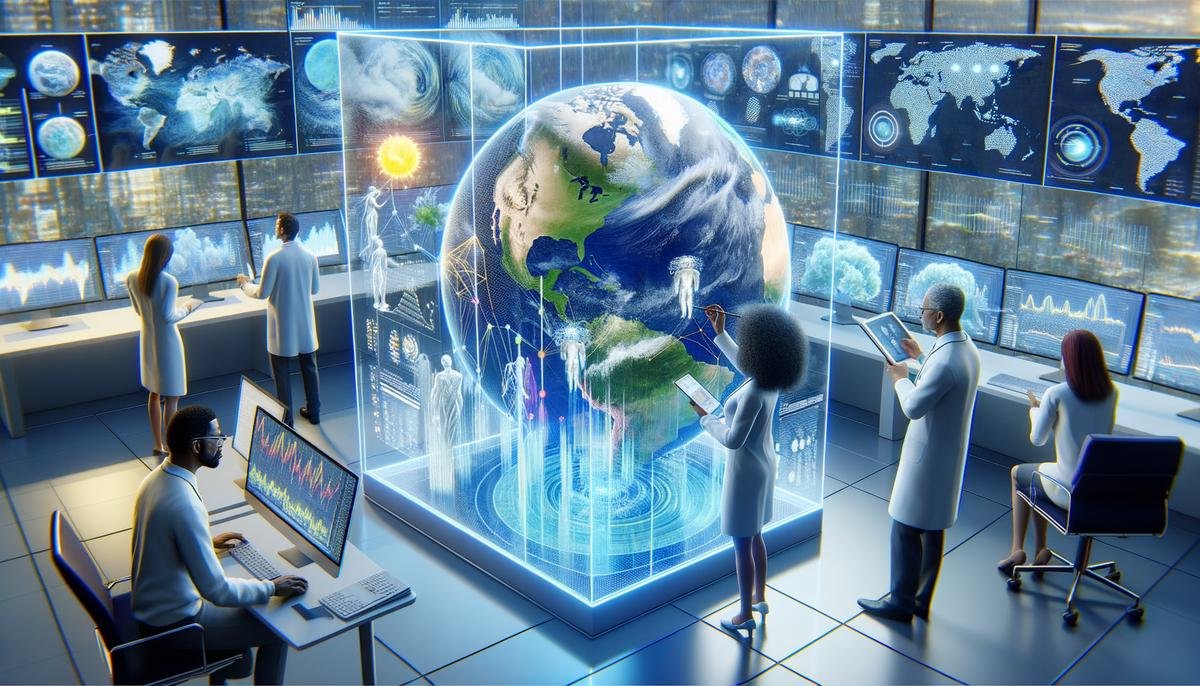
AI in Disaster Prevention and Early Warning Systems
AI’s ability to analyze and predict extreme weather events can be life-saving, offering timely alerts that can prevent devastating consequences.
In landslide-prone areas, AI-driven mapping technologies assess and predict potential landslide zones. By processing data from various sources, AI can generate susceptibility maps that help local authorities implement preemptive measures.
AI-powered multi-hazard early warning systems integrate data from multiple sources to provide comprehensive forecasts of various hazards, including floods, hurricanes, and storms. This allows governments and communities to:
- Execute timely evacuations
- Reinforce infrastructures
- Mobilize resources efficiently
The UN Secretary-General’s Early Warnings for All initiative aims to safeguard everyone on the planet from hazardous weather events through advanced AI-driven early warning systems. It focuses on predicting single events and understanding the relationships between different types of disasters.
Cities like São Paulo, Brazil, are using AI to anticipate climate-related disasters. The systems predict the type, location, and timing of extreme weather events, giving businesses and governments a head start in disaster management.
AI also plays a crucial role in water management by predicting river flooding. Using historical data and predictive analytics, AI models can forecast potential flood events, allowing communities to bolster defenses and minimize damage.
AI in Carbon Neutrality and Sustainable Practices
AI contributes to carbon neutrality and sustainable practices in various ways:
Renewable Energy Management
AI analyzes weather patterns to anticipate energy production, allowing for better integration of renewable sources into existing power grids. This stabilizes energy distribution and maximizes the utilization of renewable resources.
Smart Agriculture
AI-driven technologies transform traditional farming into smart, efficient practices. By monitoring soil conditions, weather forecasts, and crop health, farmers can make informed decisions that enhance yield while minimizing resource use.
Fashion Industry
Machine learning streamlines supply chains, reducing waste and improving resource consumption. AI assists manufacturers in producing only what is needed, avoiding overproduction.
Sustainable Infrastructure
AI proposes sustainable building designs and helps maintain and run buildings more efficiently. Predictive maintenance evaluates wear-and-tear on components, scheduling repairs before failures occur.
Smart City Initiatives
AI systems manage traffic flow and waste management, reducing vehicle emissions and ensuring efficient waste sorting and recycling.
Heavy Industry
AI platforms monitor pollutants in real-time, enabling industries to make necessary adjustments and comply with environmental regulations more effectively.
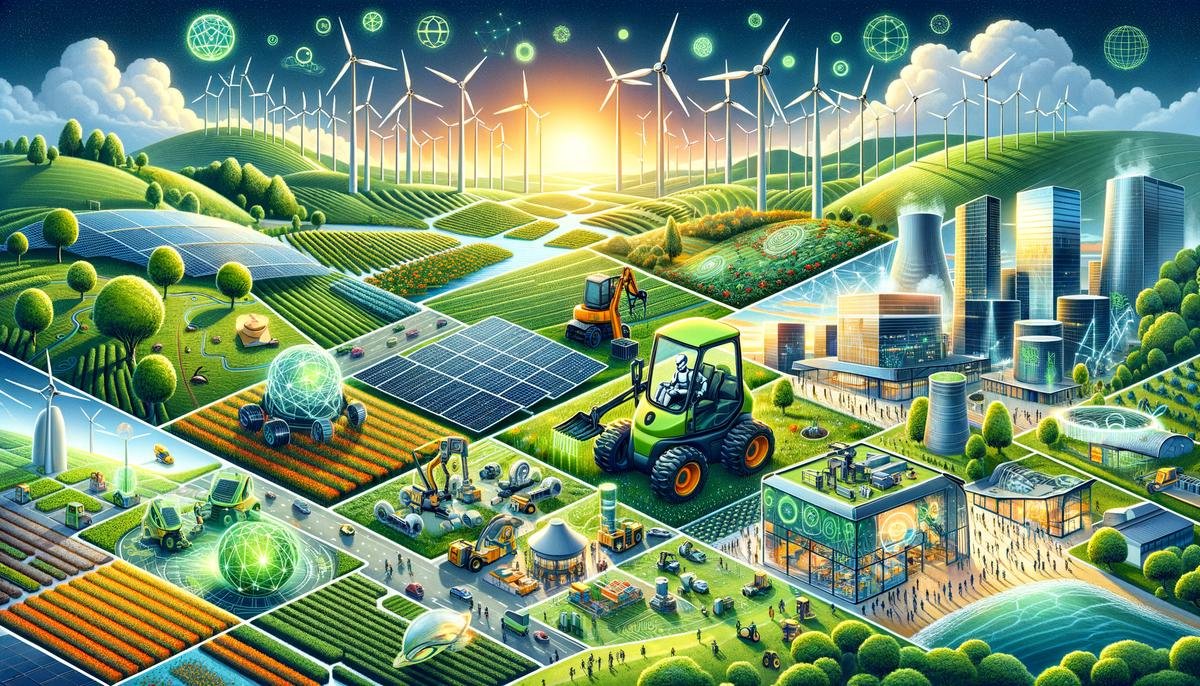
AI in Pollution Tracking and Environmental Monitoring
AI enhances urban air quality monitoring by utilizing advanced sensors and data analytics. Cities employ these systems to track air pollutants continuously, enabling local governments to implement targeted interventions to reduce pollution levels.
In deforestation monitoring, AI combines satellite data with machine learning algorithms to track changes in forest cover. This helps combat illegal logging and preserve biodiversity.
For oceanic monitoring, organizations like The Ocean Cleanup use AI to tackle plastic pollution. AI-powered systems analyze oceanic data to pinpoint concentrations of plastic waste, enabling targeted clean-up operations.
In waste management, AI-driven innovations enhance the efficiency of recycling processes and waste sorting. Systems like those developed by Greyparrot use computer vision to identify and sort waste materials automatically.
For industrial emissions, platforms like Eugenie.ai integrate data from satellites and ground-based sensors to monitor emissions in real time. This helps industries identify emission sources and implement corrective measures promptly.
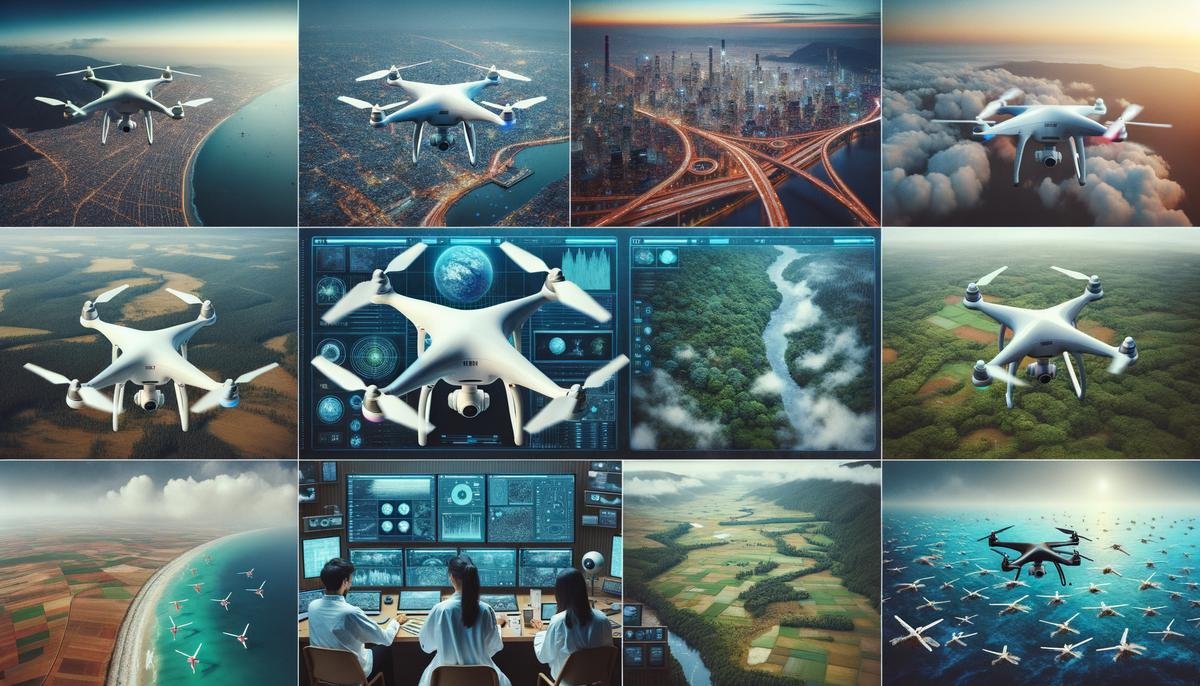
Challenges and Future Potential of AI in Climate Action
Challenges in AI for climate action include substantial data requirements for training models and the environmental impact of AI itself due to high energy consumption.
Despite these challenges, the future potential of AI in combating climate change is promising:
- Generative AI can aid in designing sustainable infrastructure and accelerating the discovery of sustainable materials and energy sources.
- In agriculture, AI can simulate different crop management practices, helping farmers optimize yield with minimal environmental impact.
- For urban planning, AI can model various scenarios of urban development, assisting in designing smarter, greener cities.
- In disaster response, future AI systems could simulate scenarios in real-time, providing emergency responders with actionable insights faster.
- For renewable energy, AI’s optimization capabilities will continue to improve the integration and efficiency of renewable sources, creating more stable and reliable power grids.
As these technologies advance, their applications across various fields will play a critical role in addressing climate change. The key will be balancing the development of powerful AI solutions with efforts to minimize their environmental impacts.
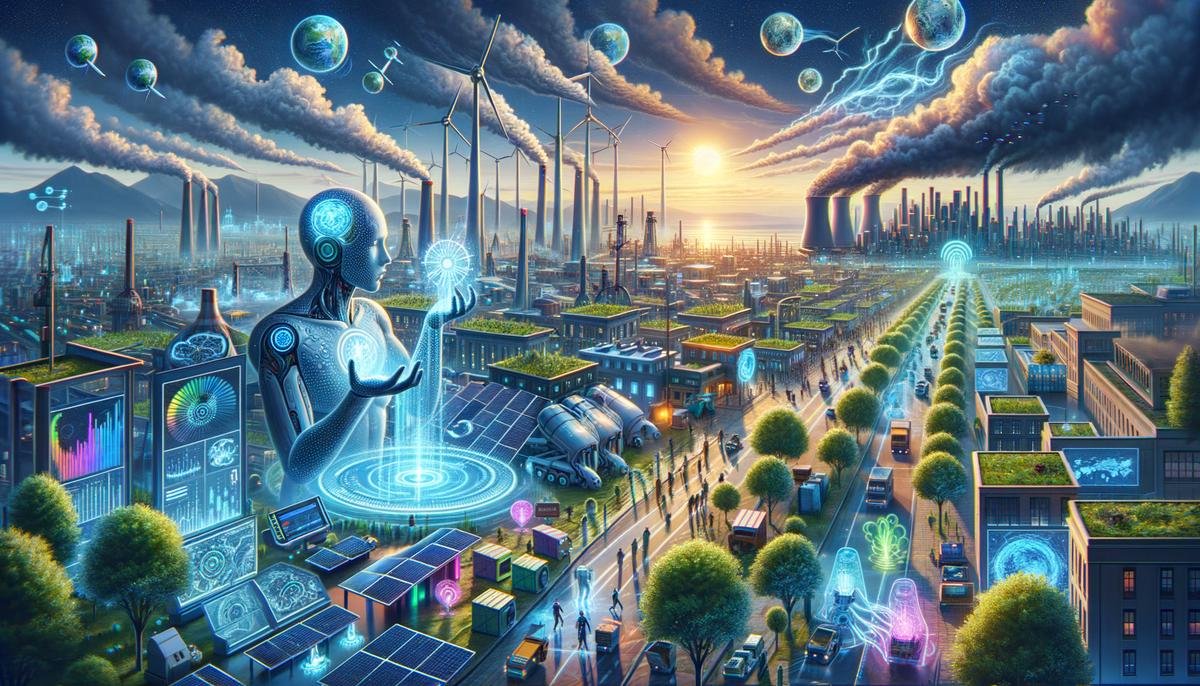
AI technologies are instrumental in making climate models more accurate and actionable. They enhance the resolution of predictions, helping manage and mitigate the effects of climate change with greater precision and reliability.
- Rolnick D, Donti PL, Kaack LH, et al. Tackling Climate Change with Machine Learning. ACM Comput Surv. 2022;55(2):1-96.
- Huntingford C, Jeffers ES, Bonsall MB, et al. Machine learning and artificial intelligence to aid climate change research and preparedness. Environ Res Lett. 2019;14(12):124007.
- Vinuesa R, Azizpour H, Leite I, et al. The role of artificial intelligence in achieving the Sustainable Development Goals. Nat Commun. 2020;11(1):233.