The Role of AI in Drug Discovery
AI is transforming drug discovery and development. Traditional drug discovery is lengthy and costly, typically taking over a decade and billions of dollars. AI aims to streamline this process using advanced algorithms to analyze biological data, predict potential drug candidates, and optimize development.
Traditional Challenges
Drug discovery involves target identification, validation, and compound screening. These processes are slow and expensive. Identifying a target alone can take years, followed by screening millions of compounds and clinical trials. High attrition rates and unpredictable results add to the challenges.
AI’s Solutions
AI, particularly machine learning, addresses these challenges:
- Target Identification and Validation: AI algorithms analyze large datasets to quickly identify and validate potential drug targets.
- High-Throughput Screening: AI speeds up data analysis, identifying promising compounds faster and shortening the hit-to-lead optimization phase.
- Predictive Modeling for Drug Design: AI-driven modeling virtually screens compounds, estimating efficacy and potential side effects, reducing physical testing needs.
- Data Integration: AI integrates diverse datasets, enhancing understanding of complex biological systems and aiding target identification.
AI-Driven Models in Action
- Generative AI for Molecular Design: Models like GANs and VAEs create new molecules with desired attributes, learning from existing data.
- Predictive Analytics: AI forecasts pharmacokinetic and pharmacodynamic properties early in discovery, helping identify promising candidates.
- Target Identification: AI models analyze biological data to pinpoint potential drug targets and suggest new ones.
Real-World Applications
AlphaFold 3: DeepMind’s AlphaFold 3 predicts protein structures accurately, speeding up the identification of viable drug candidates.
Atomwise’s Contributions: Atomwise uses convolutional neural networks to predict molecule-protein interactions. Their AtomNet platform can screen billions of compounds rapidly.
Beyond Discovery
AI accelerates new drug discovery and aids in repurposing existing drugs. It also contributes to personalized treatments by analyzing patient-specific data.
Key Takeaways
- AI algorithms analyze biological data, predict drug candidates, and optimize development processes.
- AI models like AlphaFold 3 and AtomNet are making significant strides.
- AI aids in repurposing existing drugs and personalizing treatments.
- As technology advances, AI’s impact will continue to grow.
Key Technologies in AI Drug Discovery
AlphaFold 3 and generative AI models like GANs and VAEs are key technologies in AI drug discovery.
AlphaFold 3, developed by DeepMind, predicts protein structures with high accuracy. This is crucial for understanding how drugs interact with targets. AlphaFold 3’s deep-learning algorithms analyze amino acid sequences to predict protein configurations. By making these predictions and the underlying code accessible, DeepMind fosters global collaboration and accelerates scientific discovery.1
Generative AI models create new, potential drug-like molecules. GANs use a generator and discriminator network to create and evaluate new data instances. VAEs compress and decompress input data to understand essential characteristics. These models can forecast pharmacokinetic and pharmacodynamic properties early in discovery, helping identify promising candidates.
“AI-powered drug discovery has the potential to revolutionize the pharmaceutical industry, dramatically reducing the time and cost of bringing new treatments to patients.”
These technologies have real-world applications. Atomwise’s AtomNet platform screens billions of compounds quickly, expediting the process from discovery to clinical trials. AI also aids in drug repurposing and enables precision medicine by customizing treatments based on individual patient data.
As these technologies evolve and integrate into biomedical research, they promise to develop effective and personalized treatments at unprecedented speeds.
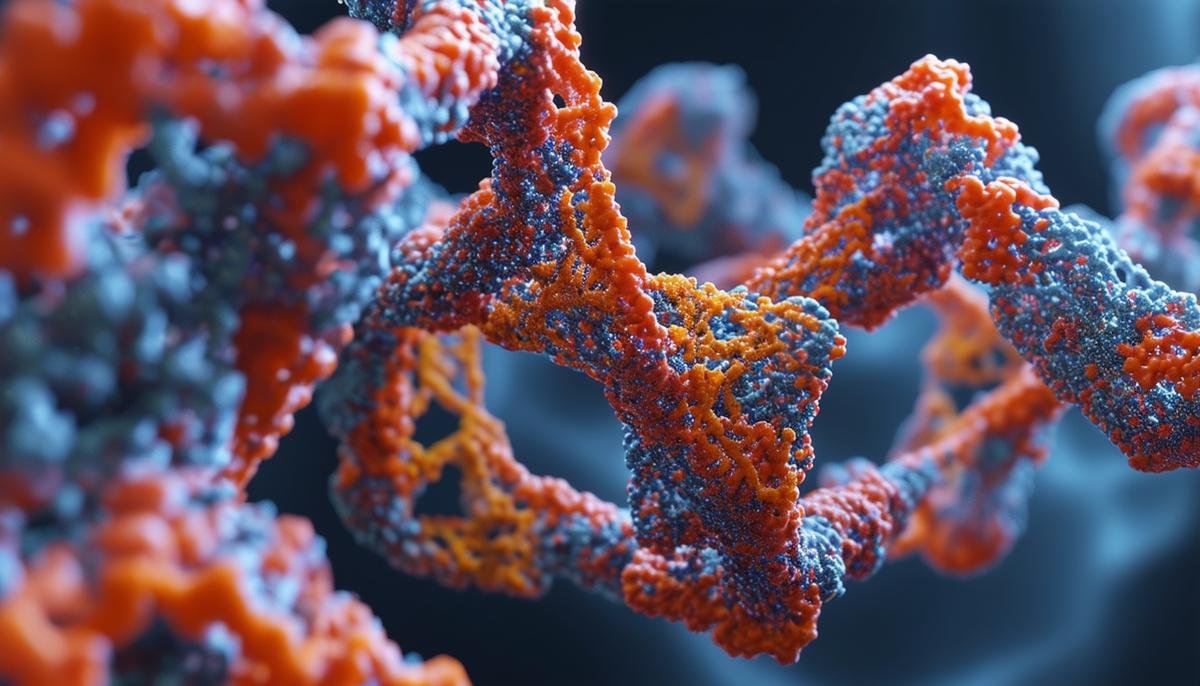
Atomwise’s AI Algorithms and Their Impact
Atomwise’s AtomNet™ platform uses convolutional neural networks (CNNs) to predict drug-protein interactions quickly and accurately. It can screen over 16 billion compounds in less than two days, significantly accelerating hit identification.2
AtomNet analyzes the spatial configurations of molecules and proteins to predict binding affinity and selectivity. This approach has shown success in various projects, including identifying a potential Ebola treatment.
Atomwise collaborates with pharmaceutical companies and academic institutions, integrating AI into diverse therapeutic research projects. Their Artificial Intelligence Molecular Screen (AIMS) initiative provides researchers with virtual screens and access to predicted binding molecules, fostering innovation across the scientific community.
Feature | Description |
---|---|
Screening Capacity | Over 16 billion compounds in <2 days |
Technology | Convolutional Neural Networks (CNNs) |
Key Application | Drug-protein interaction prediction |
The company’s use of NVIDIA’s CUDA parallel computing platform and GPUs enables large-scale screenings. In a recent project, Atomwise scaled from 10 million to 10 billion molecules, achieving screening speeds of around 2,000 molecules per second.
Atomwise’s integration of AI into drug discovery demonstrates technology’s potential to reshape the industry. By accelerating discovery and opening new avenues for collaboration, Atomwise is contributing to a future where drug discovery is faster, more efficient, and increasingly precise.
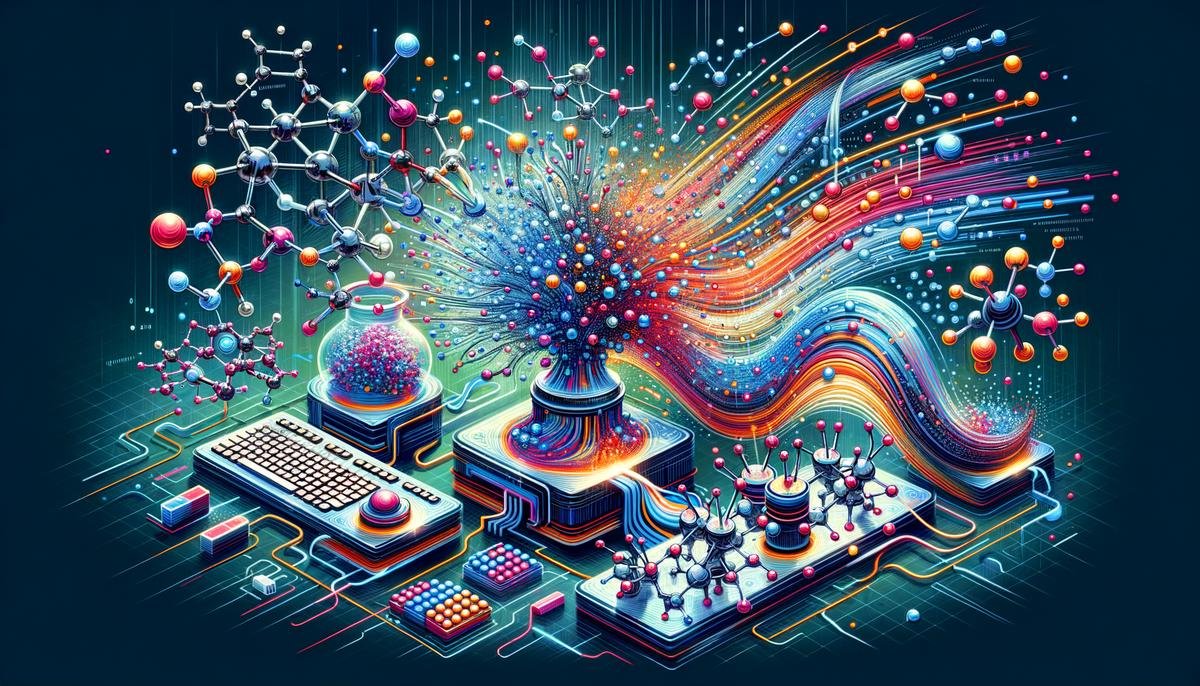
Applications and Success Stories
AI application in drug discovery has seen notable achievements, particularly with companies like Atomwise. Using their AtomNet platform, Atomwise identified potential treatments for Ebola, screening millions of molecules and pinpointing a candidate that effectively blocked the virus from infecting cells across multiple strains. This process, which typically takes years, was completed in weeks.
Atomwise’s impact extends to other diseases. In a project targeting multiple sclerosis (MS), AtomNet explored 8.2 million molecules to identify compounds that showed promise in pre-clinical trials and animal models. This achievement underscores AI’s efficiency in drug discovery and its capacity to explore complex diseases.
Collaborative Successes:
- Partnerships with pharmaceutical giants like Sanofi
- Collaboration with the University of Toronto, identifying new drug leads for neurodegenerative diseases
- Oncology breakthroughs, finding compounds for rare cancers
AI’s analytical capabilities are also leveraged in drug repurposing. During the COVID-19 pandemic, Atomwise collaborated with academic institutions to identify broad-spectrum antiviral therapies. BenevolentAI identified baricitinib, originally used for rheumatoid arthritis, as a potential COVID-19 treatment by analyzing extensive biomedical and clinical data.1
“These AI-driven discoveries shorten drug development timelines, reduce costs, and increase success rates.”
Other companies like Exscientia have also advanced AI-designed drug candidates to clinical trials, validating AI’s role in developing effective small-molecule therapeutics quickly.
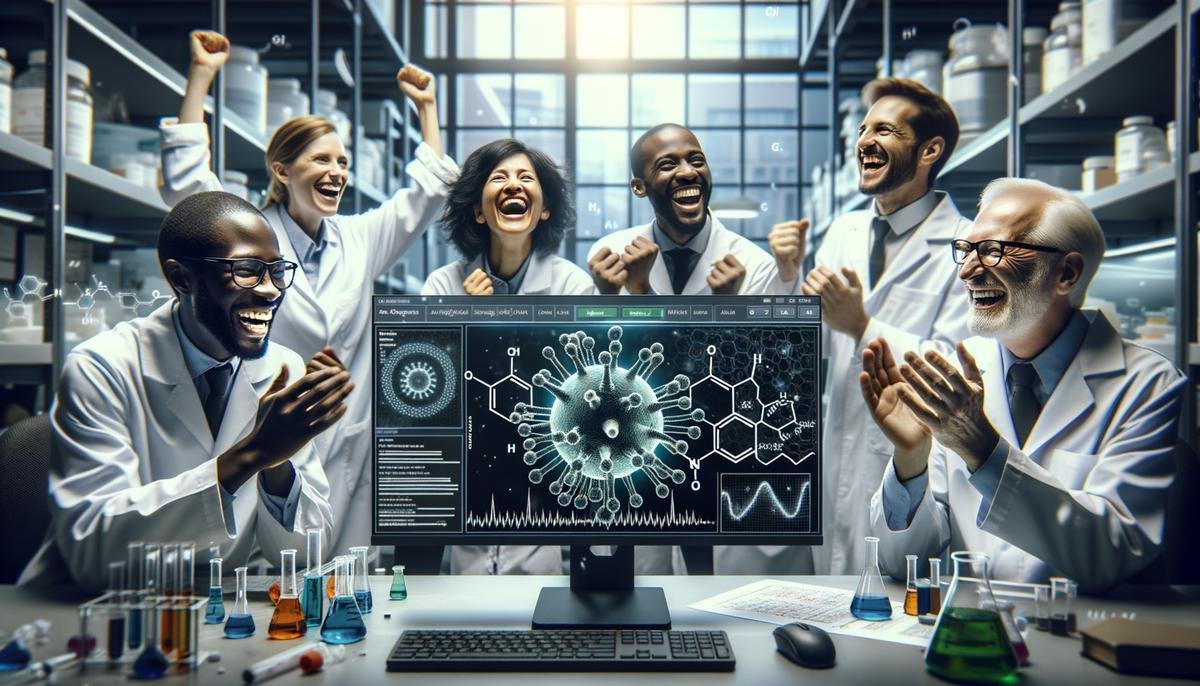
Challenges and Future Directions
While AI in drug discovery offers benefits, it also presents challenges:
- Data privacy: Ensuring protection of sensitive patient information
- Regulatory compliance: Establishing guidelines for AI-driven solutions
- Algorithmic biases: Requiring careful curation of training datasets
Future advancements in AI technologies hold promise for further revolutionizing drug discovery. Improving the interpretability of AI models is one area of development. Integrating real-world evidence (RWE) into AI models could lead to more effective and personalized treatments. Advancements in computational power and algorithm efficiency will also play a critical role in processing larger and more complex datasets.
Collaborative efforts between pharmaceutical companies, AI technology firms, regulatory bodies, and academic institutions are essential for sharing knowledge, resources, and expertise. These collaborations can help address challenges and drive advancements in the field.
As AI technologies continue to evolve, their impact on drug discovery promises to grow, potentially leading to:
- Faster drug development cycles
- More efficient resource allocation
- Highly personalized medicine
The future of AI in drug discovery is bright, with the potential to transform healthcare and improve patient outcomes worldwide.2
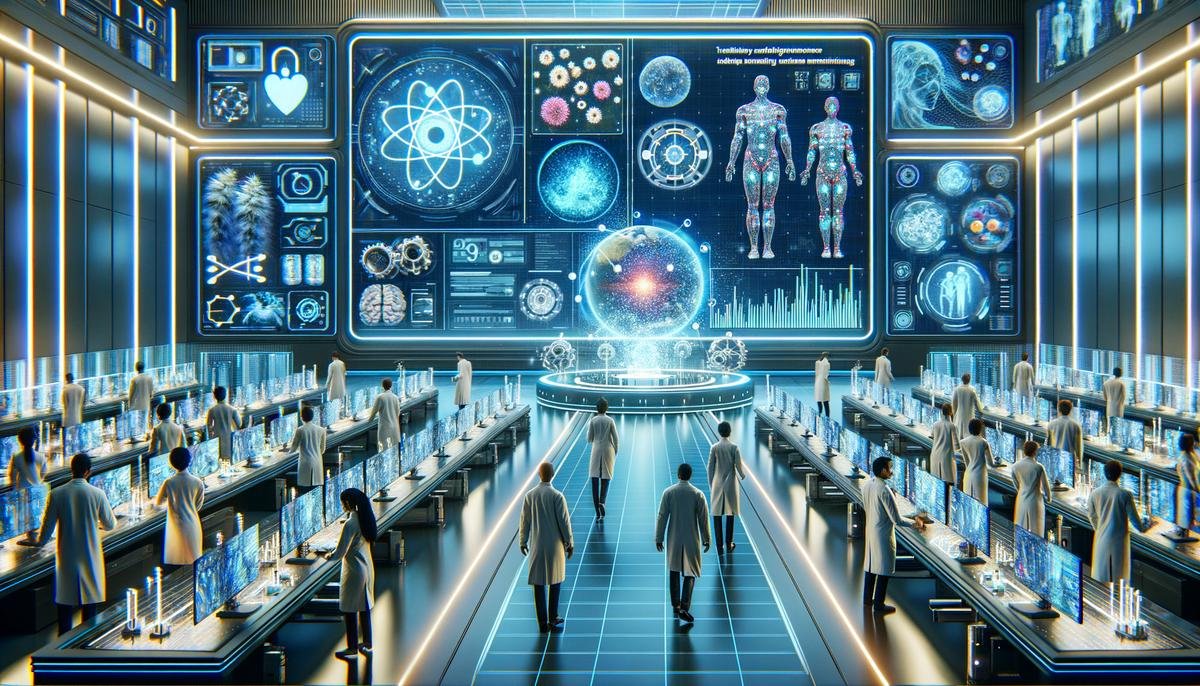