AI Predictive Analytics leverages advanced mathematical and statistical methods to forecast outcomes, making it a powerful tool for businesses. By understanding its key components—data, algorithms, and predictions—organizations can harness its potential to make informed decisions quickly and accurately.
Understanding AI Predictive Analytics
AI Predictive Analytics uses mathematical and statistical methods, including artificial intelligence and machine learning, to predict outcomes. It relies on three key components:
- Data: The foundation, with business data, often historical, being vital for training AI models.
- Algorithms: Sequences of steps for data analysis, including modern AI algorithms like machine learning and deep learning networks.
- Predictions: The ultimate goal, where AI models use algorithms to make data-driven predictions about future trends, customer behavior, or potential risks.
AI accelerates the predictive analytics process, analyzing millions of data points swiftly. This speed and scope enable businesses to make quick, informed decisions, enhancing efficiency and accuracy.
AI enhances traditional predictive analytics by rapidly processing and organizing vast data amounts. This results in accurate and actionable insights, benefiting sectors like healthcare, finance, and marketing. AI models can identify patterns, learn from them, and make data-driven predictions, improving the decision-making process.
The interplay between AI, ML, and predictive analytics brings numerous benefits. Businesses can streamline operations, enhance customer satisfaction, and gain competitive advantages, using data efficiently to make smarter decisions.
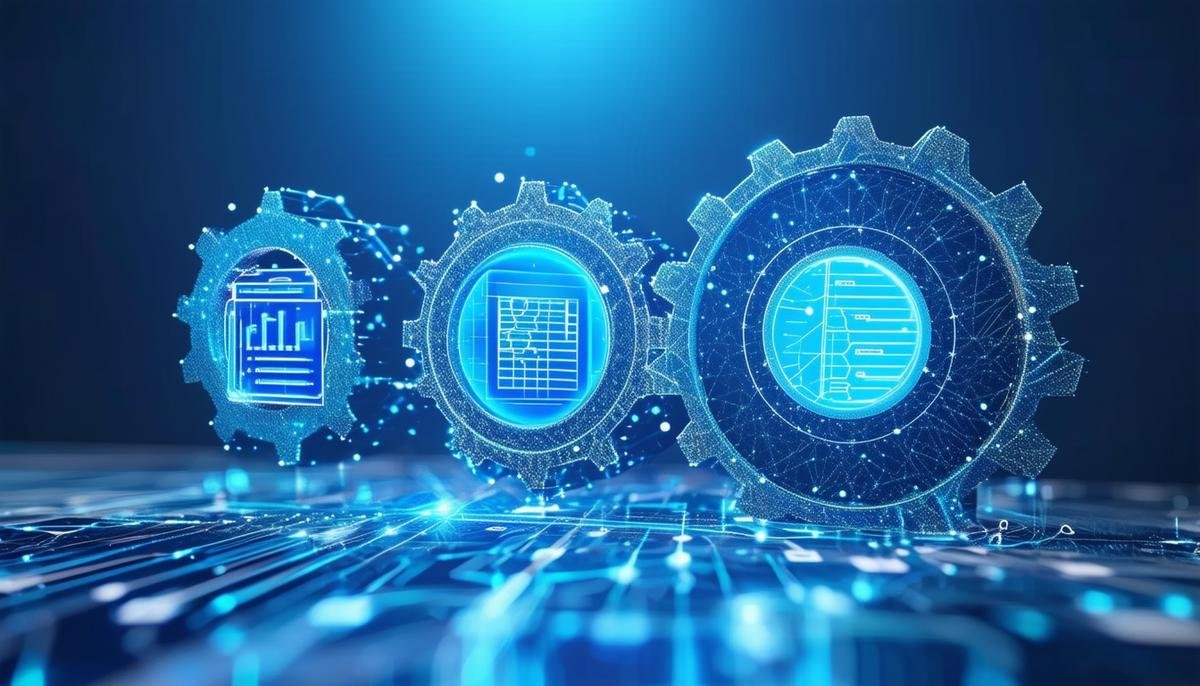
Applications of AI Predictive Analytics
AI predictive analytics finds diverse applications across various sectors:
Sector | Applications |
---|---|
Healthcare | Predicting patient admissions, identifying high-risk patients, forecasting disease outbreaks, optimizing treatment plans |
Finance | Predicting stock prices, assessing credit risk, detecting suspicious transactions |
Marketing | Analyzing customer data to predict purchasing patterns, enabling targeted advertising campaigns |
Sales | Forecasting sales trends, demand planning, identifying and prioritizing potential leads |
Customer service | Analyzing customer interactions to predict issues, providing instant support through AI-powered chatbots |
Supply chain | Optimizing logistics by predicting potential disruptions |
The adaptability of AI predictive analytics offers customized solutions across industries, leading to smarter decision-making, efficient operations, and enhanced customer satisfaction.
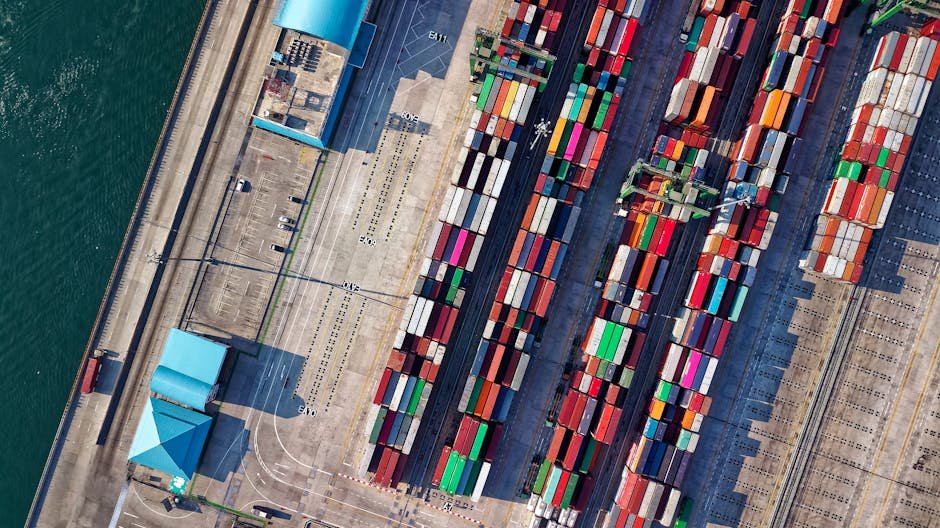
Benefits and Challenges of AI Predictive Analytics
Benefits of AI predictive analytics include:
- Improved decision-making
- Increased efficiency
- Competitive advantage
- Innovation
Challenges of AI predictive analytics include:
- Data privacy concerns
- Data quality issues
- Potential bias
- Integration complexity
- Transparency issues
Addressing these challenges effectively will allow organizations to fully realize the potential of AI predictive analytics, driving innovation and achieving sustained business success.
Future Trends in AI Predictive Analytics
Emerging technologies in AI predictive analytics include:
- Federated learning: Enables AI models to train on decentralized data sources without compromising privacy.
- Edge computing: Brings AI processing closer to the source of data generation, reducing latency and enabling real-time analytics.
Innovative applications are expanding to new domains:
- Education: Customizing learning experiences based on individual student needs and patterns.
- Agriculture: Analyzing weather patterns, soil conditions, and crop health to optimize farming practices.
To prepare for these advancements, businesses should:
- Invest in technology infrastructure
- Focus on data quality
- Enhance data privacy measures
- Cultivate a skilled workforce
- Develop explainable AI frameworks
- Adopt a flexible and adaptive strategy
By embracing these practices, businesses can leverage AI predictive analytics to stay competitive, drive innovation, and achieve sustained success.
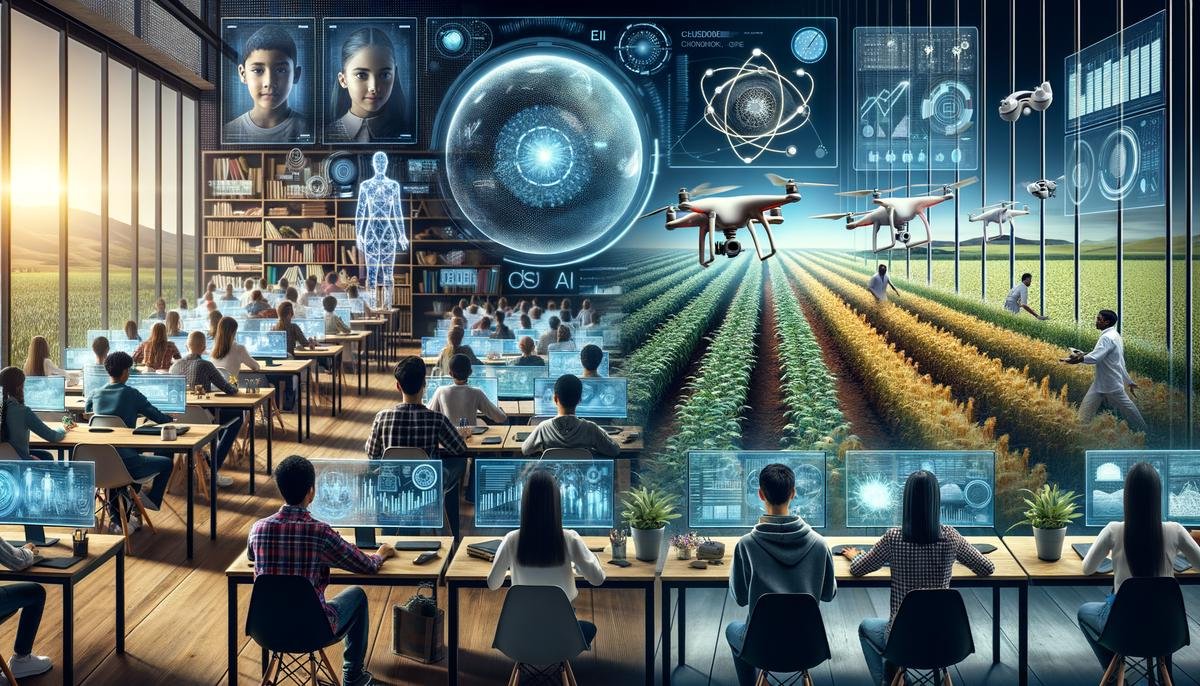
Integrating AI into predictive analytics enhances decision-making processes, offering precise and actionable insights. As technology advances, AI predictive analytics will continue to drive innovation and efficiency across various sectors. The future of AI predictive analytics holds immense potential, with an estimated market size of $28.6 billion by 20261.
- MarketsandMarkets. Predictive Analytics Market by Component, Solution, Service, Deployment Mode, Organization Size, Industry Vertical, and Region – Global Forecast to 2026. 2021.